- お役立ち記事
- Fundamentals of model predictive control (MPC) and design applications
Fundamentals of model predictive control (MPC) and design applications
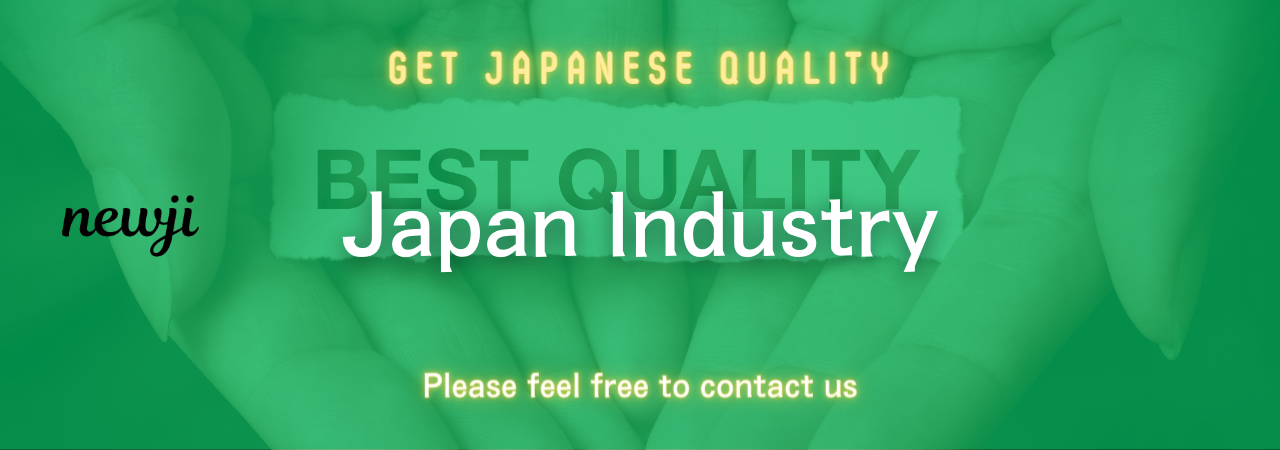
目次
Introduction to Model Predictive Control (MPC)
Model Predictive Control, commonly known as MPC, is a sophisticated control strategy widely used in various industries for dynamic system regulation.
Through the integration of a system model, MPC predicts future behavior and optimizes control moves to achieve desired outcomes.
This approach is sought after for its ability to handle multivariable control problems and constraints efficiently.
MPC is renowned for its predictive capability.
It forecasts future states of a system based on historical data and current states, adjusting controls accordingly to meet the desired targets.
By working in a receding horizon fashion, MPC continuously recalculates the control actions over a moving time frame, making it highly adaptive and robust to changes in system dynamics or external disturbances.
Core Principles of MPC
Modeling the System
At the heart of MPC is the model representing the system to be controlled.
This model captures the dynamic relationship between inputs, outputs, and system states.
The accuracy of the model is pivotal as it directly influences the effectiveness of the predictive control strategy.
Different types of models are used in MPC, including linear, nonlinear, deterministic, and stochastic models, based on the application requirements.
Prediction and Optimization
MPC utilizes the model to predict the future trajectory of the system’s outputs over a specified time horizon.
This prediction considers the current state, control actions, and potential external influences.
The optimization process then seeks to determine the optimal set of control actions that align with the desired performance objectives while respecting operational constraints.
Constraints are intrinsic to MPC’s formulation since real-world systems often have limitations such as input, output boundaries, and rate of change constraints.
MPC accounts for these constraints during the optimization process, ensuring the control actions remain feasible and practical.
Receding Horizon Strategy
A distinctive feature of MPC is its receding horizon approach.
Rather than computing control actions for an indefinite future, MPC focuses on a finite time horizon, typically a few time steps ahead.
Once the optimal sequence of control actions is determined, only the immediate action is implemented.
The horizon then recedes, and the process repeats based on new system measurements and updated states.
This iterative loop offers MPC the flexibility to adapt to state changes and disturbances in real-time.
Design Applications of MPC
Industrial Process Control
MPC is prominently used in process industries such as chemical, petrochemical, and pharmaceuticals to optimize production efficiency and product quality.
By predicting process behavior, MPC manages variables like temperature, pressure, and flow rates in complex industrial systems.
This leads to improved resource utilization, energy savings, and enhanced safety compliance.
Automotive Applications
The automotive industry leverages MPC in various applications, including autonomous driving and advanced driver-assistance systems (ADAS).
MPC helps in trajectory planning and control, vehicle dynamic stabilization, and energy management in hybrid and electric vehicles.
These applications benefit from MPC’s ability to address multivariable control challenges while maintaining system stability and performance.
Energy Management Systems
In the realm of energy management, MPC finds application in smart grids and building energy management systems.
These systems utilize MPC to optimally balance energy supply and demand, integrate renewable energies, and manage storage systems to reduce energy costs and emissions.
MPC’s predictive capabilities enable proactive adjustments in control strategies, contributing to efficient energy utilization and sustainability goals.
Robotics and Automation
Robots and automated systems utilize MPC for precise motion control and decision-making.
MPC allows these systems to predict future trajectories, handle constraints, and perform complex tasks safely and efficiently.
Applications range from robotic arms in manufacturing to drones in aerial operations, showcasing MPC’s versatility in dynamic environments.
Benefits of MPC
MPC provides numerous advantages that make it an appealing choice for sophisticated control applications.
One of its key strengths is its ability to concurrently manage multiple interdependent variables, enhancing overall system coordination and performance.
MPC excels in handling constraints naturally, ensuring all control actions remain within safe and operational boundaries.
This capability is vital in industries where safety and compliance are non-negotiable.
Moreover, MPC’s predictive nature allows for early detection of potential issues and proactive control adjustments.
Such foresight leads to improved stability, reduced variability, and optimized performance, getting the most out of available resources.
Challenges in Implementing MPC
While MPC offers numerous benefits, implementing it effectively poses challenges.
Developing accurate models that truly reflect the system dynamics can be a demanding task, requiring substantial domain expertise and data analysis.
The computational demands of MPC, especially in complex or large-scale systems, may necessitate significant computing resources.
Ensuring real-time performance in fast-paced environments requires efficient optimization algorithms and computing power.
Furthermore, tuning MPC parameters such as prediction horizon and control horizon, along with the cost function, requires careful consideration and expertise to strike a balance between performance and computational feasibility.
Conclusion
Model Predictive Control (MPC) is a robust and flexible control strategy that offers significant advantages for managing dynamic systems across a wide array of applications.
Its ability to predict future states and optimize control actions under constraints makes it invaluable in industries ranging from process control to automotive systems.
Despite the challenges associated with its implementation, advancements in computational power and modeling techniques continue to expand MPC’s applicability and effectiveness.
Understanding the fundamentals and practical applications of MPC is essential for leveraging its full potential in modern control systems.
資料ダウンロード
QCD調達購買管理クラウド「newji」は、調達購買部門で必要なQCD管理全てを備えた、現場特化型兼クラウド型の今世紀最高の購買管理システムとなります。
ユーザー登録
調達購買業務の効率化だけでなく、システムを導入することで、コスト削減や製品・資材のステータス可視化のほか、属人化していた購買情報の共有化による内部不正防止や統制にも役立ちます。
NEWJI DX
製造業に特化したデジタルトランスフォーメーション(DX)の実現を目指す請負開発型のコンサルティングサービスです。AI、iPaaS、および先端の技術を駆使して、製造プロセスの効率化、業務効率化、チームワーク強化、コスト削減、品質向上を実現します。このサービスは、製造業の課題を深く理解し、それに対する最適なデジタルソリューションを提供することで、企業が持続的な成長とイノベーションを達成できるようサポートします。
オンライン講座
製造業、主に購買・調達部門にお勤めの方々に向けた情報を配信しております。
新任の方やベテランの方、管理職を対象とした幅広いコンテンツをご用意しております。
お問い合わせ
コストダウンが利益に直結する術だと理解していても、なかなか前に進めることができない状況。そんな時は、newjiのコストダウン自動化機能で大きく利益貢献しよう!
(Β版非公開)