- お役立ち記事
- Fundamentals of data analysis using deep learning for optical measurement and optimization for optical data analysis
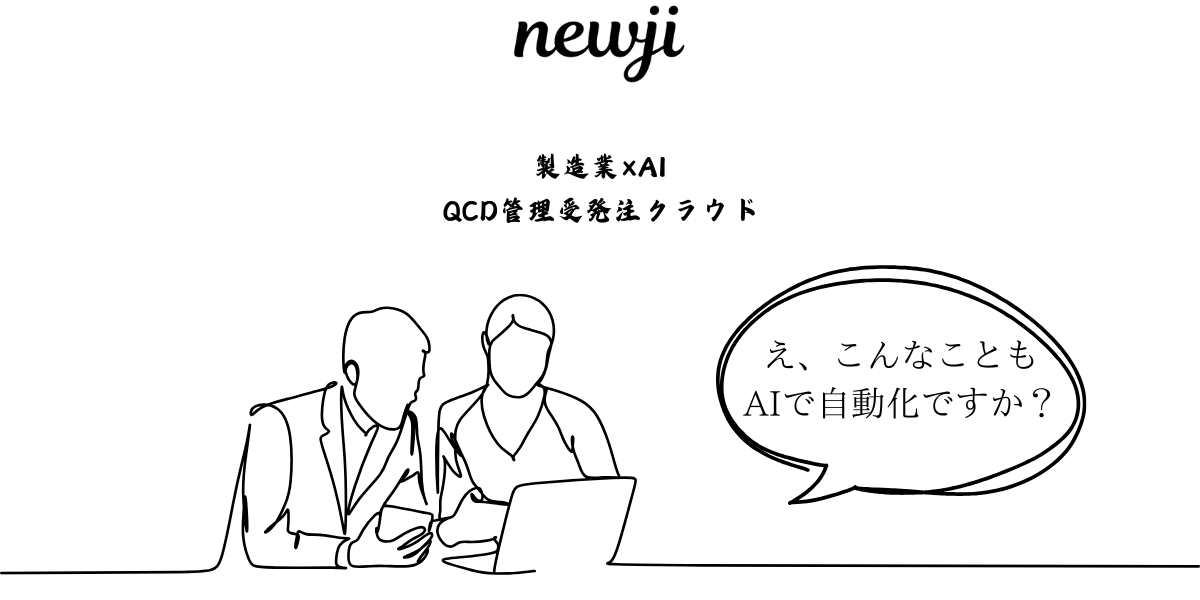
Fundamentals of data analysis using deep learning for optical measurement and optimization for optical data analysis
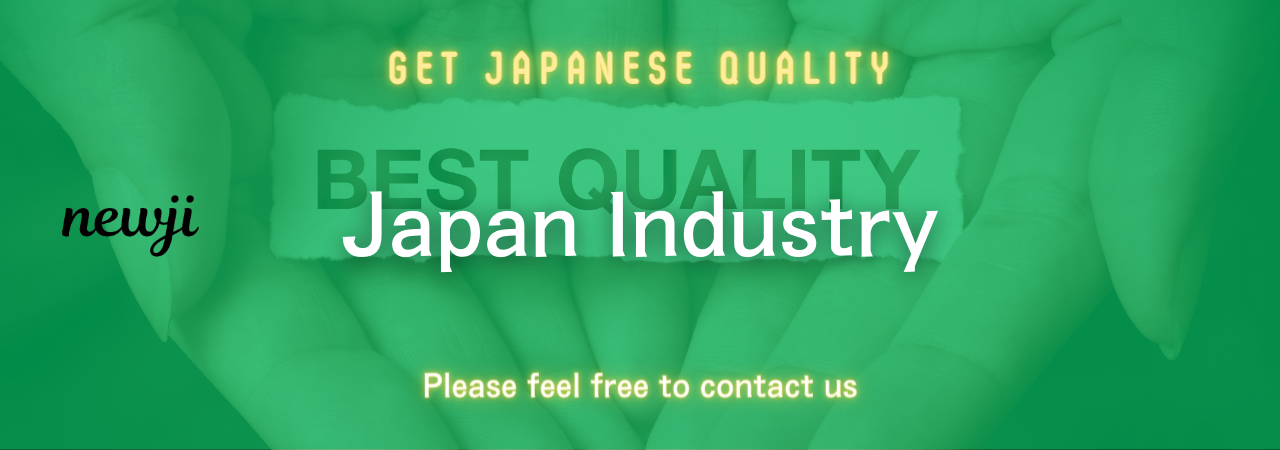
目次
Understanding Deep Learning in Optical Measurement
Deep learning is a fascinating and powerful tool in the world of data analysis.
It has the ability to learn patterns and make predictions based on data, much like our own brains.
One of the fields where deep learning has had a significant impact is optical measurement.
Optical measurement involves capturing and analyzing visual information, such as images and light patterns.
When deep learning techniques are applied, it enhances our ability to interpret and utilize this information effectively.
What is Optical Measurement?
Optical measurement is a technique used to gather and analyze data from light-based systems.
This can include anything from using cameras to capture images, to using lasers to measure distances or detect changes in the environment.
Such measurements are crucial in several industries like manufacturing, healthcare, and telecommunications, where precision and accuracy are key.
How Deep Learning Enhances Optical Measurement
Deep learning enhances optical measurement by improving the accuracy and efficiency of data processing.
Traditional techniques often require manual intervention and can be time-consuming.
But with deep learning, systems can learn from large amounts of data and automate the measurement process.
This not only speeds up analysis but also reduces the potential for human error.
For example, in a factory setting, deep learning can be used to inspect products for defects.
Cameras capture images of the products, and deep learning algorithms analyze these images quickly.
The system can then determine whether a product meets quality standards or needs to be rejected.
This kind of automation ensures consistency and enhances production quality.
Key Components of Deep Learning for Optical Analysis
Several components are crucial when applying deep learning to optical measurement.
These include neural networks, data sets, and computational power.
Neural Networks
Neural networks are at the heart of deep learning.
They are computational models inspired by the way the human brain works, consisting of layers of interconnected nodes or “neurons”.
These networks are capable of learning patterns and making predictions from data inputs.
In optical measurement, convolutional neural networks (CNNs) are frequently used due to their ability to process and analyze image data efficiently.
They are adept at recognizing patterns within the vast array of pixels that make up digital images.
Data Sets
Data sets are collections of data that the neural network learns from.
In the context of optical measurement, these data sets usually consist of images or sensor readings.
Properly annotated and diverse data sets are crucial for training deep learning models to ensure they are robust and accurate in their predictions.
When crafting data sets, quality and quantity matter.
Large data sets expose the neural network to a wide variety of scenarios, helping it learn and generalize better.
However, the data must also be representative of real-world conditions to ensure the model’s applicability.
Computational Power
Deep learning models require substantial computational power to train and execute.
This is due to the extensive calculations needed when processing large data sets and adjusting the neural network’s weights during training.
The rise of powerful graphics processing units (GPUs) has been a game-changer in this respect, allowing for faster and more efficient deep learning processes.
Optimization Techniques for Optical Data Analysis
Optimization is a critical aspect of deploying deep learning in optical measurement.
Effective optimization ensures that models run efficiently and provide accurate results.
Fine-Tuning Models
Fine-tuning involves making adjustments to a pre-trained deep learning model to better fit a specific task or data set.
This process saves time and computational resources, as it builds on existing knowledge rather than starting from scratch.
In optical measurement, fine-tuning a model might involve adjusting it to recognize specific patterns relevant to a particular application or industry.
Hyperparameter Tuning
Hyperparameters are settings that influence the training process of a deep learning model.
These can include the learning rate, the number of layers in a neural network, and other architectural choices.
Finding the optimal combination of hyperparameters is crucial for maximizing a model’s performance and ensuring fast, accurate data analysis.
Data Augmentation
Data augmentation is a technique used to increase the diversity of data available for training without actually collecting new data.
This can involve rotating images, adding noise, or altering brightness levels in optical measurement data sets.
Such techniques make models more robust by exposing them to varied data instances.
The Future of Deep Learning in Optical Measurement
Deep learning continues to evolve and expand its impact on optical measurement.
With advancements in technology, its applications are becoming more sophisticated and widespread.
For instance, in the field of autonomous vehicles, optical measurement with deep learning is being used to analyze and interpret road environments in real-time.
Moreover, as deep learning models become more efficient, they will be easier to deploy in smaller devices.
This opens up possibilities for advancements in mobile technology and wearable devices, where optical sensors can provide invaluable health and environmental data.
In conclusion, deep learning is a transformative force in optical measurement, optimizing data analysis processes and driving innovation across various industries.
By understanding its fundamental components and optimization techniques, we can better harness its potential to solve complex problems and improve our interaction with the world around us.
資料ダウンロード
QCD調達購買管理クラウド「newji」は、調達購買部門で必要なQCD管理全てを備えた、現場特化型兼クラウド型の今世紀最高の購買管理システムとなります。
ユーザー登録
調達購買業務の効率化だけでなく、システムを導入することで、コスト削減や製品・資材のステータス可視化のほか、属人化していた購買情報の共有化による内部不正防止や統制にも役立ちます。
NEWJI DX
製造業に特化したデジタルトランスフォーメーション(DX)の実現を目指す請負開発型のコンサルティングサービスです。AI、iPaaS、および先端の技術を駆使して、製造プロセスの効率化、業務効率化、チームワーク強化、コスト削減、品質向上を実現します。このサービスは、製造業の課題を深く理解し、それに対する最適なデジタルソリューションを提供することで、企業が持続的な成長とイノベーションを達成できるようサポートします。
オンライン講座
製造業、主に購買・調達部門にお勤めの方々に向けた情報を配信しております。
新任の方やベテランの方、管理職を対象とした幅広いコンテンツをご用意しております。
お問い合わせ
コストダウンが利益に直結する術だと理解していても、なかなか前に進めることができない状況。そんな時は、newjiのコストダウン自動化機能で大きく利益貢献しよう!
(Β版非公開)