- お役立ち記事
- Fundamentals of digital twin and data assimilation, model creation methods, and their applications
Fundamentals of digital twin and data assimilation, model creation methods, and their applications
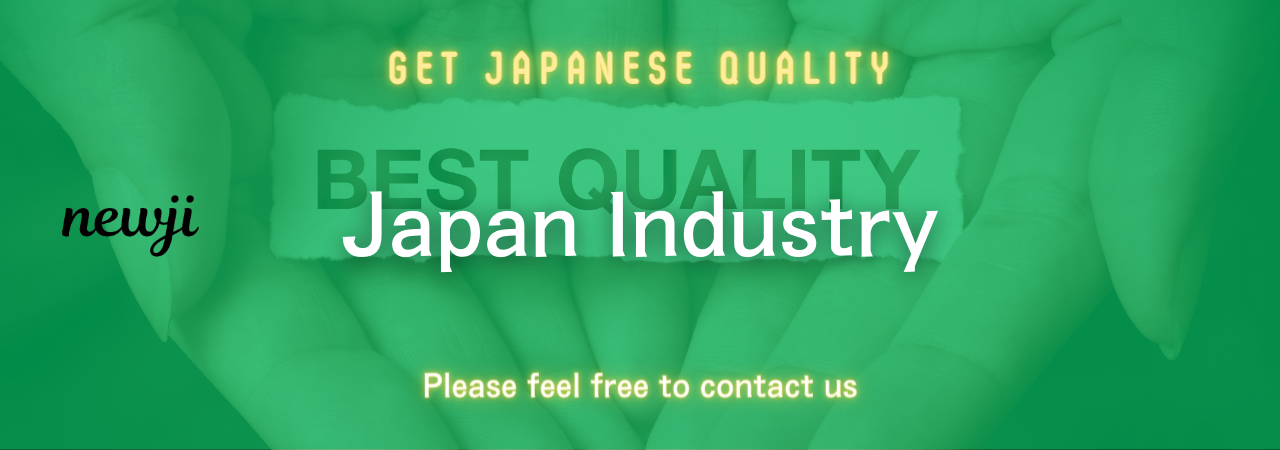
目次
Understanding Digital Twins
Digital twins are virtual replicas of physical systems, allowing us to monitor, analyze, and optimize these systems in real time.
This concept has grown in prominence with advancements in IoT, data analytics, and artificial intelligence.
The rise of Industry 4.0 has further accelerated the adoption of digital twins as industries strive for efficiency and innovation.
By leveraging these virtual models, businesses can predict outcomes, enhance operations, and improve product development.
What Are Digital Twins?
A digital twin is an exact digital copy of a physical entity.
This entity could be anything from a simple component to an entire manufacturing plant.
Digital twins operate by integrating real-time data from sensors, devices, and systems.
This data is then analyzed and visualized to provide actionable insights.
The benefits are manifold: improved operational efficiency, cost reduction, predictive maintenance, and enhanced customer satisfaction.
Data Assimilation in Digital Twin
Data assimilation is the merging of real-world measurements into a model to improve its accuracy and reliability.
In the context of digital twins, it involves the continuous integration of data streams to update the twin’s state.
By doing so, the digital twin remains a faithful representation of its physical counterpart.
Importance of Data Assimilation
Data assimilation ensures that digital twins remain relevant and reflective of the physical world’s current state.
Without it, the twin would quickly become outdated, rendering it ineffective for decision-making or predictive analytics.
Through assimilation, we can refine models, align them with reality, and thereby increase their value across various applications.
Techniques for Data Assimilation
Several methods exist for data assimilation, ranging from simple statistical techniques to advanced machine learning algorithms.
One common approach is the Kalman Filter, which continuously updates the estimate of the state of a process.
Another method is Particle Filtering, which uses a set of samples or particles to represent the probability distribution of a system.
Regardless of the technique, the goal remains the same: to ensure accuracy and reliability in digital representations.
Model Creation for Digital Twins
Creating a digital twin requires a robust model that accurately simulates the behavior and characteristics of its physical counterpart.
This involves a comprehensive understanding of both physics-based models and data-driven approaches.
Physics-Based Models
Physics-based models rely on the fundamental laws of physics to simulate how a system behaves.
These models are particularly useful for complex systems where the underlying processes are well understood.
For example, in aerospace, engineers use computational fluid dynamics (CFD) to model airflow around aircraft.
Data-Driven Models
In contrast, data-driven models leverage large datasets to identify patterns and make predictions about system behavior.
These models can take the form of machine learning algorithms trained on historical data.
Machine learning’s ability to uncover hidden patterns makes data-driven models particularly powerful in environments with intricate dynamics or sparse theoretical understanding.
Hybrid Models
To harness the best of both worlds, hybrid models integrate physics-based and data-driven methods.
By doing so, they offer a comprehensive approach that maximizes the accuracy and adaptability of the digital twin.
For instance, a hybrid model might use physics-based simulations to understand known processes while incorporating data-driven insights for unknown parameters or external influences.
Applications of Digital Twins
The application of digital twins spans across industries, each capitalizing on its capabilities to transform operations and innovation.
From manufacturing to healthcare, the potential for digital twins is vast and far-reaching.
Manufacturing
In manufacturing, digital twins are increasingly used to streamline production processes, anticipate machine failures, and enhance product design.
By simulating production lines, manufacturers can identify bottlenecks, optimize resource allocation, and maintain quality control.
Predictive maintenance is another significant application, allowing companies to prevent equipment failures before they occur, thus minimizing downtime.
Healthcare
Digital twins are making significant breakthroughs in healthcare, particularly in personalized medicine and patient care.
By creating a virtual model of a patient, healthcare professionals can tailor treatments, predict outcomes, and simulate surgical procedures.
This personalized approach enhances patient outcomes and optimizes healthcare delivery.
Urban Planning
For urban planning, digital twins can model city layouts, infrastructure, and services.
By simulating traffic patterns or energy consumption, planners can design better cities that are more sustainable and efficient.
These models also aid in disaster management by predicting the impact of natural events and optimizing response strategies.
Aerospace
The aerospace industry utilizes digital twins for the design, simulation, and monitoring of aircraft.
By modeling different flight scenarios, engineers can test and improve aircraft performance without the need for physical prototypes.
Digital twins also assist in fleet management by monitoring aircraft health and optimizing maintenance schedules.
The Future of Digital Twin Technology
The future of digital twin technology is promising, with continuous advancements in IoT, AI, and machine learning driving its evolution.
As these technologies mature, digital twins will become more sophisticated, accessible, and integrated into everyday operations.
Digital twins will increasingly support decision-making processes across industries, providing deeper insights and fostering innovation.
By enabling real-time simulations and predictions, they will unlock new possibilities in product development, operational efficiency, and customer engagement.
In conclusion, the fundamentals of digital twins, alongside data assimilation and model creation methods, are essential in leveraging this transformative technology.
As the digital and physical worlds converge, digital twins will undoubtedly play a pivotal role in shaping a smarter, more connected future.
資料ダウンロード
QCD調達購買管理クラウド「newji」は、調達購買部門で必要なQCD管理全てを備えた、現場特化型兼クラウド型の今世紀最高の購買管理システムとなります。
ユーザー登録
調達購買業務の効率化だけでなく、システムを導入することで、コスト削減や製品・資材のステータス可視化のほか、属人化していた購買情報の共有化による内部不正防止や統制にも役立ちます。
NEWJI DX
製造業に特化したデジタルトランスフォーメーション(DX)の実現を目指す請負開発型のコンサルティングサービスです。AI、iPaaS、および先端の技術を駆使して、製造プロセスの効率化、業務効率化、チームワーク強化、コスト削減、品質向上を実現します。このサービスは、製造業の課題を深く理解し、それに対する最適なデジタルソリューションを提供することで、企業が持続的な成長とイノベーションを達成できるようサポートします。
オンライン講座
製造業、主に購買・調達部門にお勤めの方々に向けた情報を配信しております。
新任の方やベテランの方、管理職を対象とした幅広いコンテンツをご用意しております。
お問い合わせ
コストダウンが利益に直結する術だと理解していても、なかなか前に進めることができない状況。そんな時は、newjiのコストダウン自動化機能で大きく利益貢献しよう!
(Β版非公開)