- お役立ち記事
- Fundamentals of materials informatics and techniques for utilizing neural networks and quantum annealing
Fundamentals of materials informatics and techniques for utilizing neural networks and quantum annealing
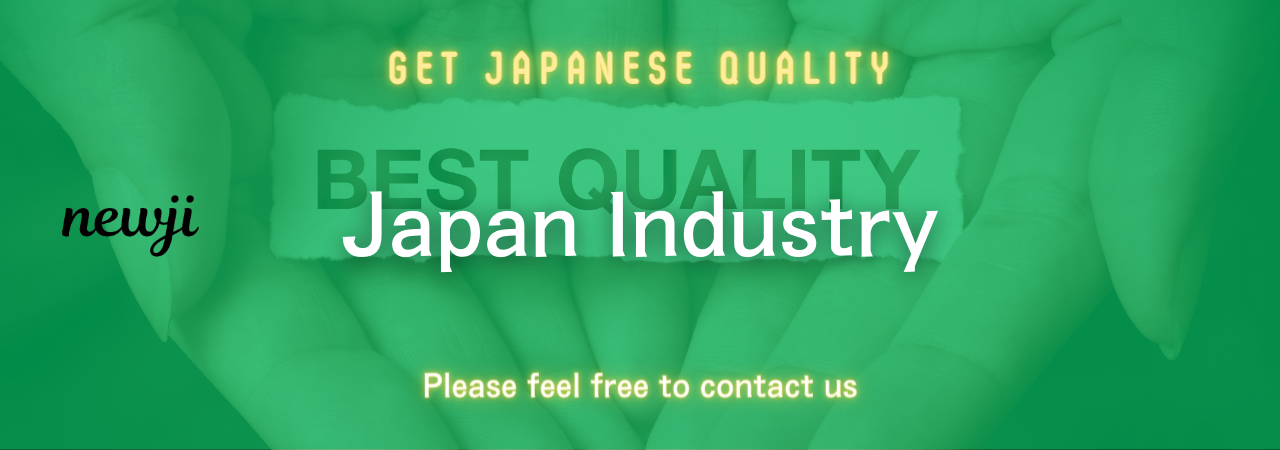
目次
Introduction to Materials Informatics
Materials informatics is an interdisciplinary field combining materials science, computer science, and data analytics to discover and design new materials.
It leverages advanced data processing, predictive modeling, and simulation techniques to accelerate material research and development.
The ultimate goal is to streamline the process of materials discovery and enhance existing materials for various applications.
In recent years, machine learning and artificial intelligence have significantly influenced materials informatics, enabling researchers to manage and interpret vast datasets.
Among these, neural networks and quantum annealing have garnered attention for their potential to revolutionize materials discovery.
This article will delve into the basics of these technologies and explore their applications in materials informatics.
Understanding Neural Networks
Neural networks are a subset of machine learning methods inspired by the human brain’s structure and functionality.
They consist of layers of interconnected nodes, or artificial neurons, which process information by simulating the way human neurons do.
These networks are particularly effective at identifying patterns and making predictions based on complex datasets.
In materials informatics, neural networks can be used to predict material properties, optimize material compositions, and identify novel materials with desired characteristics.
By training neural networks on existing materials data, researchers can create models that predict how new combinations of elements might behave, potentially uncovering materials with improved or entirely new properties.
Applications of Neural Networks in Materials Discovery
One of the primary uses of neural networks in materials discovery is for predicting the properties of yet-to-be-synthesized materials.
Researchers input data about known materials, such as their structures, compositions, and properties, to train the networks.
Once trained, these networks can predict the properties of new materials, significantly reducing the need for exhaustive experimental trials.
Additionally, neural networks are effective in optimizing the design of materials.
They assist in fine-tuning the composition and processing methods to achieve desired properties more efficiently.
Neural networks can also identify trends and anomalies in material data, providing insights that might not be apparent through traditional analysis.
Exploring Quantum Annealing
Quantum annealing is a specialized quantum computing technique designed to solve complex optimization problems more efficiently than classical computers.
It leverages quantum mechanics principles to explore multiple solutions simultaneously, which is particularly beneficial for navigating complex solution landscapes in materials science.
In materials informatics, quantum annealing can be used to optimize material structures and processes by finding the lowest energy configurations or optimal pathways.
This capability makes it an attractive tool for tackling problems with many possible solutions, such as determining the ideal atomic arrangement in a solid or optimizing a material’s properties under given constraints.
Practical Applications of Quantum Annealing
A significant application of quantum annealing in materials informatics is the optimization of material synthesis processes.
For example, researchers can use quantum annealing to determine the best sequence of processing steps or conditions to achieve a material with specific properties.
This process reduces the trial-and-error typically associated with experimental approaches, saving time and resources.
Another application is in the realm of drug discovery, where quantum annealing can assist in identifying candidate molecules or materials with the potential for targeted therapeutic effects.
The ability to navigate vast chemical spaces efficiently can accelerate the identification of promising compounds, facilitating faster development cycles.
Integrating Neural Networks and Quantum Annealing
While neural networks and quantum annealing can individually advance materials informatics, their integration presents even greater potential.
By combining the strengths of both technologies, researchers can enhance the predictive power and efficiency of material discovery processes.
For example, neural networks can be used to preprocess large datasets, highlighting promising material candidates or compositions.
These results can then be fed into a quantum annealer to optimize the structures further or predict processes with minimal experimental intervention.
This hybrid approach allows researchers to tackle more complex material problems, combining the predictive capabilities of neural networks with the optimization prowess of quantum annealing.
Such integration fosters a more comprehensive exploration of material possibilities, fostering innovation and discovery.
Challenges and Future Directions
Despite the promising applications of neural networks and quantum annealing in materials informatics, there are still challenges to address.
These include the need for vast amounts of quality data to train neural networks effectively and the current limitations in quantum hardware, which restrict the scale and complexity of problems quantum annealing can solve.
However, advancements are being made rapidly in both fields.
As quantum computing technology matures and more comprehensive material datasets become available, the impact of these technologies will continue to grow.
The future of materials informatics lies in the further development and integration of machine learning techniques and quantum computing.
Continued research and innovation will pave the way for new materials that could transform industries, from semiconductors and pharmaceuticals to sustainable energy solutions.
Conclusion
Materials informatics is at the forefront of scientific discovery, with neural networks and quantum annealing playing crucial roles in its progression.
These technologies offer new methods for predicting and optimizing materials, shortening the path from discovery to application.
As the field evolves, integrating these advanced tools will be key to unlocking the potential for groundbreaking materials that can address global challenges and drive technological advancement.
資料ダウンロード
QCD調達購買管理クラウド「newji」は、調達購買部門で必要なQCD管理全てを備えた、現場特化型兼クラウド型の今世紀最高の購買管理システムとなります。
ユーザー登録
調達購買業務の効率化だけでなく、システムを導入することで、コスト削減や製品・資材のステータス可視化のほか、属人化していた購買情報の共有化による内部不正防止や統制にも役立ちます。
NEWJI DX
製造業に特化したデジタルトランスフォーメーション(DX)の実現を目指す請負開発型のコンサルティングサービスです。AI、iPaaS、および先端の技術を駆使して、製造プロセスの効率化、業務効率化、チームワーク強化、コスト削減、品質向上を実現します。このサービスは、製造業の課題を深く理解し、それに対する最適なデジタルソリューションを提供することで、企業が持続的な成長とイノベーションを達成できるようサポートします。
オンライン講座
製造業、主に購買・調達部門にお勤めの方々に向けた情報を配信しております。
新任の方やベテランの方、管理職を対象とした幅広いコンテンツをご用意しております。
お問い合わせ
コストダウンが利益に直結する術だと理解していても、なかなか前に進めることができない状況。そんな時は、newjiのコストダウン自動化機能で大きく利益貢献しよう!
(Β版非公開)