- お役立ち記事
- Fundamentals of image recognition technology and application and implementation of CNN and Vision Transformer
月間77,185名の
製造業ご担当者様が閲覧しています*
*2025年2月28日現在のGoogle Analyticsのデータより
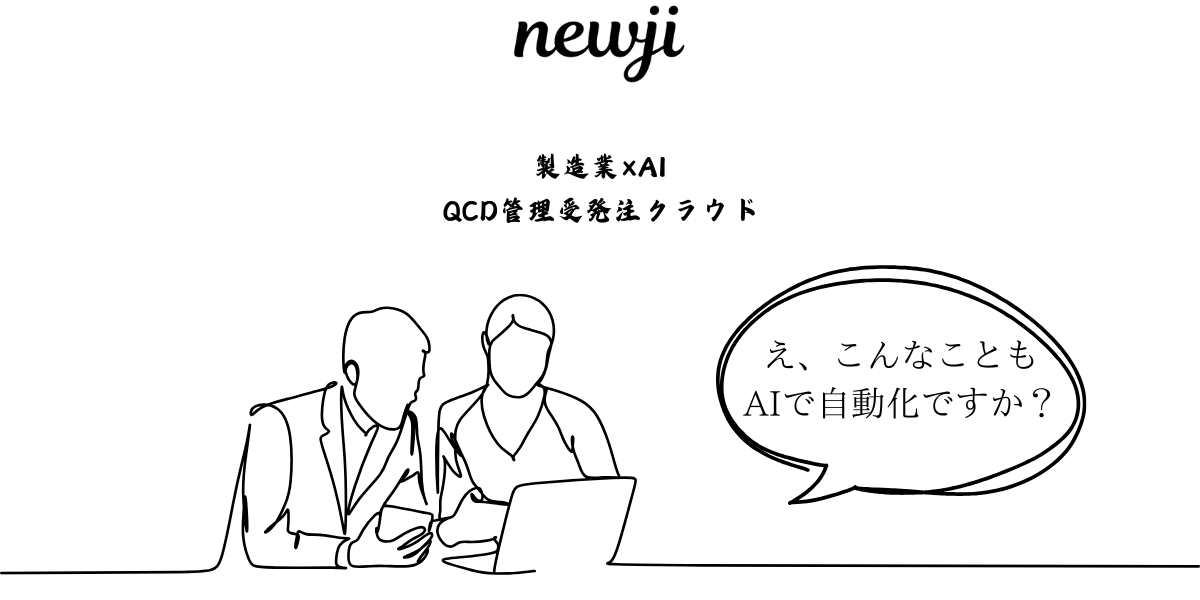
Fundamentals of image recognition technology and application and implementation of CNN and Vision Transformer
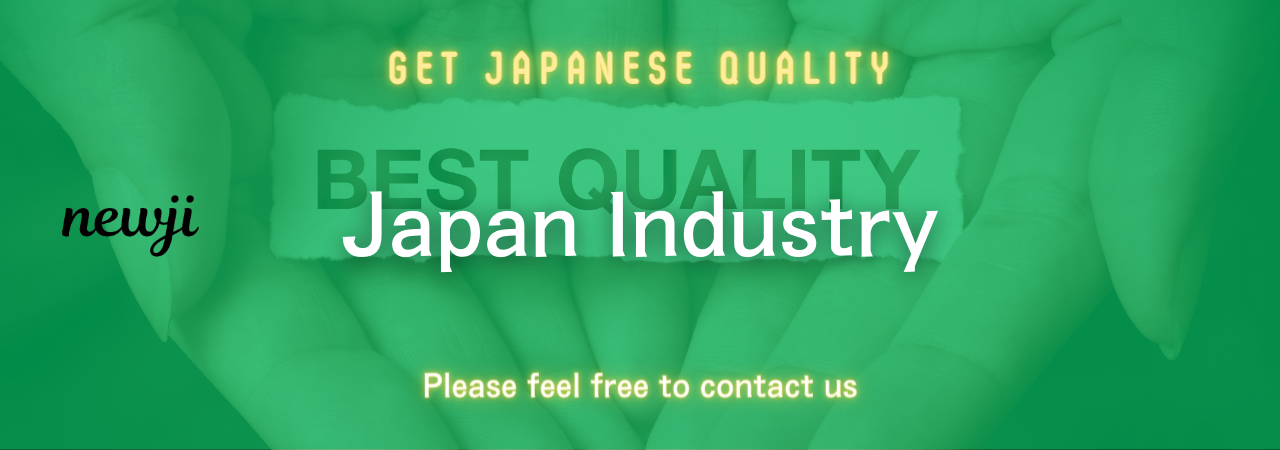
目次
Understanding Image Recognition Technology
Image recognition technology has rapidly developed over the past few years, becoming an integral part of various industries.
Its ability to analyze and interpret visual information has led to advancements in fields such as healthcare, automotive, and security.
At its core, image recognition technology involves the identification and categorization of objects within an image.
The Role of Machine Learning in Image Recognition
Machine learning plays a crucial role in the development of image recognition systems.
By training on vast datasets, these systems learn to recognize patterns and features within images.
This process involves deep learning, a subset of machine learning, which uses neural networks with multiple layers to perform analysis on data.
The Importance of CNN in Image Recognition
Convolutional Neural Networks (CNNs) have emerged as a powerful tool in image recognition.
They are designed specifically to take advantage of the spatial structure of images.
CNNs use a series of convolutional layers to detect features, making them particularly effective in handling complex image data.
How CNNs Work
CNNs start by applying convolutional layers to input images, which involves using filters to detect specific features like edges, corners, or patterns.
Each layer extracts different features, which are then combined through pooling and fully connected layers to generate predictions about the objects in the image.
The architecture of CNNs allows them to effectively recognize increasingly complex patterns as they progress through the layers.
Vision Transformer: A Modern Approach
The Vision Transformer (ViT) represents a modern approach to image recognition, diverging from the traditional CNNs.
It is based on the transformer architecture, originally developed for natural language processing tasks.
ViT operates by breaking down an image into patches and processing these patches independently to understand the image.
How Vision Transformer Works
ViT begins by dividing an image into fixed-size patches, effectively turning a 2D image into a sequence of linear embeddings.
These embeddings are then fed into a transformer encoder, which processes the entire sequence using its self-attention mechanism.
This method allows ViT to capture long-distance dependencies within the image, offering a new way to handle visual data.
Applications of Image Recognition Technology
The advancements in image recognition have led to its application in numerous fields.
In healthcare, it aids in diagnosing diseases by analyzing medical images like X-rays and MRIs.
For the automotive industry, image recognition is a key component in developing autonomous driving systems, enabling vehicles to detect road signs and pedestrians.
Security and Surveillance
In security and surveillance, image recognition technology is used to identify and track individuals through facial recognition systems.
It can detect suspicious activities in real-time, enhancing security measures in public spaces.
Consumer Electronics and Retail
Image recognition also finds significant use in consumer electronics and retail.
For example, it powers features like face unlock in smartphones and assists in inventory management through visual recognition of products in stores.
Implementing CNN and Vision Transformer in Your Projects
To implement CNN and Vision Transformer in image recognition projects, one must start with selecting the right framework and dataset.
Popular frameworks include TensorFlow and PyTorch, both offering robust libraries for building and training image recognition models.
Training a CNN Model
When training a CNN model, it’s crucial to have access to a labelled dataset that represents the kinds of images you expect the model to analyze.
The training process involves iterating through the dataset, allowing the CNN to learn and adjust its parameters based on the features it must detect.
Utilizing Vision Transformer
Implementing Vision Transformer involves similar initial steps as CNN, but with a different architecture set-up.
Using the transformer framework requires understanding how to break images into patches and set the appropriate parameters for the attention mechanism.
ViT models can provide high accuracy, especially when handling diverse image datasets.
Final Thoughts on Image Recognition Technology
Image recognition technology continues to evolve, with CNN and Vision Transformer leading the way in innovation.
Understanding the fundamentals of these technologies is vital for anyone looking to implement image recognition systems in real-world applications.
As these technologies advance, we can expect even greater enhancements in accuracy and efficiency, further broadening their application across various sectors.
資料ダウンロード
QCD管理受発注クラウド「newji」は、受発注部門で必要なQCD管理全てを備えた、現場特化型兼クラウド型の今世紀最高の受発注管理システムとなります。
ユーザー登録
受発注業務の効率化だけでなく、システムを導入することで、コスト削減や製品・資材のステータス可視化のほか、属人化していた受発注情報の共有化による内部不正防止や統制にも役立ちます。
NEWJI DX
製造業に特化したデジタルトランスフォーメーション(DX)の実現を目指す請負開発型のコンサルティングサービスです。AI、iPaaS、および先端の技術を駆使して、製造プロセスの効率化、業務効率化、チームワーク強化、コスト削減、品質向上を実現します。このサービスは、製造業の課題を深く理解し、それに対する最適なデジタルソリューションを提供することで、企業が持続的な成長とイノベーションを達成できるようサポートします。
製造業ニュース解説
製造業、主に購買・調達部門にお勤めの方々に向けた情報を配信しております。
新任の方やベテランの方、管理職を対象とした幅広いコンテンツをご用意しております。
お問い合わせ
コストダウンが利益に直結する術だと理解していても、なかなか前に進めることができない状況。そんな時は、newjiのコストダウン自動化機能で大きく利益貢献しよう!
(β版非公開)