- お役立ち記事
- Fundamentals of material mechanics and fatigue strength, fracture surface analysis, and fracture surface image recognition using machine learning and its applications
月間76,176名の
製造業ご担当者様が閲覧しています*
*2025年3月31日現在のGoogle Analyticsのデータより
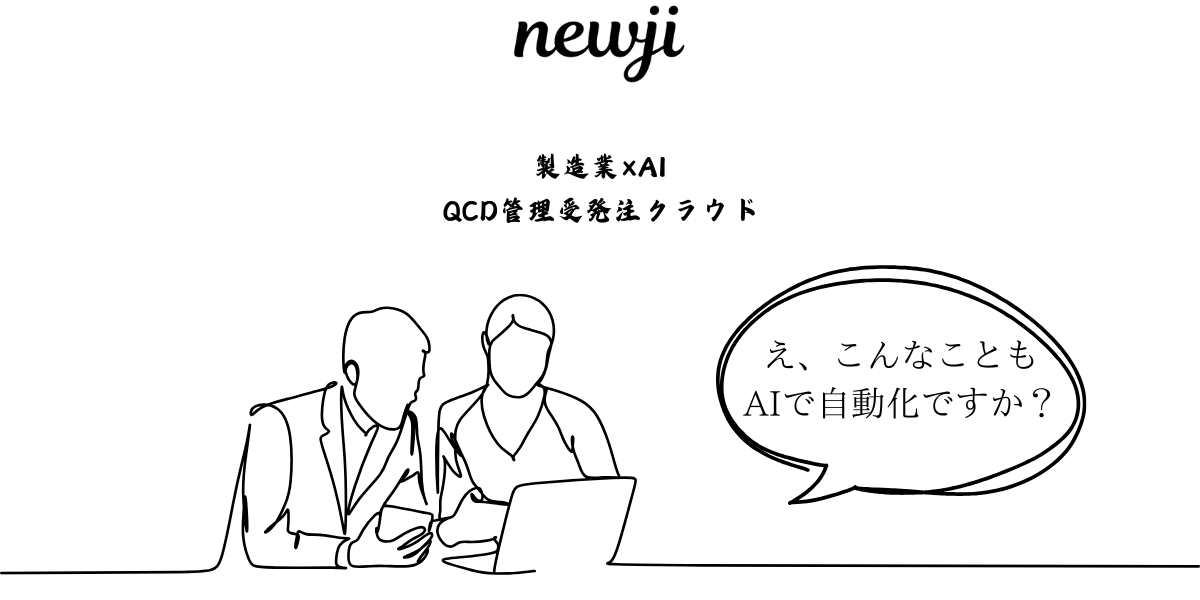
Fundamentals of material mechanics and fatigue strength, fracture surface analysis, and fracture surface image recognition using machine learning and its applications
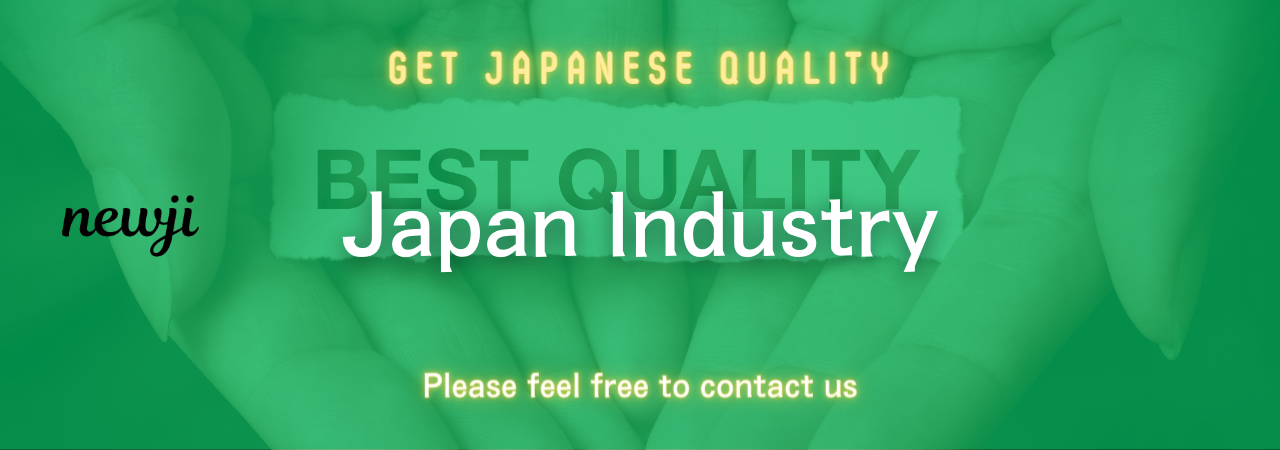
目次
Understanding Material Mechanics
Material mechanics is a branch of engineering that explores the behavior of materials under different forces and conditions.
It is essential to understand the fundamental concepts of material mechanics to design and analyze structures effectively.
Material mechanics involves studying how materials respond to stress, strain, and other external loads.
This knowledge helps engineers predict how materials will react when subjected to various forces.
Material mechanics encompasses several key concepts, including elasticity, plasticity, and toughness.
Elasticity refers to a material’s ability to return to its original shape after being deformed.
Plasticity, on the other hand, is the measure of a material’s capacity to undergo permanent deformation without breaking.
Toughness indicates a material’s ability to absorb energy and resist fracturing.
Fatigue Strength in Materials
Fatigue strength is a critical factor in evaluating the longevity and durability of materials.
It describes a material’s ability to withstand cyclic loading without experiencing failure over time.
In real-world applications, materials often face repetitive stress that can lead to fatigue failure if not properly understood or mitigated.
Fatigue failure occurs when a material is subjected to repeated cyclic loads, even if these loads are below the material’s ultimate tensile strength.
This type of failure is particularly dangerous because it can happen suddenly and without noticeable deformation.
Engineers must consider fatigue strength in the design and assessment of components in structures like bridges, airplanes, and machinery to ensure safety and reliability.
Fracture Surface Analysis
Fracture surface analysis is an essential technique for understanding material failures.
By examining the surface of a fracture, engineers can identify the cause of failure and preventive measures.
The appearance of a fracture surface can reveal valuable information about the conditions that led to the failure.
There are various features on a fracture surface, such as beach marks, striations, and crack initiation points, which can be studied in detail.
These features help determine the type of loading, the environmental conditions, and other factors that contributed to the material’s failure.
Fracture surface analysis is a crucial tool in failure analysis, allowing engineers to improve material design and prevent future failures.
Machine Learning in Fracture Surface Image Recognition
Advancements in technology have introduced machine learning as a powerful tool for fracture surface image recognition.
Machine learning algorithms can be trained to identify and classify the features of fracture surfaces automatically.
This application greatly enhances the speed and accuracy of fracture surface analysis.
With machine learning, large datasets of fracture surfaces can be analyzed quickly, enabling engineers to identify patterns and correlations that might not be apparent through manual inspection.
Machine learning models can be used to automate the classification of fracture types, predict potential failure points, and even suggest improvements in material design.
Applications of Machine Learning in Material Mechanics
Machine learning has various applications in material mechanics beyond fracture surface analysis.
It can be used to predict material behavior under different conditions and optimize material composition for specific applications.
By leveraging machine learning, engineers can develop more efficient and safer materials and structures.
One application is in the prediction of fatigue life.
Machine learning models can analyze historical data to predict the fatigue life of materials with greater accuracy.
This helps in the design process by identifying the most durable materials for a given application.
Moreover, machine learning can enhance the testing and development processes by reducing the need for extensive physical testing.
Predictive models can simulate material behavior under various scenarios, saving time and resources while innovating new materials more rapidly.
The Future of Material Mechanics with Machine Learning
The integration of machine learning in material mechanics represents a promising direction for the future of engineering.
With ongoing advancements, machine learning algorithms will become even more sophisticated, allowing for deeper insights into material behavior and failure mechanisms.
As data collection and processing capabilities continue to improve, the accuracy and applicability of machine learning models will expand.
This development will enable engineers to tackle complex challenges in material mechanics with greater efficiency.
In conclusion, understanding the fundamentals of material mechanics, including fatigue strength and fracture surface analysis, is essential for designing and analyzing reliable materials and structures.
By applying machine learning to these areas, engineers can enhance their ability to predict material failure and innovate more robust and efficient materials and designs for future applications.
資料ダウンロード
QCD管理受発注クラウド「newji」は、受発注部門で必要なQCD管理全てを備えた、現場特化型兼クラウド型の今世紀最高の受発注管理システムとなります。
ユーザー登録
受発注業務の効率化だけでなく、システムを導入することで、コスト削減や製品・資材のステータス可視化のほか、属人化していた受発注情報の共有化による内部不正防止や統制にも役立ちます。
NEWJI DX
製造業に特化したデジタルトランスフォーメーション(DX)の実現を目指す請負開発型のコンサルティングサービスです。AI、iPaaS、および先端の技術を駆使して、製造プロセスの効率化、業務効率化、チームワーク強化、コスト削減、品質向上を実現します。このサービスは、製造業の課題を深く理解し、それに対する最適なデジタルソリューションを提供することで、企業が持続的な成長とイノベーションを達成できるようサポートします。
製造業ニュース解説
製造業、主に購買・調達部門にお勤めの方々に向けた情報を配信しております。
新任の方やベテランの方、管理職を対象とした幅広いコンテンツをご用意しております。
お問い合わせ
コストダウンが利益に直結する術だと理解していても、なかなか前に進めることができない状況。そんな時は、newjiのコストダウン自動化機能で大きく利益貢献しよう!
(β版非公開)