- お役立ち記事
- Fundamentals of materials informatics (MI) and its applications using machine learning/Bayesian statistics
月間77,185名の
製造業ご担当者様が閲覧しています*
*2025年2月28日現在のGoogle Analyticsのデータより
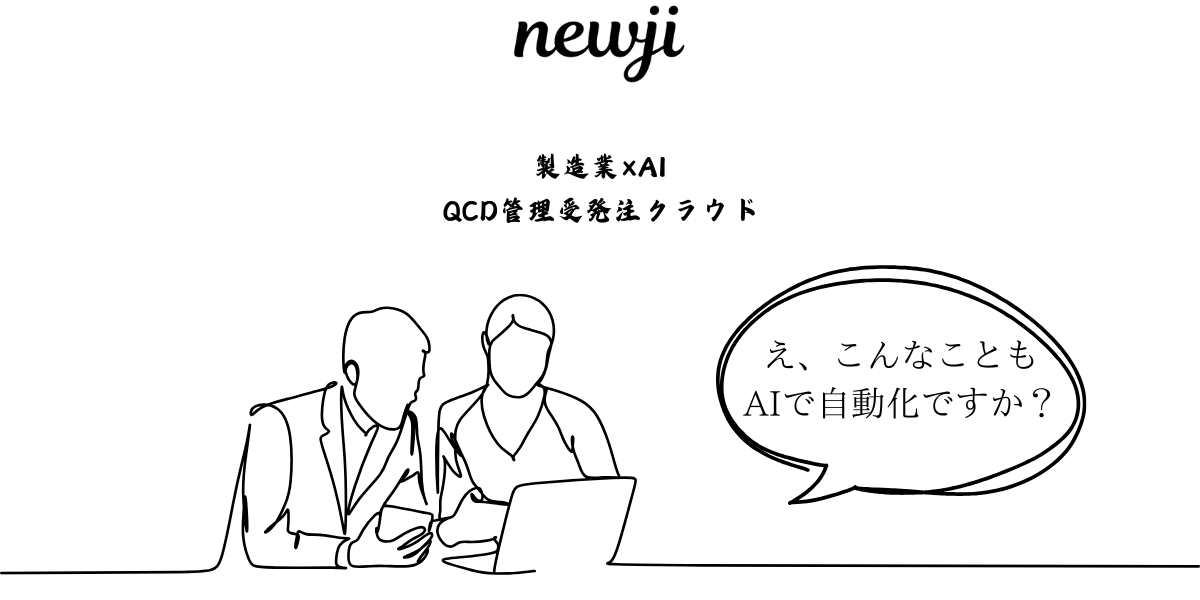
Fundamentals of materials informatics (MI) and its applications using machine learning/Bayesian statistics
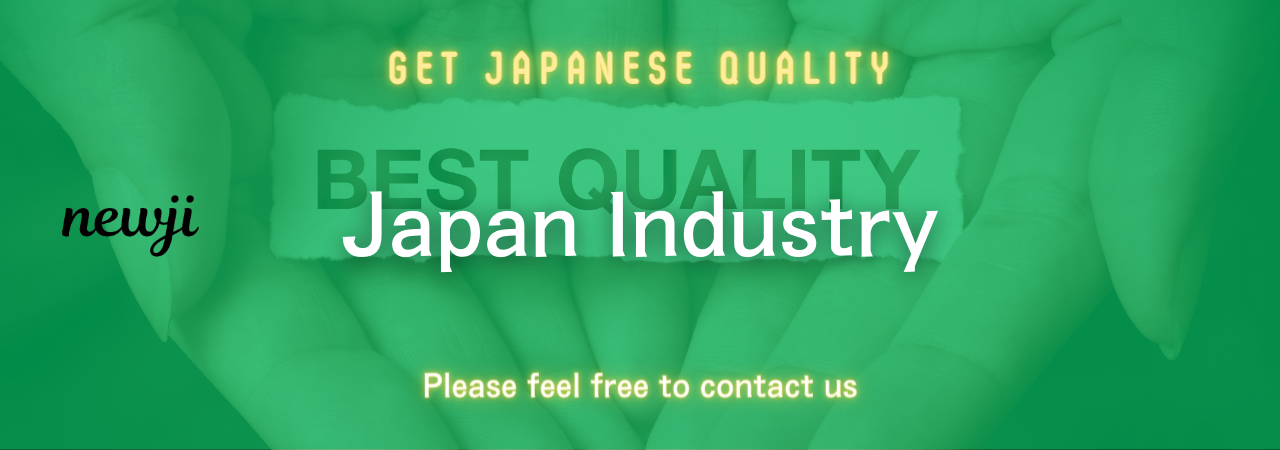
Understanding Materials Informatics
Materials Informatics (MI) is an innovative field that intersects materials science with cutting-edge computational technologies to accelerate the discovery and development of new materials.
By leveraging tools such as machine learning and Bayesian statistics, researchers can predict material properties and behaviors without the need for exhaustive experimental testing.
This approach is crucial for industries aiming to innovate with new materials faster than ever before.
Let’s delve into how MI works and its applications.
The Concept of Materials Informatics
At its core, MI involves the use of data-driven techniques to understand and predict the properties of materials.
Instead of relying solely on traditional experimentation, which can be time-consuming and costly, MI utilizes large datasets and computational models to draw insights.
Machine learning algorithms, for example, can analyze patterns and relationships within data that may be too complex for humans to discern.
This ability allows them to predict how changes in a material’s composition or structure can impact its properties.
The Role of Machine Learning in MI
Machine learning, a subset of artificial intelligence, plays a pivotal role in materials informatics.
Its algorithms are designed to learn from data by identifying patterns and drawing conclusions, making them ideal for handling the intricate datasets often seen in materials research.
Machine learning methods in MI typically involve three main steps: data collection, model training, and prediction.
Initially, data is collected from various sources such as experimental results, scientific literature, and computational simulations.
This dataset then feeds into a machine learning model, which is trained to understand relationships between different material properties.
Once trained, these models can predict the properties of unknown materials, significantly reducing the need for initial physical testing.
Bayesian Statistics: A Probabilistic Approach
Bayesian statistics provide another layer of computational power in materials informatics.
It’s a method based on Bayes’ Theorem, which updates the probability of a hypothesis as more evidence becomes available.
In MI, Bayesian methods can be used to model uncertainties and incorporate prior knowledge into predictions.
This is particularly useful when dealing with complex materials systems where uncertain parameters are present.
By continuously updating predictions with new data, Bayesian statistics ensure that MI approaches remain robust and reliable.
Applications of Materials Informatics
The application areas for materials informatics are vast and growing rapidly.
Let’s explore a few key sectors:
Energy Sector
In the search for clean energy solutions, MI plays an essential role.
For instance, in battery technology, researchers use MI to discover new materials that can offer higher energy density and longer life cycles.
This can expedite the development of more efficient and sustainable energy storage solutions.
Pharmaceuticals and Health
In pharmaceuticals, materials informatics helps identify materials capable of enhancing drug delivery systems.
By predicting the interactions between different compounds, MI can lead to the development of more effective pharmaceutical formulations.
Automotive and Aerospace
The automotive and aerospace industries benefit significantly from materials informatics by optimizing materials for lighter and stronger vehicle components.
This optimization leads to improved fuel efficiency and safety, crucial factors for these sectors.
Challenges in Materials Informatics
Despite its advantages, materials informatics does come with challenges.
Data Quality and Quantity
A significant challenge is the availability and quality of data.
Successful MI approaches rely heavily on large datasets, which are not always available or may contain inconsistencies.
Interdisciplinary Skills
MI requires a blend of materials science knowledge and computational skills, posing a challenge for researchers to possess or collaborate across these disciplines.
Computational Resources
Developing and executing sophisticated models requires substantial computational power, which might not be accessible to all research institutions.
The Future of Materials Informatics
Looking forward, the future of materials informatics is bright and full of potential.
Continual advancements in machine learning, artificial intelligence, and computational power will further push the boundaries of what MI can achieve.
We anticipate more seamless data integration from diverse sources, better computational models, and more accurate predictions.
As more industries recognize the value of MI, its application scope is set to widen, driving innovation and efficiency across sectors.
In conclusion, materials informatics represents a frontier with the potential to transform how we develop materials.
By combining advanced computational techniques with traditional materials science, MI allows for faster, more cost-effective materials innovation.
As we continue to refine these technologies, the possibilities for new and improved materials will only expand, bringing exciting advancements across various industries.
資料ダウンロード
QCD管理受発注クラウド「newji」は、受発注部門で必要なQCD管理全てを備えた、現場特化型兼クラウド型の今世紀最高の受発注管理システムとなります。
ユーザー登録
受発注業務の効率化だけでなく、システムを導入することで、コスト削減や製品・資材のステータス可視化のほか、属人化していた受発注情報の共有化による内部不正防止や統制にも役立ちます。
NEWJI DX
製造業に特化したデジタルトランスフォーメーション(DX)の実現を目指す請負開発型のコンサルティングサービスです。AI、iPaaS、および先端の技術を駆使して、製造プロセスの効率化、業務効率化、チームワーク強化、コスト削減、品質向上を実現します。このサービスは、製造業の課題を深く理解し、それに対する最適なデジタルソリューションを提供することで、企業が持続的な成長とイノベーションを達成できるようサポートします。
製造業ニュース解説
製造業、主に購買・調達部門にお勤めの方々に向けた情報を配信しております。
新任の方やベテランの方、管理職を対象とした幅広いコンテンツをご用意しております。
お問い合わせ
コストダウンが利益に直結する術だと理解していても、なかなか前に進めることができない状況。そんな時は、newjiのコストダウン自動化機能で大きく利益貢献しよう!
(β版非公開)