- お役立ち記事
- Fundamentals of explainable AI (XAI) and applications to modern models and systems
Fundamentals of explainable AI (XAI) and applications to modern models and systems
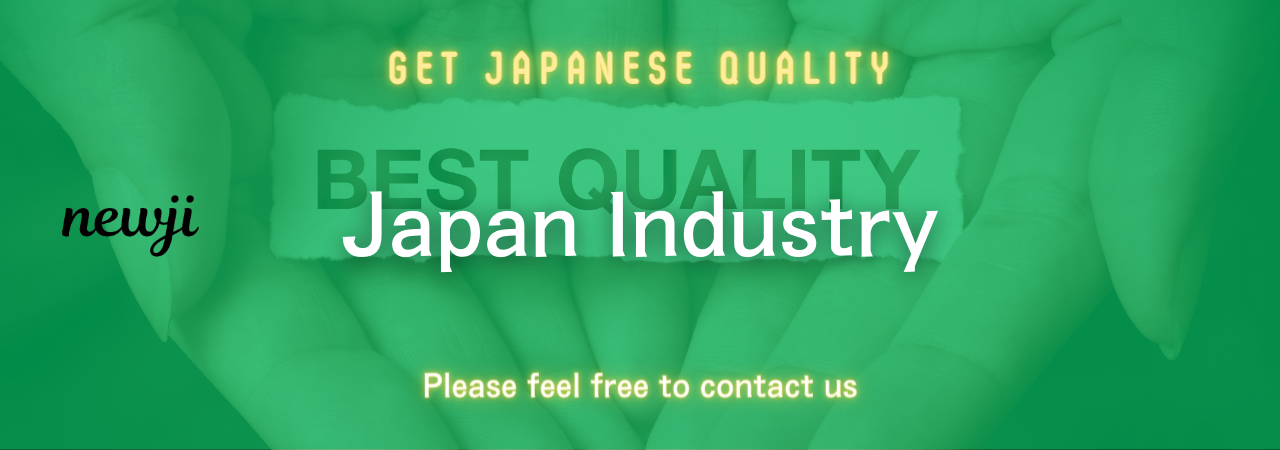
目次
Understanding Explainable AI (XAI)
Explainable AI (XAI) refers to artificial intelligence systems that are designed to be transparent in their decision-making processes.
Unlike traditional AI, which often operates like a “black box,” XAI allows humans to understand why and how an AI system arrives at specific conclusions.
The ability to explain AI models is crucial, especially in sensitive sectors like healthcare, finance, and law, where decisions can significantly impact individuals and society.
The Importance of Explainable AI
As AI becomes more integrated into daily life, the demand for transparency and trustworthiness in AI systems grows.
Explainability bridges the gap between complex machine operations and human understanding.
This, in turn, increases trust in AI systems and encourages their adoption across various industries.
Could one imagine allowing an unexplainable AI to dictate medical treatments without understanding the rationale behind the decisions?
Probably not.
This is why explainability is paramount in promoting the responsible use of AI.
Core Principles of Explainable AI
Transparency
Transparency in AI systems refers to the ability to access understandable information regarding how AI makes its predictions or decisions.
It includes understanding the inputs the system uses, the processes it employs, and how it weighs different factors to reach an outcome.
Interpretability
Interpretability deals with the extent to which a human can understand or predict the model’s output.
In other words, it’s about making AI models clear enough so that humans can grasp the reasoning behind their predictions.
Trustworthiness
Trust is built when AI systems provide consistent and reliable results.
When models are explainable, users can better evaluate the accuracy and fairness of the AI system, fostering greater reliance on its outcomes.
Applications of Explainable AI in Modern Models and Systems
Healthcare
In healthcare, explainable AI is used to support clinical decision-making.
For example, AI models that predict disease risks can accompany their predictions with reasons, such as past medical history or genetic data.
This helps healthcare professionals make better-informed decisions and understand any potential biases in the AI’s recommendations.
Finance
In the financial sector, explainable AI assists with fraud detection, credit scoring, and risk assessment.
Models used in credit scoring can show factors that influenced an individual’s creditworthiness, aiding in disputing errors or biases.
Furthermore, fraud detection algorithms can illustrate how certain transactions are flagged as suspicious, increasing the financial institution’s credibility and customer trust.
Legal Systems
In legal applications, AI systems can streamline procedures by providing explainable outcomes in document analysis, case law research, and predictive judgments.
Legal professionals can verify AI predictions with confidence knowing the rationale behind them, which reduces the risk of unjust decisions and enhances the efficiency of the legal process.
Challenges in Implementing Explainable AI
Complexity of AI Models
Some AI models, particularly deep learning and neural networks, are intrinsically complex, making their decision-making processes harder to decipher.
Balancing high model performance with interpretability remains a significant challenge for researchers.
Trade-offs between Accuracy and Explainability
Simplifying a model to make it explainable can sometimes come at the cost of accuracy.
Finding a balance where models are both accurate and explainable is ongoing work in the AI field.
Resistance to Change
Organizations might resist adopting explainable AI models due to the resources required to develop and integrate them.
AI practitioners need to champion the long-term benefits of explainability, such as reduced risk and enhanced credibility, to overcome such resistance.
The Future of Explainable AI
As AI systems evolve, the focus on making them explainable will only intensify.
Regulatory demands and consumer expectations will drive organizations to prioritize transparency in their AI endeavors.
Moreover, advancements in techniques for interpreting AI models, like SHAP (SHapley Additive exPlanations) values, are ongoing and will continue to enhance our understanding of AI systems.
Ultimately, integrating explainability into AI models will shape how society views and utilizes AI technology, fostering a future where AI systems are trusted allies across various domains.
資料ダウンロード
QCD調達購買管理クラウド「newji」は、調達購買部門で必要なQCD管理全てを備えた、現場特化型兼クラウド型の今世紀最高の購買管理システムとなります。
ユーザー登録
調達購買業務の効率化だけでなく、システムを導入することで、コスト削減や製品・資材のステータス可視化のほか、属人化していた購買情報の共有化による内部不正防止や統制にも役立ちます。
NEWJI DX
製造業に特化したデジタルトランスフォーメーション(DX)の実現を目指す請負開発型のコンサルティングサービスです。AI、iPaaS、および先端の技術を駆使して、製造プロセスの効率化、業務効率化、チームワーク強化、コスト削減、品質向上を実現します。このサービスは、製造業の課題を深く理解し、それに対する最適なデジタルソリューションを提供することで、企業が持続的な成長とイノベーションを達成できるようサポートします。
オンライン講座
製造業、主に購買・調達部門にお勤めの方々に向けた情報を配信しております。
新任の方やベテランの方、管理職を対象とした幅広いコンテンツをご用意しております。
お問い合わせ
コストダウンが利益に直結する術だと理解していても、なかなか前に進めることができない状況。そんな時は、newjiのコストダウン自動化機能で大きく利益貢献しよう!
(Β版非公開)