- お役立ち記事
- Fundamentals of materials informatics using artificial intelligence (AI) and key points for material design and material exploration
月間76,176名の
製造業ご担当者様が閲覧しています*
*2025年3月31日現在のGoogle Analyticsのデータより
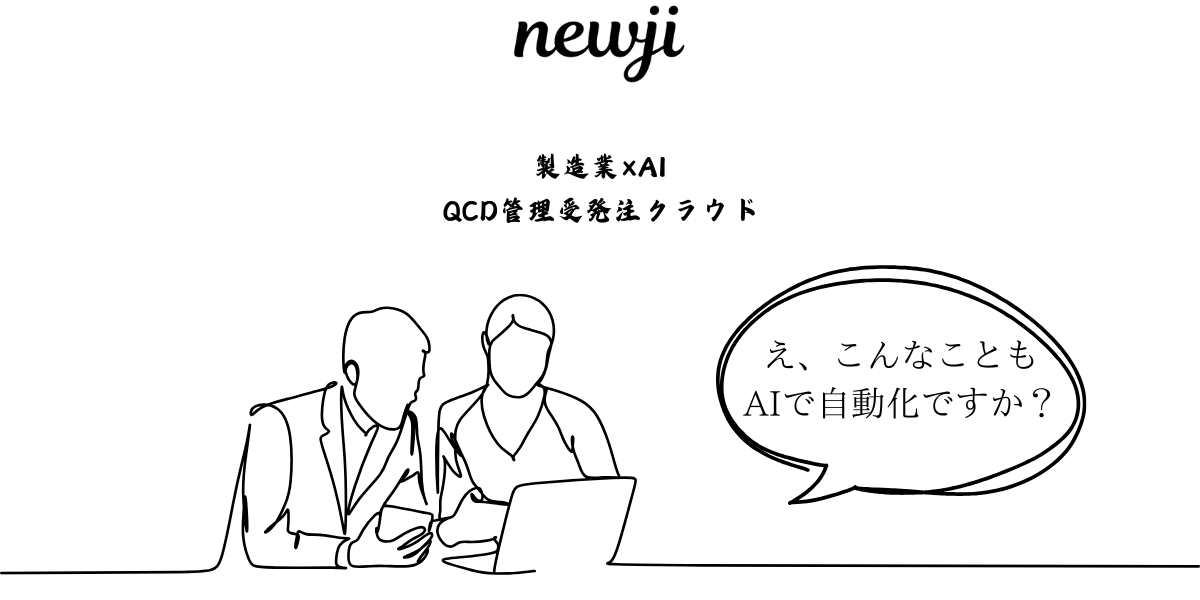
Fundamentals of materials informatics using artificial intelligence (AI) and key points for material design and material exploration
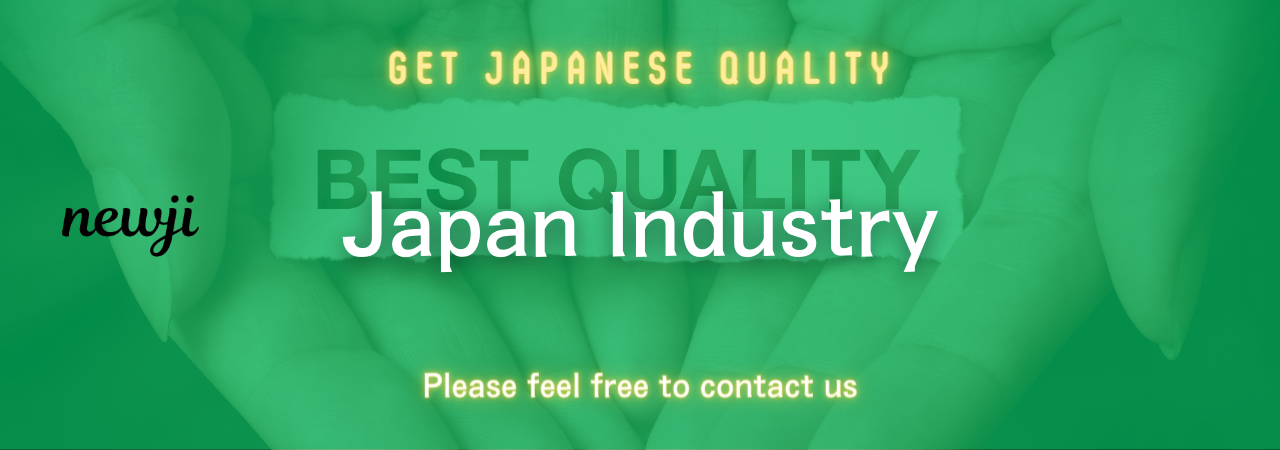
目次
Introduction to Materials Informatics
Materials informatics is an interdisciplinary field that leverages data science to design and discover new materials.
With the advent of advanced artificial intelligence (AI) techniques, materials informatics is poised to revolutionize how materials are researched and developed.
By combining massive datasets with powerful AI algorithms, researchers can predict material properties and optimize processes, reducing both time and cost.
Understanding the fundamentals of this field is crucial for driving innovation in material science and engineering sectors.
The Role of AI in Materials Informatics
AI and machine learning algorithms provide critical tools for analyzing complex datasets in materials science.
These technologies enable the identification of patterns and predictions that might be difficult to deduce through traditional methods.
Machine learning models can analyze vast amounts of data from experiments and simulations to uncover hidden correlations between different material properties.
AI plays a crucial role in optimizing the material discovery process.
It helps in simulating experiments, testing hypotheses, and iterating designs faster than ever before.
By automating these processes, researchers can focus on refining and innovating rather than spending excessive time on routine experiments.
Data Collection and Preprocessing
Any successful materials informatics project hinges on the quality and quantity of data.
The first step involves collecting comprehensive datasets which can include experimental data, simulation outputs, and historical records.
Preprocessing this data is essential to ensure accuracy and reliability.
This involves cleaning the data to remove any inconsistencies or outliers and standardizing the data format for analysis.
Algorithm Selection and Model Training
Choosing the right AI algorithm is crucial in materials informatics.
Popular algorithms include neural networks, decision trees, and support vector machines, each suitable for different types of data and analysis needs.
Once the algorithm is selected, the model training process begins.
This step involves feeding the cleaned and preprocessed data into the model so it can learn the patterns and relationships needed for accurate predictions.
Key Points for Material Design
Designing materials with AI requires careful planning and a strategic approach.
Defining the Objective
One of the first steps is to clearly define the objective.
Understanding what properties are desired in the new material can guide the entire design process.
This can include factors such as strength, durability, conductivity, or specific reaction to environmental stimuli.
Feature Engineering
Feature engineering is critical for material design in informatics.
It involves selecting the right input variables (features) that the algorithm will use to make predictions.
This step may require collaboration between data scientists and material experts to ensure the features are truly representative of the material properties.
Simulation and Optimization
Using AI to simulate various conditions and predict how materials will behave is a core part of the design process.
These simulations help highlight which material designs are most likely to succeed, allowing researchers to focus their efforts on the most promising options.
Optimization algorithms can then refine these designs to enhance specific attributes or improve performance.
Exploring New Materials with AI
The exploration of new materials is a promising application of AI in materials informatics.
AI techniques enable the discovery of novel material structures and compositions that may not have been considered under traditional research methods.
Predicting Material Properties
AI models can predict a wide range of material properties with high accuracy.
By inputting the characteristics of a potential material structure, researchers can quickly gain insights into its properties without needing to create it physically.
This predictive capability helps narrow down potential candidates for further exploration and development.
Generative Models
Generative models are a type of AI technique that can propose entirely new material compositions.
These models, such as Generative Adversarial Networks (GANs) or variational autoencoders, can create new data points that fit within existing datasets.
In materials informatics, they can suggest innovative structures with potentially groundbreaking properties, saving researchers both time and experimental resources.
Challenges and Future Directions
While AI provides numerous benefits in materials informatics, several challenges remain.
Data Limitations and Quality
Access to high-quality, comprehensive datasets continues to be a significant challenge.
In many cases, the available data might be scarce, noisy, or incomplete, which can affect the accuracy and reliability of AI predictions.
Improved data curation and sharing practices will be necessary to overcome this hurdle.
Interdisciplinary Collaboration
Successful materials informatics projects often require collaboration across different fields such as material science, data science, and computer science.
Integrating knowledge from these domains can be complex, yet it is crucial for developing effective AI models.
Efforts to foster interdisciplinary collaboration and communication should be encouraged.
Ethical and Practical Considerations
As with any AI application, ethical considerations are vital.
Ensuring that AI systems in materials informatics are transparent, fair, and accountable is essential.
Moreover, practical considerations involve ensuring that the AI tools remain accessible to a broader range of researchers, not just those at well-funded institutions.
Looking forward, the integration of AI in materials informatics will continue to advance rapidly.
With persistent innovation and collaboration, the potential for discovering novel materials and enhancing existing ones is immense.
The progress in this field not only promises to boost the efficiency of materials research but also to unlock new opportunities for technological advancement across various industries.
資料ダウンロード
QCD管理受発注クラウド「newji」は、受発注部門で必要なQCD管理全てを備えた、現場特化型兼クラウド型の今世紀最高の受発注管理システムとなります。
ユーザー登録
受発注業務の効率化だけでなく、システムを導入することで、コスト削減や製品・資材のステータス可視化のほか、属人化していた受発注情報の共有化による内部不正防止や統制にも役立ちます。
NEWJI DX
製造業に特化したデジタルトランスフォーメーション(DX)の実現を目指す請負開発型のコンサルティングサービスです。AI、iPaaS、および先端の技術を駆使して、製造プロセスの効率化、業務効率化、チームワーク強化、コスト削減、品質向上を実現します。このサービスは、製造業の課題を深く理解し、それに対する最適なデジタルソリューションを提供することで、企業が持続的な成長とイノベーションを達成できるようサポートします。
製造業ニュース解説
製造業、主に購買・調達部門にお勤めの方々に向けた情報を配信しております。
新任の方やベテランの方、管理職を対象とした幅広いコンテンツをご用意しております。
お問い合わせ
コストダウンが利益に直結する術だと理解していても、なかなか前に進めることができない状況。そんな時は、newjiのコストダウン自動化機能で大きく利益貢献しよう!
(β版非公開)