- お役立ち記事
- Fundamentals of deep learning programming using Python, its applications and implementation
月間76,176名の
製造業ご担当者様が閲覧しています*
*2025年3月31日現在のGoogle Analyticsのデータより
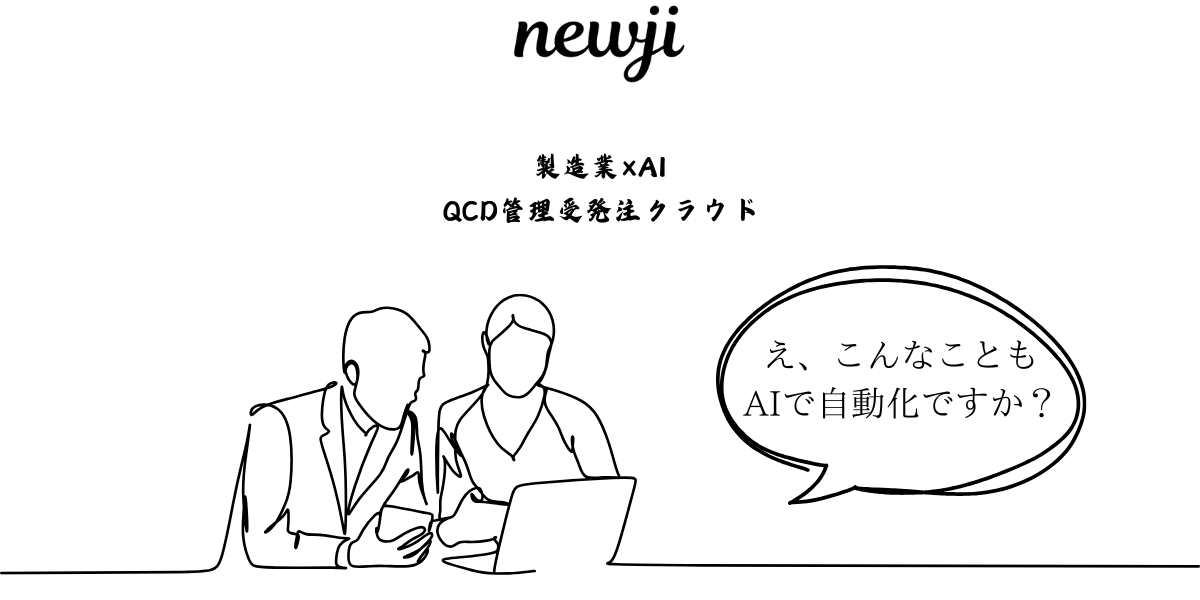
Fundamentals of deep learning programming using Python, its applications and implementation
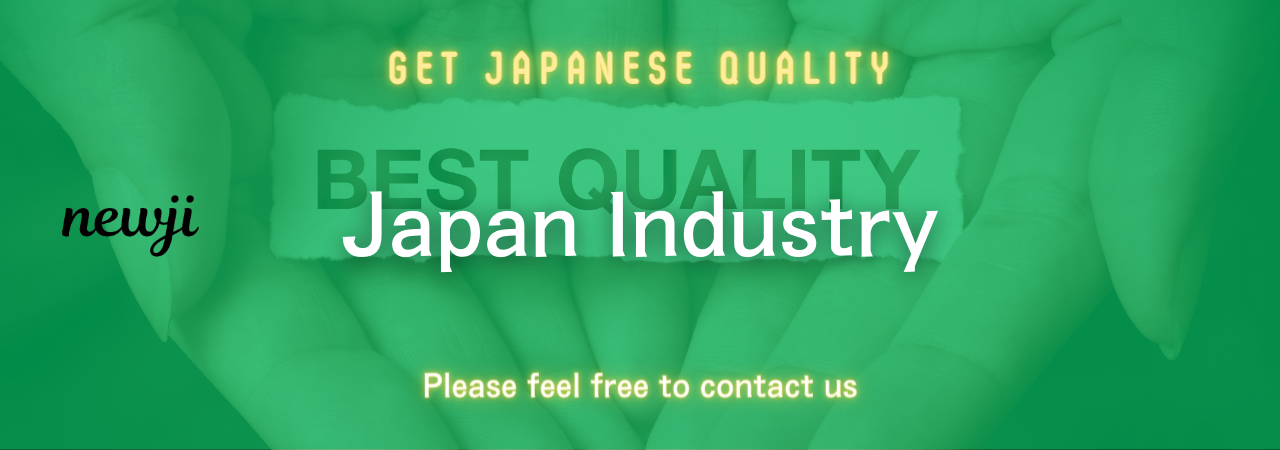
目次
Understanding Deep Learning
Deep learning is a subset of machine learning that uses neural networks with three or more layers to simulate human decision-making.
It enables systems to learn from data, recognize patterns, and make decisions with minimal human intervention.
The deep learning models aim to learn complex patterns and relationships in data by automatically adjusting the connections among neurons in the neural network.
This sophisticated method has revolutionized industries, offering unparalleled opportunities in fields such as computer vision, natural language processing, and more.
The key to unlocking the potential of deep learning lies in its programming foundation, often built upon Python, a versatile and user-friendly language.
The Role of Python in Deep Learning
Python is a powerful programming language that has become the backbone of deep learning development.
Its concise syntax, extensive libraries, and vibrant community make it ideal for both beginners and experts.
Python’s libraries such as TensorFlow, Keras, and PyTorch simplify the implementation of complex deep learning models.
These libraries provide pre-built classes and functions that reduce the complexity of deep learning algorithms, allowing developers to focus on model efficacy and deployment.
Furthermore, Python’s compatibility with other tools and platforms encourages innovation and collaboration in the deep learning ecosystem.
Implementing Deep Learning with Python
To implement deep learning with Python, one needs to follow a structured approach that involves several steps.
First, data collection and preprocessing are crucial stages that ensure the quality of input data.
Python libraries such as NumPy and Pandas aid in handling large datasets, while libraries like OpenCV and Pillow offer image processing capabilities.
Next, model selection and architecture design involve choosing the right type of neural network model depending on the problem at hand.
Convolutional Neural Networks (CNNs) are typically used for image-related tasks, while Recurrent Neural Networks (RNNs) are favored for sequential data.
Python’s deep learning libraries offer predefined architectures to facilitate this process.
Training the deep learning model requires selecting appropriate hyperparameters and employing optimization techniques.
Using Python, you can implement training algorithms such as backpropagation with minimal code.
Validation and testing follow, where the model’s performance is evaluated with unseen data to ensure it generalizes well.
Finally, deployment is the last step, where the trained model is integrated into an application.
Python supports various APIs and frameworks to streamline the deployment process.
Applications of Deep Learning
Deep learning has a wide range of applications across multiple industries.
In healthcare, it aids in medical image analysis, detecting patterns that may be unnoticeable to the human eye, supporting early diagnosis and treatment decisions.
Autonomous driving utilizes deep learning to process visual data, making real-time decisions for safe and efficient vehicle control.
In finance, deep learning models are applied to algorithmic trading, fraud detection, and risk management through understanding complex market signals and trends.
The entertainment industry benefits by using deep learning for content personalization, generating recommendations based on user behavior patterns.
Moreover, in agriculture, deep learning powers crop monitoring, soil analysis, and yield prediction, optimizing farming practices and resource usage.
Each application showcases deep learning’s transformative impact across various domains, leading to innovative solutions and enhanced productivity.
Challenges in Deep Learning Implementation
Despite its potential, deep learning comes with its own set of challenges.
Data availability and quality can significantly affect model performance, as deep learning algorithms require vast amounts of labeled data for training.
Model training is computationally intensive and often demands high-performance hardware, which can be costly.
Overfitting is another common issue, where a model performs well on training data but poorly on unseen data.
Balancing model complexity with generalization is crucial to overcoming this challenge.
Moreover, deep learning models are often seen as “black boxes,” lacking transparency in their decision-making process, which can hinder trust and understanding.
Continuous research and development are necessary to address these challenges, improve model explainability, and make deep learning equally adaptable for all applications.
The Future of Deep Learning
The future of deep learning is promising, with advancements on the horizon poised to address current limitations and unlock new possibilities.
Research is focusing on developing models that require less data while retaining high accuracy.
Efforts are also underway to improve model interpretability, making deep learning systems more transparent and accountable.
There is an increasing emphasis on edge computing, where models are optimized to run on edge devices, reducing the need for constant cloud connectivity and enhancing real-time processing.
These innovations will drive widespread adoption and integration of deep learning technologies into everyday life, pushing the boundaries of what is possible.
Python remains a critical player in this future landscape, providing the tools necessary to experiment, develop, and implement deep learning models efficiently.
As technology progresses, professionals armed with Python deep learning skills will be at the forefront of shaping the future, solving complex problems, and driving technological advancements.
資料ダウンロード
QCD管理受発注クラウド「newji」は、受発注部門で必要なQCD管理全てを備えた、現場特化型兼クラウド型の今世紀最高の受発注管理システムとなります。
ユーザー登録
受発注業務の効率化だけでなく、システムを導入することで、コスト削減や製品・資材のステータス可視化のほか、属人化していた受発注情報の共有化による内部不正防止や統制にも役立ちます。
NEWJI DX
製造業に特化したデジタルトランスフォーメーション(DX)の実現を目指す請負開発型のコンサルティングサービスです。AI、iPaaS、および先端の技術を駆使して、製造プロセスの効率化、業務効率化、チームワーク強化、コスト削減、品質向上を実現します。このサービスは、製造業の課題を深く理解し、それに対する最適なデジタルソリューションを提供することで、企業が持続的な成長とイノベーションを達成できるようサポートします。
製造業ニュース解説
製造業、主に購買・調達部門にお勤めの方々に向けた情報を配信しております。
新任の方やベテランの方、管理職を対象とした幅広いコンテンツをご用意しております。
お問い合わせ
コストダウンが利益に直結する術だと理解していても、なかなか前に進めることができない状況。そんな時は、newjiのコストダウン自動化機能で大きく利益貢献しよう!
(β版非公開)