- お役立ち記事
- Fundamentals of controller design using data directly (FRIT) and application to data-driven prediction
Fundamentals of controller design using data directly (FRIT) and application to data-driven prediction
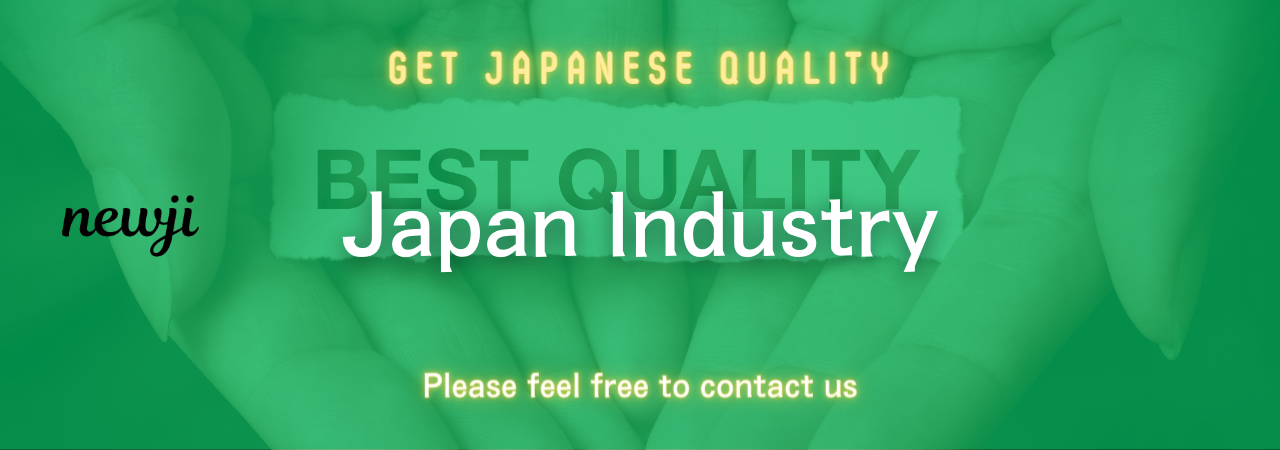
目次
Introduction to Data-Driven Controller Design
The world of control engineering is evolving rapidly with advancements in data-driven approaches.
Traditional methods heavily relied on pre-defined mathematical models and assumptions about the system being controlled.
However, with the emergence of big data and advanced computational techniques, there is a shift towards utilizing real-time data for control system design.
Understanding FRIT: Fundamentals of Controller Design Using Data Directly
FRIT, or the Fundamentals of Controller Design Using Data Directly, is a groundbreaking approach in the realm of control systems.
Instead of leaning on theoretical models, FRIT aims to design controllers based on actual data collected from the system.
This methodology leverages data to understand the dynamics and behaviors of the system, which, in turn, helps derive more effective and resilient control strategies.
The Advantages of Direct Data Utilization
The primary advantage of using data directly in controller design is its adaptability.
Systems often undergo changes over time due to wear and tear, environmental variations, or unforeseen factors.
FRIT allows engineers to design controllers that can adapt to these changes more fluidly than traditional methods.
Another benefit is the reduction of assumptions.
When creating models, engineers often need to make assumptions about the system, which may not always hold true.
Direct data allows for a design that’s inherently tailored to the system’s real state.
Challenges in Data-Driven Approaches
Despite its advantages, designing controllers using data directly is not without challenges.
One significant hurdle is the quality of data itself.
Noisy or incomplete data can lead to erroneous controller designs.
Thus, ensuring data accuracy and integrity is paramount.
Additionally, processing large volumes of data in real-time requires sophisticated algorithms and computational resources.
Engineers must balance between data accuracy, processing speed, and computational constraints to achieve optimal results.
Data-Driven Prediction Techniques
As part of FRIT, data-driven prediction techniques play a vital role.
By utilizing historical and real-time data, these techniques aim to forecast the behavior of the system and anticipate potential issues before they arise.
This predictive capability enhances the controller’s ability to manage the system effectively.
Machine Learning in Predictions
Machine learning has become a pivotal tool in data-driven prediction.
Algorithms such as neural networks, support vector machines, and decision trees analyze and learn from data patterns, enabling them to predict future system states.
These predictions are invaluable in creating controllers that are not only reactive but also proactive.
The Role of Real-Time Data Analysis
Real-time data analysis is fundamental to making accurate predictions.
Systems must have the capability to ingest and process data as it is generated, enabling immediate insights and corrective actions.
Techniques like online learning and adaptive filtering are crucial in maintaining the relevance of predictions over time.
Applications of FRIT in Various Industries
The principles of FRIT and data-driven predictions are being applied across numerous industries.
From manufacturing to healthcare, the ability to design adaptive, data-informed controllers is proving invaluable.
Manufacturing and Automation
In manufacturing, systems and equipment need to operate optimally at all times.
FRIT allows for controllers that can adapt to wear, supply chain variations, and operational changes by leveraging data from IoT devices and sensors.
This ensures minimal downtime and maximum efficiency.
Healthcare and Medical Devices
In healthcare, precise control and prediction are vital—especially for life-supporting equipment.
FRIT-based designs can adapt to patient-specific data, offering personalized treatments and timely interventions.
Automotive and Aerospace Industries
Both the automotive and aerospace sectors face demanding environments requiring robust control systems.
Data-driven designs can cater to changing operational conditions, ensuring safety and performance over the vehicle’s or aircraft’s lifespan.
Conclusion: The Future of Controller Design
FRIT represents a paradigm shift in how controllers are designed, emphasizing the importance of using real system data.
While challenges remain, the benefits of adaptability and decreased reliance on static models hold significant promise.
By integrating predictive techniques, engineers can elevate system performance to new heights, ensuring smarter, more responsive technologies.
As industries continue to harness and perfect these methods, the potential for innovation and efficiency remains boundless.
資料ダウンロード
QCD調達購買管理クラウド「newji」は、調達購買部門で必要なQCD管理全てを備えた、現場特化型兼クラウド型の今世紀最高の購買管理システムとなります。
ユーザー登録
調達購買業務の効率化だけでなく、システムを導入することで、コスト削減や製品・資材のステータス可視化のほか、属人化していた購買情報の共有化による内部不正防止や統制にも役立ちます。
NEWJI DX
製造業に特化したデジタルトランスフォーメーション(DX)の実現を目指す請負開発型のコンサルティングサービスです。AI、iPaaS、および先端の技術を駆使して、製造プロセスの効率化、業務効率化、チームワーク強化、コスト削減、品質向上を実現します。このサービスは、製造業の課題を深く理解し、それに対する最適なデジタルソリューションを提供することで、企業が持続的な成長とイノベーションを達成できるようサポートします。
オンライン講座
製造業、主に購買・調達部門にお勤めの方々に向けた情報を配信しております。
新任の方やベテランの方、管理職を対象とした幅広いコンテンツをご用意しております。
お問い合わせ
コストダウンが利益に直結する術だと理解していても、なかなか前に進めることができない状況。そんな時は、newjiのコストダウン自動化機能で大きく利益貢献しよう!
(Β版非公開)