- お役立ち記事
- Fundamentals of image sensing technology, image recognition technology, and deep learning and their application to autonomous driving
Fundamentals of image sensing technology, image recognition technology, and deep learning and their application to autonomous driving
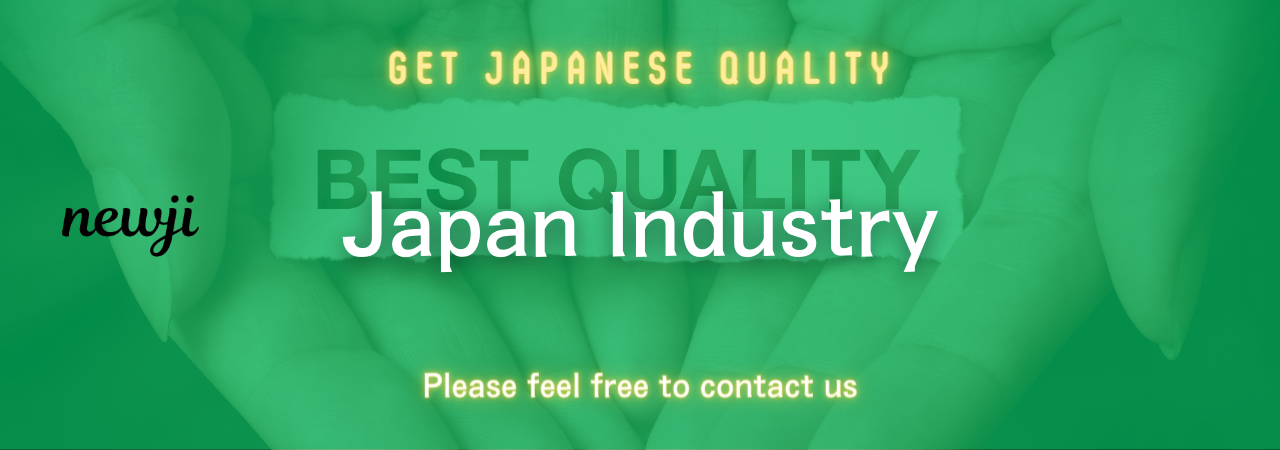
目次
Introduction to Image Sensing Technology
Image sensing technology has significantly evolved over the years, becoming an integral part of many modern applications, including autonomous driving.
At its core, image sensing involves capturing visual data from the environment using cameras or other sensors.
These sensors convert the captured light into electronic signals, creating digital images that can be processed and analyzed.
Image sensors are designed to emulate the human eye, with various configurations and methods to capture light efficiently.
From simple cameras in consumer devices to advanced sensors in robotics and vehicles, image sensing is the starting point for any visual processing task.
Types of Image Sensors
Two common types of image sensors are CCD (Charge-Coupled Device) and CMOS (Complementary Metal-Oxide-Semiconductor) sensors.
CCD sensors are known for their high-quality images and sensitivity to light, making them ideal for scientific and professional photography.
However, they tend to be more expensive and consume more power.
CMOS sensors, on the other hand, are widely used in consumer electronics due to their lower cost, reduced power consumption, and faster readout speeds.
This makes CMOS sensors especially suitable for applications like smartphones, security cameras, and autonomous vehicles, where cost and energy efficiency are crucial.
Understanding Image Recognition Technology
Image recognition technology transforms captured images into information that machines can understand.
This involves identifying objects, patterns, faces, and scenes within digital images.
The technology relies on machine learning algorithms that are trained on millions of images to recognize various elements within a picture.
Applications of Image Recognition
Image recognition has found applications in numerous fields.
For example, in healthcare, it is used for diagnosing diseases from medical scans.
In retail, image recognition powers virtual try-on tools for clothing and accessories.
Importantly, in the realm of autonomous driving, this technology helps vehicles understand their surroundings by identifying objects such as pedestrians, other vehicles, road signs, and obstacles.
Image Recognition Techniques
Convolutional Neural Networks (CNNs) are the backbone of modern image recognition techniques.
CNNs mimic the human visual cortex and consist of layers that automatically and adaptively learn spatial hierarchies of features, from edges to complex objects.
Transfer learning, a subfield of image recognition, involves leveraging pre-trained models on large datasets to enhance the recognition accuracy of new models with limited data.
This approach is particularly effective in saving time and computational resources.
Deep Learning: A Revolution in Image Processing
Deep learning has transformed how image processing tasks are performed.
It involves neural networks with many layers (hence “deep”) that learn representations of data with multiple levels of abstraction.
The Role of Deep Learning in Image Processing
Deep learning enables unprecedented accuracy in image processing tasks.
For instance, deep networks can automatically classify and label complex scenes in an image.
This capability is advantageous in scenarios like autonomous driving, where real-time decision-making based on accurate scene understanding is crucial.
Deep learning models, such as Deep Convolutional Networks (DCNs) and Generative Adversarial Networks (GANs), have been employed to enhance image quality, perform super-resolution tasks, and generate realistic images.
Challenges in Deep Learning
While deep learning offers remarkable possibilities, it also presents challenges.
Training deep networks requires substantial data and computational power.
Moreover, these models can be sensitive to changes in input data, suffering from issues like adversarial attacks where small, imperceptible changes can lead to incorrect predictions.
Thus, developing robust algorithms that can handle variations in real-world data is an ongoing research focus.
Application to Autonomous Driving
Autonomous driving systems combine image sensing, recognition, and deep learning to enable self-driving capabilities.
How These Technologies Work Together
In autonomous vehicles, image sensors capture real-time visual data from the vehicle’s surroundings.
This data is fed to image recognition systems that identify and categorize different objects and scenarios on the road.
Deep learning algorithms further process this information, helping the vehicle make informed driving decisions, such as navigating through traffic, recognizing road signs, and avoiding obstacles.
Benefits of Autonomous Driving
Autonomous vehicles promise increased safety by reducing human errors, which are the leading cause of traffic accidents.
They offer convenience, allowing passengers to engage in other activities while commuting.
Moreover, autonomous driving can optimize traffic flow, reduce congestion, and improve fuel efficiency, contributing to environmental sustainability.
Challenges in Implementing Autonomous Driving
Despite its potential, autonomous driving presents challenges.
Safety remains a primary concern, as systems must be foolproof, and handle diverse driving conditions.
Regulatory hurdles, public acceptance, and ethical issues regarding decision-making in critical situations are also significant barriers.
Additionally, ensuring data security and privacy is crucial, preventing unauthorized access and tampering with vehicle systems.
Conclusion
Image sensing, recognition, and deep learning collectively form the backbone of modern autonomous driving systems.
These technologies not only improve how vehicles perceive and interact with their environments but also enhance safety and efficiency.
The future of autonomous driving and related applications is burgeoning with promise, yet necessitates ongoing innovation and oversight to address technical, regulatory, and ethical challenges.
As technology continues to advance, we move closer to realizing the full potential of self-driving vehicles, transforming the way we travel and interact with the world around us.
資料ダウンロード
QCD調達購買管理クラウド「newji」は、調達購買部門で必要なQCD管理全てを備えた、現場特化型兼クラウド型の今世紀最高の購買管理システムとなります。
ユーザー登録
調達購買業務の効率化だけでなく、システムを導入することで、コスト削減や製品・資材のステータス可視化のほか、属人化していた購買情報の共有化による内部不正防止や統制にも役立ちます。
NEWJI DX
製造業に特化したデジタルトランスフォーメーション(DX)の実現を目指す請負開発型のコンサルティングサービスです。AI、iPaaS、および先端の技術を駆使して、製造プロセスの効率化、業務効率化、チームワーク強化、コスト削減、品質向上を実現します。このサービスは、製造業の課題を深く理解し、それに対する最適なデジタルソリューションを提供することで、企業が持続的な成長とイノベーションを達成できるようサポートします。
オンライン講座
製造業、主に購買・調達部門にお勤めの方々に向けた情報を配信しております。
新任の方やベテランの方、管理職を対象とした幅広いコンテンツをご用意しております。
お問い合わせ
コストダウンが利益に直結する術だと理解していても、なかなか前に進めることができない状況。そんな時は、newjiのコストダウン自動化機能で大きく利益貢献しよう!
(Β版非公開)