- お役立ち記事
- Fundamentals of deep learning and applied technology to high-precision image recognition systems
Fundamentals of deep learning and applied technology to high-precision image recognition systems
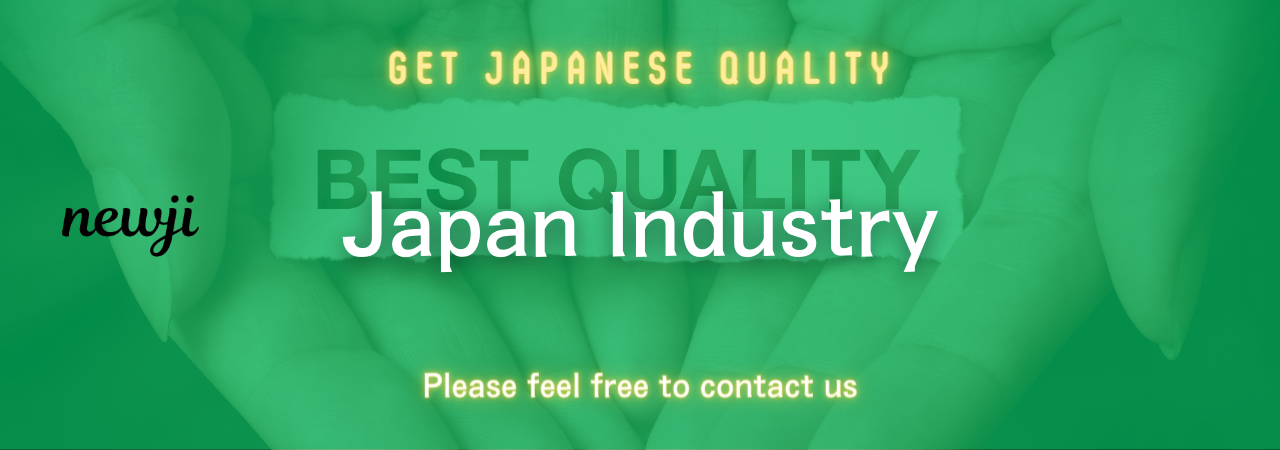
目次
Understanding Deep Learning
Deep learning is a subset of artificial intelligence (AI) that mimics the workings of the human brain to process data, create patterns, and make decisions.
At its core, deep learning uses neural networks with three or more layers to analyze data at multiple levels.
This approach enables machines to perform tasks such as image recognition and natural language processing with impressive accuracy.
Unlike traditional machine learning algorithms, deep learning models continuously analyze data to identify patterns.
The more the model is exposed to data, the more it improves its accuracy.
The intricate structure of neural networks, coupled with vast amounts of training data, allows deep learning to excel in tasks that require high precision.
The Significance of Image Recognition Systems
Image recognition is a technology that enables digital devices to identify and process images in the same way that human vision works.
This technology has a myriad of applications, from facial recognition in security systems to object detection in autonomous vehicles.
High-precision image recognition systems are crucial in today’s tech-driven world.
They transform how industries operate by offering automation solutions and enhancing user experiences.
For example, in healthcare, advanced image recognition systems assist radiologists in identifying anomalies in medical images, which can lead to earlier diagnosis and treatment of diseases.
How Deep Learning Enhances Image Recognition
Deep learning is at the heart of modern image recognition systems.
These systems are capable of learning complex patterns and features from raw image data through convolutional neural networks (CNNs).
A CNN’s architecture is designed to mimic the visual cortex, the part of the brain responsible for processing visual information.
Using multiple layers of neurons, CNNs automatically learn to adapt to the intricacies of visual data.
The first layers might detect simple edges and shapes, while deeper layers recognize complex structures and patterns.
This hierarchical learning process is what allows deep learning models to achieve high levels of accuracy in image classification and object detection tasks.
Challenges in High-Precision Image Recognition
Despite its potential, implementing deep learning in image recognition does come with its challenges.
One major hurdle is the need for vast amounts of labeled data to train these models effectively.
Acquiring such data can be resource-intensive and time-consuming.
Furthermore, deep learning models can require significant computational power.
Training a model demands advanced hardware, such as GPUs, and can take days or even weeks, depending on the complexity of the task.
Another challenge is the possibility of overfitting.
A model that performs exceptionally well on training data might fail to generalize and perform accurately on new, unseen data.
To combat this, researchers employ techniques like data augmentation and regularization to ensure that the model remains robust and versatile.
Solutions and Innovations
To address the data challenge, the field is seeing a shift towards semi-supervised and unsupervised learning approaches.
These techniques use fewer labeled data and leverage large amounts of unlabeled data to improve model accuracy.
The rise of transfer learning is another exciting development in deep learning.
In transfer learning, a model trained on a large dataset is fine-tuned on a smaller, specific dataset.
This allows developers to create high-precision models more efficiently, saving both time and resources.
Advancements in hardware have also facilitated deep learning’s growth.
Innovations in GPUs and the development of specialized AI accelerators have drastically reduced training times.
Merging deep learning with other technologies, such as edge computing, is paving the way for real-time image recognition.
This combination allows the processing of data closer to the source, reducing latency and improving the efficiency of image recognition systems.
Applications of High-Precision Image Recognition Systems
Deep learning-driven image recognition systems have permeated various sectors.
In security, facial recognition technology enhances surveillance systems, helping to verify identities with unmatched precision.
In the automotive industry, camera-based systems use image recognition to detect objects on the road, enabling features like automatic braking and lane-keeping in autonomous vehicles.
These systems are crucial for ensuring passenger safety and enhancing the performance of driverless cars.
Retailers utilize image recognition to personalize shopping experiences.
By analyzing customer images, stores can recommend products and even enable cashier-less checkouts.
Such innovations improve customer satisfaction and streamline retail operations.
Healthcare benefits significantly from high-precision image recognition systems.
They assist in analyzing medical images like X-rays, MRIs, and CT scans, offering doctors a powerful tool for early disease detection and accurate diagnosis.
The Future of Deep Learning in Image Recognition
The future of deep learning and its application to image recognition is promising.
With ongoing research and technological advancements, we’re likely to see even more precise and efficient systems.
As AI ethics continue to evolve, it’s essential to ensure that these systems are designed and deployed responsibly.
Privacy and fairness must be prioritized to build public trust and maximize the benefits of image recognition technologies.
Collaboration across different sectors, along with continuous innovation, will drive the enhanced implementation of deep learning in image recognition systems.
With increased accuracy and efficiency, these systems will undoubtedly play a pivotal role in the future of technological development.
資料ダウンロード
QCD調達購買管理クラウド「newji」は、調達購買部門で必要なQCD管理全てを備えた、現場特化型兼クラウド型の今世紀最高の購買管理システムとなります。
ユーザー登録
調達購買業務の効率化だけでなく、システムを導入することで、コスト削減や製品・資材のステータス可視化のほか、属人化していた購買情報の共有化による内部不正防止や統制にも役立ちます。
NEWJI DX
製造業に特化したデジタルトランスフォーメーション(DX)の実現を目指す請負開発型のコンサルティングサービスです。AI、iPaaS、および先端の技術を駆使して、製造プロセスの効率化、業務効率化、チームワーク強化、コスト削減、品質向上を実現します。このサービスは、製造業の課題を深く理解し、それに対する最適なデジタルソリューションを提供することで、企業が持続的な成長とイノベーションを達成できるようサポートします。
オンライン講座
製造業、主に購買・調達部門にお勤めの方々に向けた情報を配信しております。
新任の方やベテランの方、管理職を対象とした幅広いコンテンツをご用意しております。
お問い合わせ
コストダウンが利益に直結する術だと理解していても、なかなか前に進めることができない状況。そんな時は、newjiのコストダウン自動化機能で大きく利益貢献しよう!
(Β版非公開)