- お役立ち記事
- Fundamentals of image recognition technology, machine learning, applications of deep learning and their key points
月間77,185名の
製造業ご担当者様が閲覧しています*
*2025年2月28日現在のGoogle Analyticsのデータより
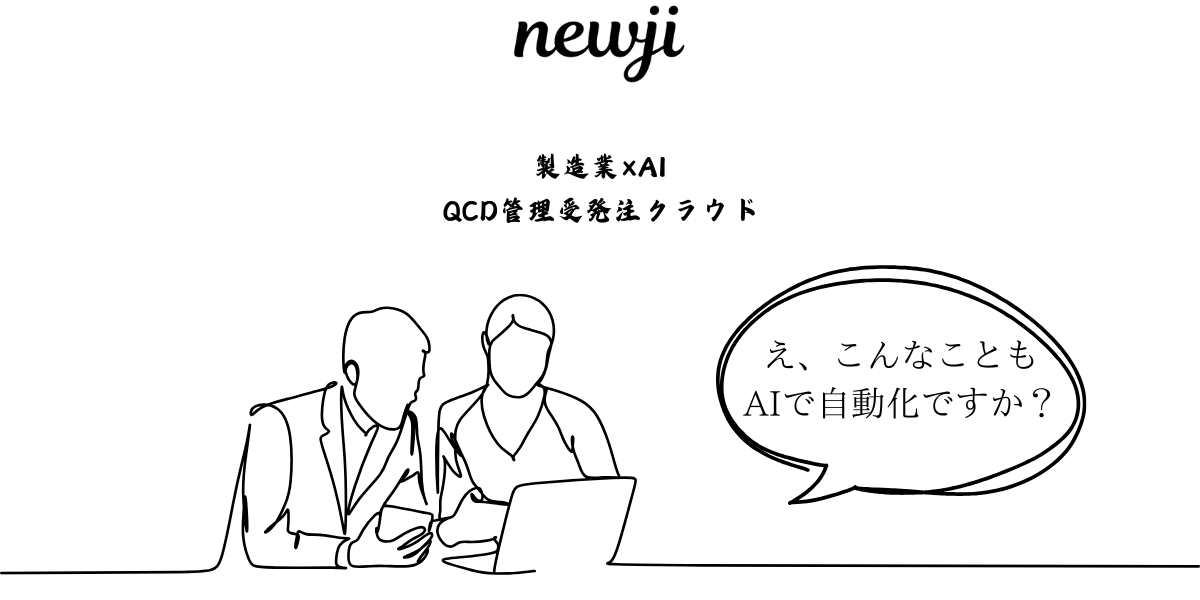
Fundamentals of image recognition technology, machine learning, applications of deep learning and their key points
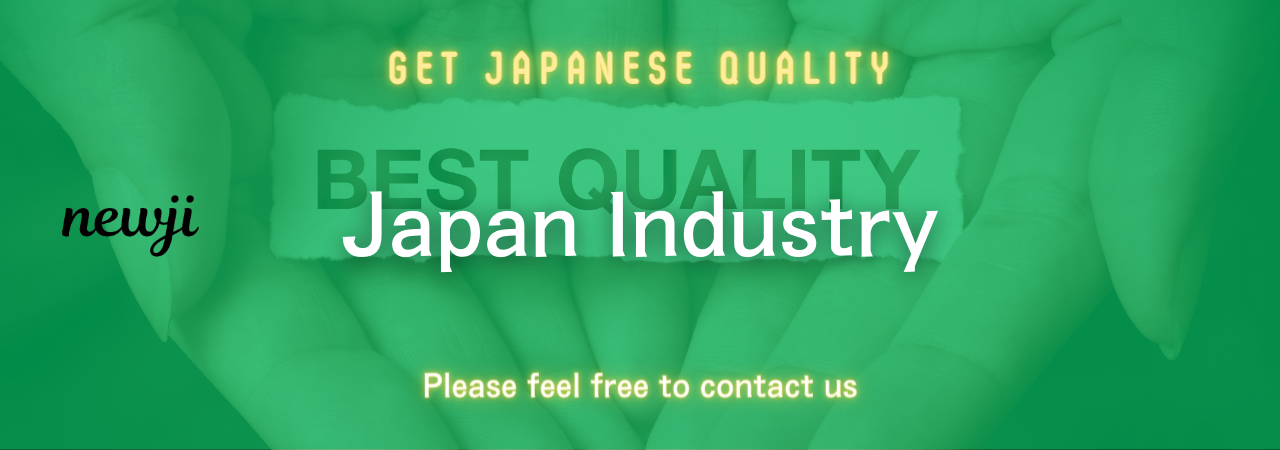
目次
Understanding Image Recognition Technology
Image recognition technology is an integral component of modern AI systems, enabling machines to interpret and understand visual data in the form of digital images or video frames.
At its core, image recognition allows for the identification and classification of objects, patterns, and features within an image.
The backbone of image recognition technology relies on sophisticated algorithms and models that discern and categorize different visual elements.
Historically, this field was limited by the computational power available, but with recent advancements, it has seen unprecedented progress.
Today, image recognition is not only more accurate but also much faster, thanks to modern innovations.
Evolution of Image Recognition
The evolution of image recognition technology has been significant over the past few decades.
Initially, image processing involved basic techniques like edge detection and pixel differentiation.
These methods were limited in scope and struggled with complex images.
As computational power increased, more elaborate models like Convolutional Neural Networks (CNNs) were developed.
CNNs, in particular, revolutionized the field with their ability to process data in a hierarchical manner, allowing deeper insights into image properties and facilitating more accurate recognition and classification.
The Role of Machine Learning in Image Recognition
Machine learning techniques are at the forefront of image recognition, enabling systems to improve their accuracy and efficiency over time by learning from data.
Supervised Learning
In image recognition, supervised learning involves training a model using labeled datasets.
For example, a dataset may contain images of cats and dogs labeled accordingly.
Through exposure to this data, the model learns to differentiate between the two, gaining the ability to recognize new, unlabeled images correctly.
Supervised learning is highly effective when there is plenty of labeled data available.
However, acquiring and annotating such data can be time-consuming and costly.
Unsupervised Learning
Unsupervised learning, in contrast, utilizes unlabeled data to train models.
The system identifies patterns and structures in the data without explicit instructions.
In image recognition, it can be used to cluster similar images or segments within an image.
This approach is beneficial when labeled data is scarce or nonexistent, allowing machines to discover underlying patterns independently.
Deep Learning: A Game-Changer in Image Recognition
Deep learning, a subset of machine learning, has brought unprecedented enhancements to image recognition technology.
Its ability to learn from vast amounts of data and process information in multi-layered networks has set a new standard in accuracy and performance.
Convolutional Neural Networks (CNNs)
CNNs are specifically designed to process pixel data and are widely used in image recognition for their ability to capture spatial hierarchies in images.
They utilize layers of convolutions and pooling to reduce image dimensions while maintaining critical features.
This process enables CNNs to focus on parts of an image that are most indicative of the object being recognized, making them highly efficient and effective.
Transfer Learning
Transfer learning involves taking a pre-trained model and applying it to a new but related problem.
For example, a model trained to identify various dog breeds can be adjusted to recognize cat breeds with minimal additional training.
This technique is particularly valuable for image recognition tasks where training data is limited, as it allows the reuse of models without starting from scratch.
Applications of Image Recognition Technology
The applications of image recognition technology are vast and diverse, impacting various sectors from healthcare to retail, and even autonomous vehicles.
Healthcare
In healthcare, image recognition technology plays a vital role in diagnostics.
It is used to analyze medical images such as X-rays, CT scans, and MRIs to detect and diagnose conditions like tumors, fractures, and other anomalies.
By providing timely and accurate analysis, this technology assists healthcare professionals in making informed decisions, ultimately improving patient outcomes.
Retail and Marketing
Retail and marketing sectors leverage image recognition to enhance customer experience and operational efficiency.
In retail, it is used for inventory management and checkout processes through visual recognition systems.
For marketing, companies use it to analyze consumer behavior and preferences by examining image data from social media and advertisements.
Autonomous Vehicles
Autonomous vehicles rely heavily on image recognition technology to navigate and understand their surroundings.
By continuously scanning the environment, it allows vehicles to identify other cars, pedestrians, signals, and road signs.
This capability is crucial for the safe and efficient operation of self-driving cars.
Key Points to Consider in Image Recognition
While image recognition technology offers numerous benefits, there are key challenges and considerations to be aware of.
Data Diversity and Quality
For image recognition systems to perform effectively, they require access to diverse and high-quality datasets.
The more varied the data, the better the model can generalize and perform across different scenarios.
Ensuring quality involves careful curation and annotation of training datasets to minimize biases and errors.
Computational Requirements
Image recognition technologies, especially deep learning models, demand significant computational resources for training and inference.
This can be a limitation for some applications where resources are scarce.
It necessitates a balance between performance and resource constraints, often leading to the use of cloud-based solutions.
Ethical and Privacy Concerns
The use of image recognition technology raises ethical and privacy concerns around data collection and usage.
Systems must ensure compliance with data protection regulations and respect user privacy.
Transparency in how data is used and implementing robust security measures are crucial to building trust and acceptance of this technology.
By understanding the fundamentals of image recognition technology, machine learning, and deep learning, along with their applications and key considerations, we better appreciate how these technologies are shaping our world.
With continuous advancements, the potential for image recognition to transform industries and improve lives is immense.
資料ダウンロード
QCD管理受発注クラウド「newji」は、受発注部門で必要なQCD管理全てを備えた、現場特化型兼クラウド型の今世紀最高の受発注管理システムとなります。
ユーザー登録
受発注業務の効率化だけでなく、システムを導入することで、コスト削減や製品・資材のステータス可視化のほか、属人化していた受発注情報の共有化による内部不正防止や統制にも役立ちます。
NEWJI DX
製造業に特化したデジタルトランスフォーメーション(DX)の実現を目指す請負開発型のコンサルティングサービスです。AI、iPaaS、および先端の技術を駆使して、製造プロセスの効率化、業務効率化、チームワーク強化、コスト削減、品質向上を実現します。このサービスは、製造業の課題を深く理解し、それに対する最適なデジタルソリューションを提供することで、企業が持続的な成長とイノベーションを達成できるようサポートします。
製造業ニュース解説
製造業、主に購買・調達部門にお勤めの方々に向けた情報を配信しております。
新任の方やベテランの方、管理職を対象とした幅広いコンテンツをご用意しております。
お問い合わせ
コストダウンが利益に直結する術だと理解していても、なかなか前に進めることができない状況。そんな時は、newjiのコストダウン自動化機能で大きく利益貢献しよう!
(β版非公開)