- お役立ち記事
- Fundamentals of LiDAR point cloud processing and sensing applications using deep learning
月間76,176名の
製造業ご担当者様が閲覧しています*
*2025年3月31日現在のGoogle Analyticsのデータより
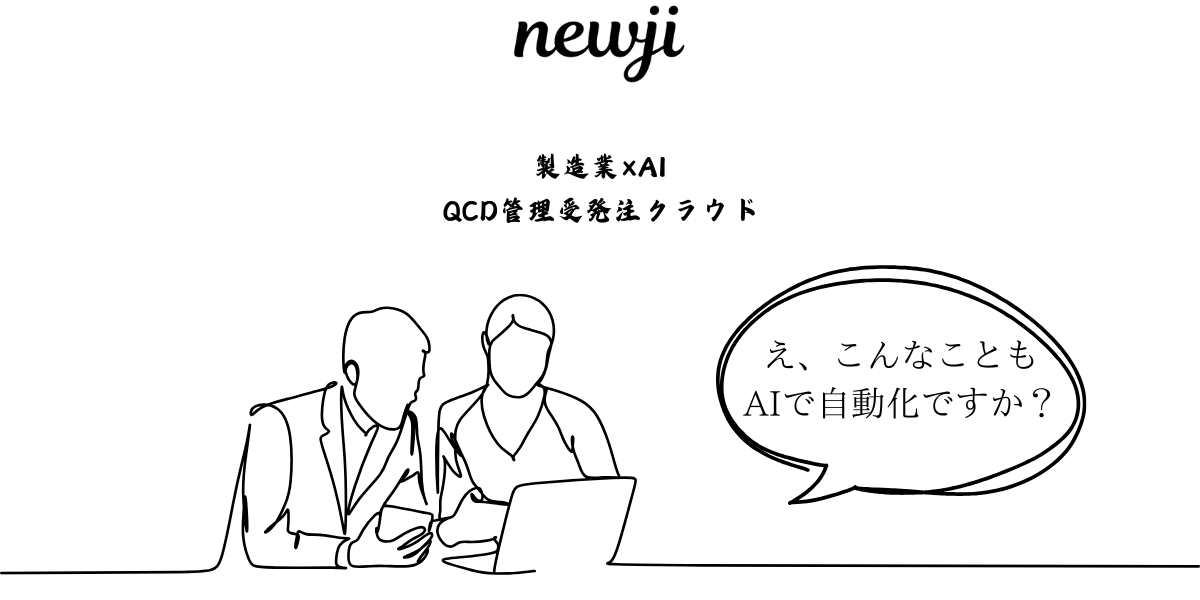
Fundamentals of LiDAR point cloud processing and sensing applications using deep learning
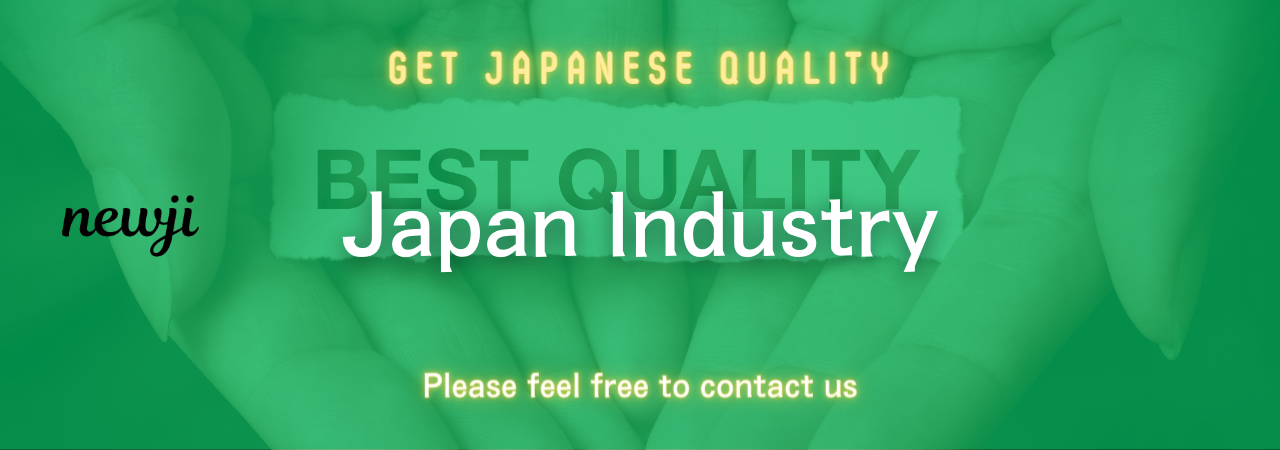
目次
Understanding LiDAR Technology
Light Detection and Ranging, commonly known as LiDAR, is a remote sensing method that uses laser light to measure distances between objects.
This technology creates precise, three-dimensional information about the shape and surface characteristics of the Earth.
Widely used in various industries, LiDAR is crucial for applications such as topographic mapping, forestry management, urban planning, and, more recently, autonomous vehicles.
LiDAR systems work by emitting laser pulses at an object and measuring the time it takes for the pulses to return.
These measurements are used to determine the distance between the sensor (on ground or aerial) and the object.
Equipped with high precision and rapid acquisition rates, these systems can capture a myriad of data points in a short period.
These data points are then compiled to form a comprehensive “point cloud”.
What are LiDAR Point Clouds?
A LiDAR point cloud is a collection of data points representing the spatial characteristics of surfaces and objects within the Laser’s range.
Each point is mapped with X, Y, and Z coordinates, denoting its position in three-dimensional space.
The dense formation of these points provides detailed and accurate representations of geographic and man-made environments.
Point clouds are invaluable in various sectors as they provide a realistic model of the scanned environment.
They are instrumental for 3D modeling, object detection, and pattern recognition.
For instance, in environmental monitoring, they help in tracking changes in terrain over time.
In autonomous vehicles, point clouds assist in real-time navigation and obstacle avoidance.
Deep Learning in LiDAR Point Cloud Processing
Deep learning, a subset of artificial intelligence, has made notable strides in processing LiDAR point clouds.
Traditionally, point cloud processing involved manual extraction and classification, a task that was labor-intensive and prone to human error.
However, deep learning algorithms, particularly convolutional neural networks (CNNs), have simplified and accelerated this process.
Deep learning models are trained on vast datasets to recognize patterns and categorize objects within point clouds.
For instance, they can classify different segments of a scanned area as buildings, roads, vegetation, or water bodies.
This automation reduces errors, enhances efficiency, and makes processing large datasets more feasible.
Applications of Deep Learning in LiDAR Processing
1. **Autonomous Vehicles**:
Deep learning algorithms facilitate real-time decision-making by classifying objects and predicting their movements.
This processing aids in obstacle detection, road sign recognition, and traffic management, enhancing safety features in autonomous driving systems.
2. **Smart Cities and Urban Planning**:
Accurate 3D models derived from LiDAR point clouds help urban planners in designing infrastructure and analyzing spatial constraints.
Deep learning assists in managing and interpreting extensive datasets, allowing for more effective planning and monitoring of urban expansion.
3. **Environmental Monitoring and Disaster Management**:
With deep learning, LiDAR data is used to analyze changes in landscapes, such as deforestation patterns or flood impact assessment.
This capability helps governments and organizations in formulating strategies for environmental conservation and disaster response planning.
4. **Forestry Management**:
In forestry, LiDAR helps map forest structures, calculate biomass, and assess vegetation health.
Deep learning enhances data classification, helping in precise forest inventory and sustainable forestry practices.
Challenges and Future of LiDAR Point Cloud Processing
While LiDAR and deep learning together offer significant advantages, certain challenges remain.
One of the primary issues is the volume and complexity of data generated, requiring robust algorithms capable of handling such datasets efficiently.
Moreover, ensuring the accuracy of data interpretation and minimizing potential errors poses another layer of challenge.
Advancements in computational power and the development of more sophisticated algorithms continue to mitigate these challenges.
Many researchers are focusing on improving the speed and precision of deep learning models, enabling real-time processing of LiDAR data.
As technology evolves, the integration of LiDAR with other sensory data (like cameras and radar) can lead to more comprehensive environmental modeling.
Such advancements will open new frontiers in applications not only in automotive industries but also in fields like archeology, agriculture, and public safety.
Conclusion
LiDAR technology, combined with the power of deep learning, is propelling forward our ability to understand and interact with the world.
It streamlines processes across industries, offering efficient solutions in data acquisition, modeling, and analysis.
Though challenges exist, the continued refinement of deep learning methods points towards a future of even greater potential and innovation.
As LiDAR becomes more accessible, its applications will undoubtedly expand, impacting new areas and paving the way for groundbreaking developments in how we perceive and manage our environments.
資料ダウンロード
QCD管理受発注クラウド「newji」は、受発注部門で必要なQCD管理全てを備えた、現場特化型兼クラウド型の今世紀最高の受発注管理システムとなります。
ユーザー登録
受発注業務の効率化だけでなく、システムを導入することで、コスト削減や製品・資材のステータス可視化のほか、属人化していた受発注情報の共有化による内部不正防止や統制にも役立ちます。
NEWJI DX
製造業に特化したデジタルトランスフォーメーション(DX)の実現を目指す請負開発型のコンサルティングサービスです。AI、iPaaS、および先端の技術を駆使して、製造プロセスの効率化、業務効率化、チームワーク強化、コスト削減、品質向上を実現します。このサービスは、製造業の課題を深く理解し、それに対する最適なデジタルソリューションを提供することで、企業が持続的な成長とイノベーションを達成できるようサポートします。
製造業ニュース解説
製造業、主に購買・調達部門にお勤めの方々に向けた情報を配信しております。
新任の方やベテランの方、管理職を対象とした幅広いコンテンツをご用意しております。
お問い合わせ
コストダウンが利益に直結する術だと理解していても、なかなか前に進めることができない状況。そんな時は、newjiのコストダウン自動化機能で大きく利益貢献しよう!
(β版非公開)