- お役立ち記事
- Fundamentals of fatigue damage in metal materials and application to fatigue life and strength prediction using AI (machine learning)
月間76,176名の
製造業ご担当者様が閲覧しています*
*2025年3月31日現在のGoogle Analyticsのデータより
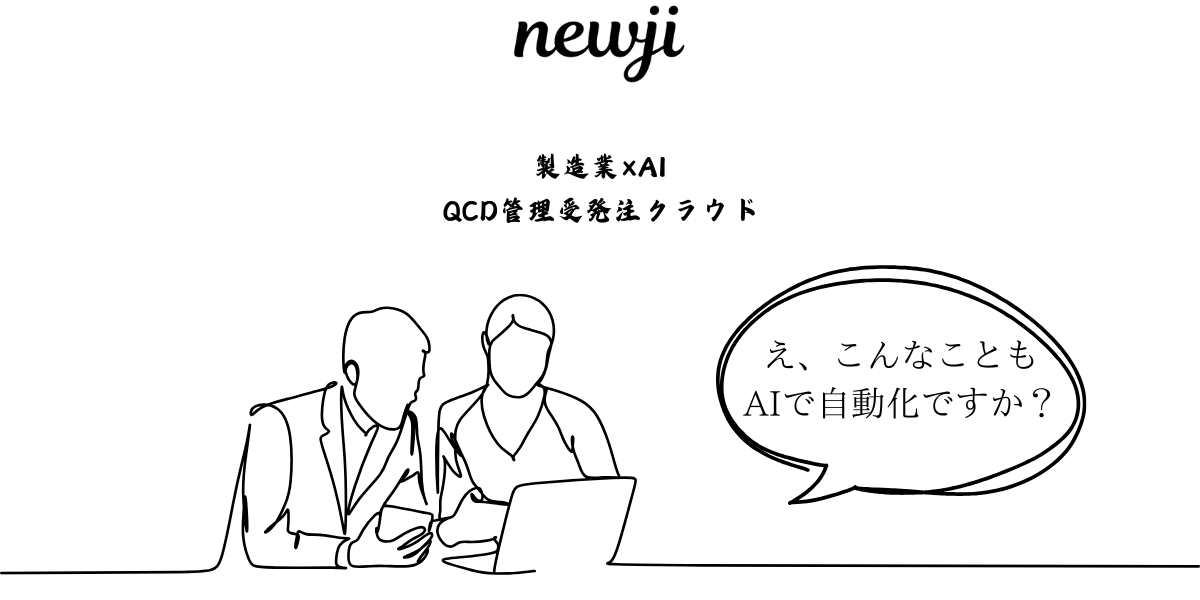
Fundamentals of fatigue damage in metal materials and application to fatigue life and strength prediction using AI (machine learning)
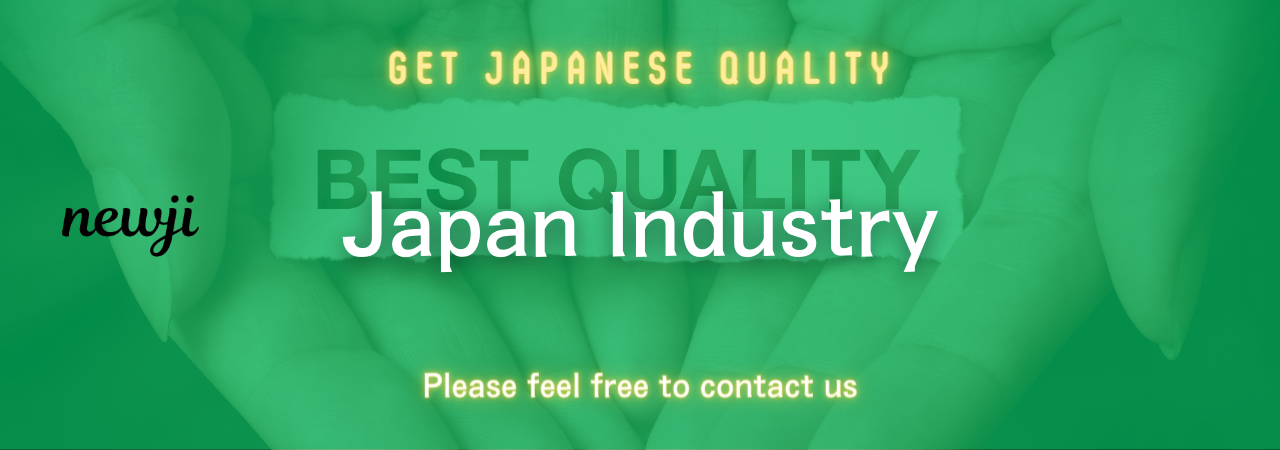
目次
Understanding Fatigue Damage in Metal Materials
Fatigue damage is a critical phenomenon that affects the lifespan and strength of metal materials.
It occurs when metal components are subjected to cyclic loading, which leads to the gradual accumulation of damage.
This can eventually result in catastrophic failure, particularly in applications where metal integrity is crucial, such as in aerospace, automotive, and construction industries.
The Basics of Fatigue Damage
Fatigue damage typically begins with the formation of micro-cracks at stress concentration points within the metal.
These stress concentrations occur due to irregularities in the metal structure, such as inclusions, voids, or surface defects.
As the metal undergoes repeated loading and unloading cycles, these micro-cracks grow and coalesce to form larger cracks.
Over time, the structural integrity of the metal is compromised, leading to failure.
The process of fatigue damage can be divided into three stages: crack initiation, crack propagation, and final fracture.
Initial crack formation is often difficult to detect, as it occurs at the microscopic level.
However, as cracks grow, they can significantly reduce the load-bearing capacity of the metal, ultimately leading to a complete break.
Factors Influencing Fatigue Life
Several factors influence the fatigue life of metal materials.
These include the material’s properties, environmental conditions, and the nature of the applied cyclic loads.
1. Material Properties: The chemical composition and microstructure of a metal significantly affect its fatigue life.
Stronger materials with high tensile strength and toughness tend to have longer fatigue lives.
However, certain microstructural features, such as grain size and phase distribution, can also influence the material’s fatigue behavior.
2. Stress Concentration: Components with sharp corners, notches, or holes are more prone to fatigue damage, as they exhibit higher stress concentrations.
Designing components with smooth transitions and minimizing sharp features can help reduce the risk of fatigue failure.
3. Loading Conditions: The magnitude and frequency of the applied cyclic loads significantly affect the rate of fatigue damage accumulation.
Higher loads and frequencies typically lead to faster crack initiation and propagation.
4. Environmental Effects: Corrosive environments can accelerate fatigue damage by promoting crack growth.
The presence of moisture, chemicals, or extreme temperatures can exacerbate the metal’s susceptibility to fatigue.
Predicting Fatigue Life and Strength Using AI
Advancements in artificial intelligence (AI) and machine learning provide new opportunities for predicting fatigue life and strength in metal materials.
These technologies offer significant advantages in improving the accuracy and efficiency of fatigue assessments.
Application of Machine Learning Models
Machine learning models can be trained to recognize patterns in large datasets of material properties, loading conditions, and environmental factors.
These models can predict fatigue life and strength with high accuracy by analyzing complex relationships that are difficult to discern using traditional methods.
1. Data Collection: High-quality data is essential for training machine learning models.
Data may include information on material composition, stress-strain behavior, loading histories, and environmental conditions.
2. Model Training: Machine learning algorithms learn from the collected data to develop predictive models.
Common algorithms used for fatigue prediction include decision trees, random forests, support vector machines, and neural networks.
3. Validation and Testing: Once trained, the models are validated using separate datasets to ensure their accuracy and reliability in real-world applications.
Benefits of AI in Fatigue Analysis
The integration of AI in fatigue analysis offers numerous benefits, making it an invaluable tool for modern engineering applications.
1. Increased Accuracy: AI models can analyze vast amounts of data quickly and accurately, providing reliable predictions for fatigue life and strength.
This helps engineers make informed decisions in design and maintenance.
2. Cost Efficiency: By accurately predicting fatigue failure, AI can help minimize costly downtimes and extend the lifespan of metal components, leading to significant cost savings.
3. Continuous Improvement: AI models can adapt and improve over time as more data becomes available.
This continuous learning process allows for more accurate and precise predictions.
Challenges and Future Prospects
While AI and machine learning hold great promise for fatigue analysis, certain challenges remain to be addressed.
1. Data Quality: Obtaining high-quality, comprehensive datasets can be challenging, particularly for complex materials and loading conditions.
2. Model Interpretability: Understanding and explaining the decision-making process of AI models can be difficult, which may limit their acceptance in critical applications where transparency is essential.
3. Integration with Existing Systems: Seamlessly incorporating AI tools into current engineering processes may require significant effort and investment.
Despite these challenges, the future prospects for AI in fatigue analysis are promising.
As technology advances, AI models will continue to become more sophisticated and accurate.
This will lead to safer and more efficient designs, enhancing the durability and reliability of metal components across various industries.
In summary, understanding fatigue damage in metal materials is crucial for ensuring the safety and reliability of engineering structures.
With the application of AI and machine learning, engineers can predict fatigue life and strength more accurately and efficiently, ultimately extending the lifespan of metal components and reducing the risk of failure.
資料ダウンロード
QCD管理受発注クラウド「newji」は、受発注部門で必要なQCD管理全てを備えた、現場特化型兼クラウド型の今世紀最高の受発注管理システムとなります。
ユーザー登録
受発注業務の効率化だけでなく、システムを導入することで、コスト削減や製品・資材のステータス可視化のほか、属人化していた受発注情報の共有化による内部不正防止や統制にも役立ちます。
NEWJI DX
製造業に特化したデジタルトランスフォーメーション(DX)の実現を目指す請負開発型のコンサルティングサービスです。AI、iPaaS、および先端の技術を駆使して、製造プロセスの効率化、業務効率化、チームワーク強化、コスト削減、品質向上を実現します。このサービスは、製造業の課題を深く理解し、それに対する最適なデジタルソリューションを提供することで、企業が持続的な成長とイノベーションを達成できるようサポートします。
製造業ニュース解説
製造業、主に購買・調達部門にお勤めの方々に向けた情報を配信しております。
新任の方やベテランの方、管理職を対象とした幅広いコンテンツをご用意しております。
お問い合わせ
コストダウンが利益に直結する術だと理解していても、なかなか前に進めることができない状況。そんな時は、newjiのコストダウン自動化機能で大きく利益貢献しよう!
(β版非公開)