- お役立ち記事
- Fundamentals of experimental design and statistical analysis
Fundamentals of experimental design and statistical analysis
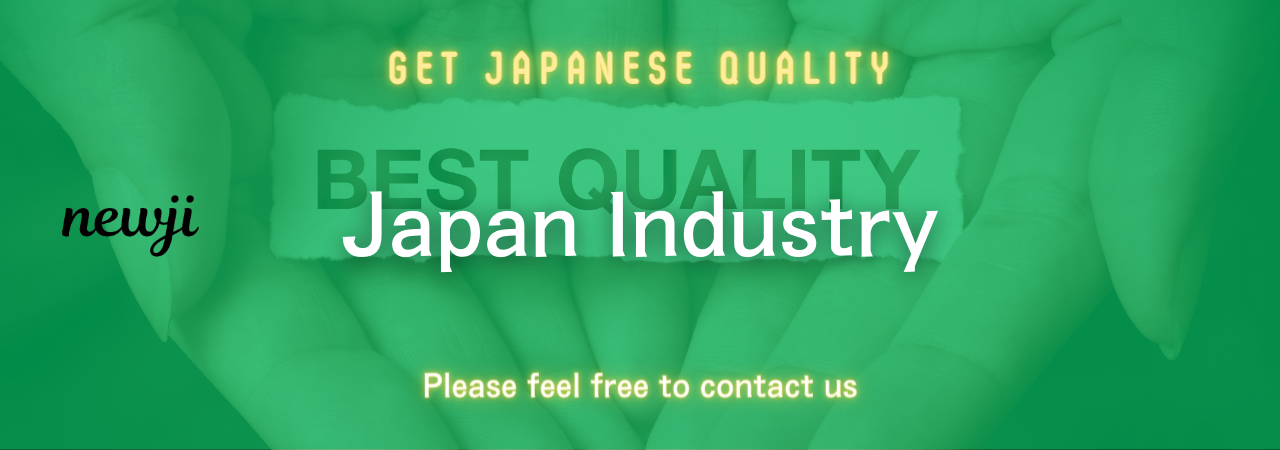
目次
Understanding Experimental Design
Experimental design is the blueprint for conducting a scientific experiment.
It involves organizing, planning, and structuring an experiment to ensure the results are valid, reliable, and can be reproduced.
A well-thought-out experimental design can help researchers minimize errors, control variables, and make accurate inferences about the relationship between variables.
To begin with, the main objective of experimental design is to establish a causal relationship between an independent variable and a dependent variable.
An independent variable is the one that is manipulated by the researcher, while the dependent variable is the outcome that is measured.
The researcher must ensure that any changes in the dependent variable are solely due to modifications in the independent variable.
There are several components that one must consider when designing an experiment.
These include defining the research question, formulating a hypothesis, selecting the experimental units, choosing the methods of data collection, and deciding on the data analysis techniques.
Each of these components plays a crucial role in determining the success of the experiment.
The Importance of Randomization
Randomization is a fundamental aspect of experimental design.
It involves assigning experimental units to different treatment groups using a random method.
The primary goal of randomization is to eliminate bias and ensure that the treatment groups are comparable at the beginning of the experiment.
Through randomization, researchers can evenly distribute both known and unknown confounding variables across the treatment groups.
Confounding variables are factors that may affect the dependent variable but are not of interest to the study.
By controlling for these variables, randomization helps to isolate the effect of the independent variable on the dependent variable.
Types of Experimental Designs
There are various types of experimental designs, each suited for different kinds of research questions and contexts.
Completely Randomized Design
In a completely randomized design, subjects are assigned to treatments entirely by chance.
This type of experimental design is simple and easy to implement, making it ideal for experiments where the variables are limited and control is easy.
However, it may not be suitable for experiments with a large number of variables or where it is essential to control more tightly for variability among experimental units.
Randomized Block Design
A randomized block design is an extension of the completely randomized design that includes blocking.
Blocking involves grouping experimental units that are similar in some way that is relevant to the response variable.
Within each block, treatments are randomly assigned.
This design reduces the impact of variability among experimental units that could obscure the treatment effect, providing a more precise estimate of the effect.
Factorial Design
Factorial design is used when researchers want to study the effect of two or more independent variables simultaneously.
In this design, treatments consist of all possible combinations of the levels of different factors.
This type of design allows researchers to evaluate not only the individual effects of each factor but also the interaction effects between them.
Statistical Analysis in Experimental Design
Statistical analysis is a critical component of experimental design as it helps in interpreting the data collected and making informed conclusions.
Several statistical methods can be employed depending on the research question and the design of the experiment.
Descriptive Statistics
Descriptive statistics provide a simple summary of the data collected in an experiment.
Measures such as mean, median, mode, standard deviation, and variance offer insights into the data distribution, central tendency, and variability.
Inferential Statistics
Inferential statistics enable researchers to make predictions or inferences about a population based on sample data.
Commonly used inferential statistical techniques include t-tests, analysis of variance (ANOVA), chi-square tests, and regression analysis.
These techniques help determine whether the observed differences between treatment groups are statistically significant.
Common Pitfalls in Experimental Design and Analysis
Despite the best efforts, researchers can sometimes fall into common pitfalls that affect the validity and reliability of their findings.
Avoiding Bias
Bias occurs when there is a systematic error in the experiment that influences the results.
It can arise from various sources, including selection bias, measurement bias, or response bias.
Researchers must be vigilant in identifying potential sources of bias and take steps to mitigate them.
Ensuring Adequate Sample Size
The sample size must be sufficiently large to detect an effect if it exists.
A small sample size can lead to underpowered studies, increasing the likelihood of Type II errors, where researchers fail to detect a true effect.
On the other hand, unnecessarily large sample sizes may waste resources and time.
Maintaining Sufficient Control
Controlling extraneous variables is essential to isolate the relationship between the independent and dependent variables.
Failure to do so can result in confounding, where the effect of the independent variable is mixed with other variables, leading to erroneous conclusions.
Conclusion
Understanding the fundamentals of experimental design and statistical analysis is crucial for conducting robust scientific research.
By thoughtfully planning and executing an experiment while employing appropriate statistical techniques, researchers can draw meaningful and reliable conclusions from their data.
Avoiding common pitfalls and remaining diligent in the design and analysis process ensures that the findings contribute significantly to the body of scientific knowledge.
資料ダウンロード
QCD調達購買管理クラウド「newji」は、調達購買部門で必要なQCD管理全てを備えた、現場特化型兼クラウド型の今世紀最高の購買管理システムとなります。
ユーザー登録
調達購買業務の効率化だけでなく、システムを導入することで、コスト削減や製品・資材のステータス可視化のほか、属人化していた購買情報の共有化による内部不正防止や統制にも役立ちます。
NEWJI DX
製造業に特化したデジタルトランスフォーメーション(DX)の実現を目指す請負開発型のコンサルティングサービスです。AI、iPaaS、および先端の技術を駆使して、製造プロセスの効率化、業務効率化、チームワーク強化、コスト削減、品質向上を実現します。このサービスは、製造業の課題を深く理解し、それに対する最適なデジタルソリューションを提供することで、企業が持続的な成長とイノベーションを達成できるようサポートします。
オンライン講座
製造業、主に購買・調達部門にお勤めの方々に向けた情報を配信しております。
新任の方やベテランの方、管理職を対象とした幅広いコンテンツをご用意しております。
お問い合わせ
コストダウンが利益に直結する術だと理解していても、なかなか前に進めることができない状況。そんな時は、newjiのコストダウン自動化機能で大きく利益貢献しよう!
(Β版非公開)