- お役立ち記事
- Fundamentals of data analysis using deep learning for optical measurement and optimization for optical data analysis
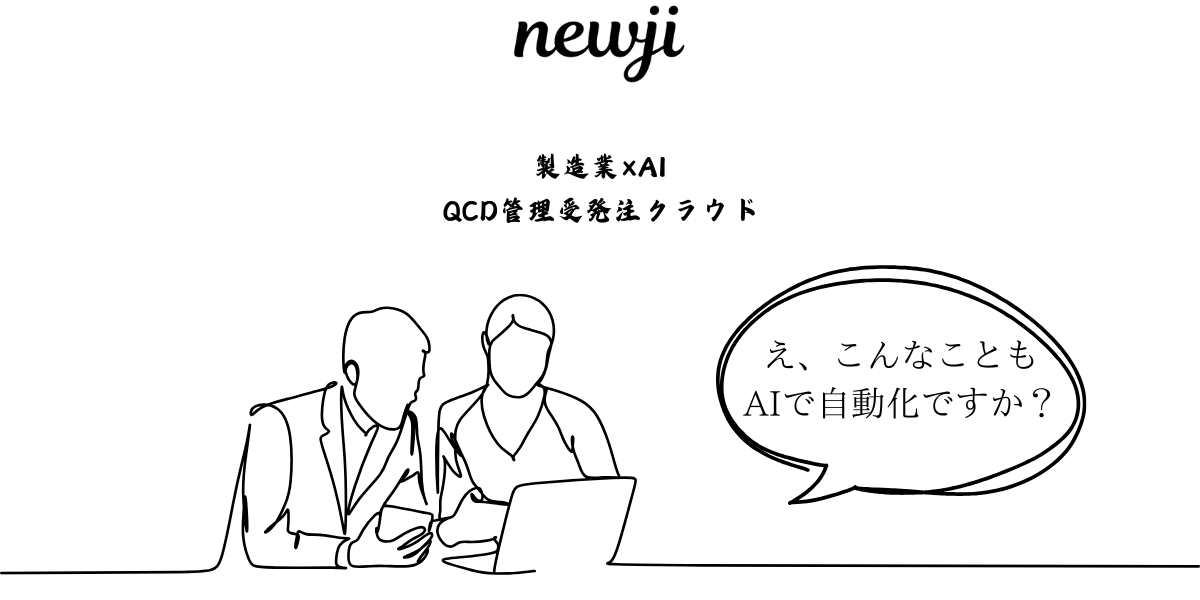
Fundamentals of data analysis using deep learning for optical measurement and optimization for optical data analysis
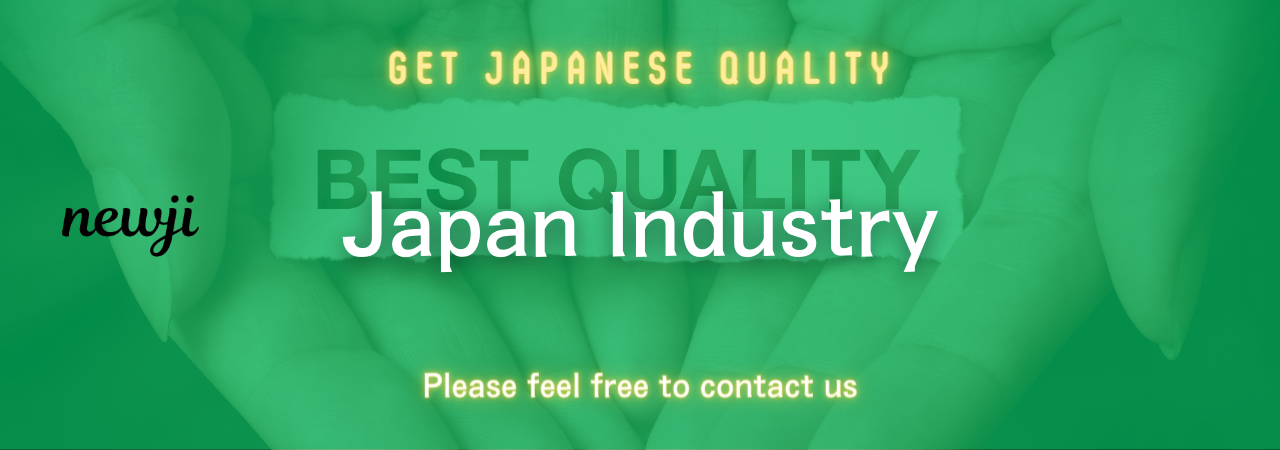
目次
Introduction to Deep Learning for Optical Measurement
Deep learning, a subset of artificial intelligence, has revolutionized numerous industries, including optical measurement.
Optical measurement involves analyzing light to gather data, and with the advent of deep learning, the precision and efficiency of this field have significantly improved.
This article delves into the fundamentals of data analysis using deep learning for optical measurement and explores optimization techniques for optical data analysis.
Understanding Optical Measurement
Optical measurement is a technique used to determine the characteristics of objects or systems by analyzing how light interacts with them.
It can include measuring dimensions, surface qualities, and even chemical compositions.
These measurements are crucial in industries such as manufacturing, healthcare, and telecommunications.
Traditionally, optical measurement relied on manual methods or basic algorithmic processes, which could be time-consuming and prone to errors.
Deep learning has emerged as a powerful tool to automate and enhance these processes, leading to more accurate and reliable results.
Introduction to Deep Learning
Deep learning is a subset of machine learning that uses neural networks with multiple layers to model and understand complex patterns in data.
This approach mimics the human brain’s neural networks, allowing systems to learn and make decisions independently.
For optical measurement, deep learning algorithms can process vast amounts of optical data, identify patterns, and provide insights that would be impossible or too labor-intensive for humans to discern manually.
The Role of Neural Networks in Optical Measurement
Neural networks play a crucial role in the application of deep learning for optical measurement.
These networks consist of layers of interconnected nodes or “neurons,” each performing simple calculations.
When these layers are stacked, they can model complex patterns and relationships in data.
For optical data, neural networks can be trained to recognize specific patterns or features, such as the size and shape of an object or variations in surface texture.
Once trained, they can quickly analyze new data and provide accurate predictions or measurements.
Data Preprocessing for Deep Learning
Before feeding data into a deep learning model, it needs to be preprocessed to ensure accuracy and efficiency.
Preprocessing involves cleaning the data, normalizing it, and sometimes augmenting it to create more robust datasets.
For optical data, preprocessing might include adjusting for variations in lighting, removing noise, and enhancing features that are important for analysis.
These steps help the deep learning model to focus on relevant features and improve its performance.
Applications of Deep Learning in Optical Measurement
The applications of deep learning in optical measurement are vast and varied, ranging from industrial to medical settings.
Quality Control in Manufacturing
In manufacturing, optical measurement is used extensively for quality control.
Deep learning models can rapidly analyze images or scans of products to detect defects, measure dimensions, and ensure compliance with specifications.
These systems can operate continuously, providing real-time feedback to production lines and significantly reducing the incidence of defective products.
Medical Imaging
In healthcare, optical measurement plays a crucial role in medical imaging, including procedures like MRI and CT scans.
Deep learning enhances these processes by improving image interpretation, identifying abnormalities, and assisting in diagnostics.
For example, deep learning models can be used to analyze images for early signs of diseases such as cancer, enabling timely and accurate treatment.
Telecommunications
In telecommunications, optical measurement ensures the efficiency and reliability of optical fibers used in data transmission.
Deep learning can optimize the analysis of fiber optic signals, detecting issues such as signal degradation or breakage, thereby enhancing network performance.
Optimization Techniques for Optical Data Analysis
To fully leverage the benefits of deep learning in optical measurement, optimization techniques must be applied to improve the accuracy and efficiency of data analysis.
Hyperparameter Tuning
Hyperparameters are settings that define the architecture of a neural network, such as the number of layers and learning rate.
Tuning these parameters can significantly impact the performance of a deep learning model.
By systematically adjusting hyperparameters, models can be optimized to achieve better accuracy and faster processing times.
Transfer Learning
Transfer learning is a technique that involves using a pre-trained model on a similar task to improve the performance on a new, but related, task.
This method saves time and computational resources by leveraging existing learning rather than training a model from scratch.
In optical measurement, transfer learning can be particularly effective in adapting models to new measurement scenarios or different types of optical data.
Data Augmentation
Data augmentation involves artificially expanding a dataset by creating new modified versions of existing data.
This practice prevents overfitting, where a model performs well on training data but poorly on new data.
Incorporating data augmentation in optical measurement ensures that deep learning models remain robust and generalize well across different datasets.
Conclusion
Deep learning has significantly transformed the field of optical measurement, improving accuracy, efficiency, and reliability.
By understanding the fundamentals of deep learning and employing optimization techniques, industries can enhance their optical data analysis processes, resulting in better quality control, accurate diagnostics, and reliable data transmissions.
As technology continues to evolve, the integration of deep learning in optical measurement will undoubtedly lead to further advancements and innovations, shaping the future of numerous sectors.
資料ダウンロード
QCD調達購買管理クラウド「newji」は、調達購買部門で必要なQCD管理全てを備えた、現場特化型兼クラウド型の今世紀最高の購買管理システムとなります。
ユーザー登録
調達購買業務の効率化だけでなく、システムを導入することで、コスト削減や製品・資材のステータス可視化のほか、属人化していた購買情報の共有化による内部不正防止や統制にも役立ちます。
NEWJI DX
製造業に特化したデジタルトランスフォーメーション(DX)の実現を目指す請負開発型のコンサルティングサービスです。AI、iPaaS、および先端の技術を駆使して、製造プロセスの効率化、業務効率化、チームワーク強化、コスト削減、品質向上を実現します。このサービスは、製造業の課題を深く理解し、それに対する最適なデジタルソリューションを提供することで、企業が持続的な成長とイノベーションを達成できるようサポートします。
オンライン講座
製造業、主に購買・調達部門にお勤めの方々に向けた情報を配信しております。
新任の方やベテランの方、管理職を対象とした幅広いコンテンツをご用意しております。
お問い合わせ
コストダウンが利益に直結する術だと理解していても、なかなか前に進めることができない状況。そんな時は、newjiのコストダウン自動化機能で大きく利益貢献しよう!
(Β版非公開)