- お役立ち記事
- Fundamentals of model predictive control technology and effective application methods
月間76,176名の
製造業ご担当者様が閲覧しています*
*2025年3月31日現在のGoogle Analyticsのデータより
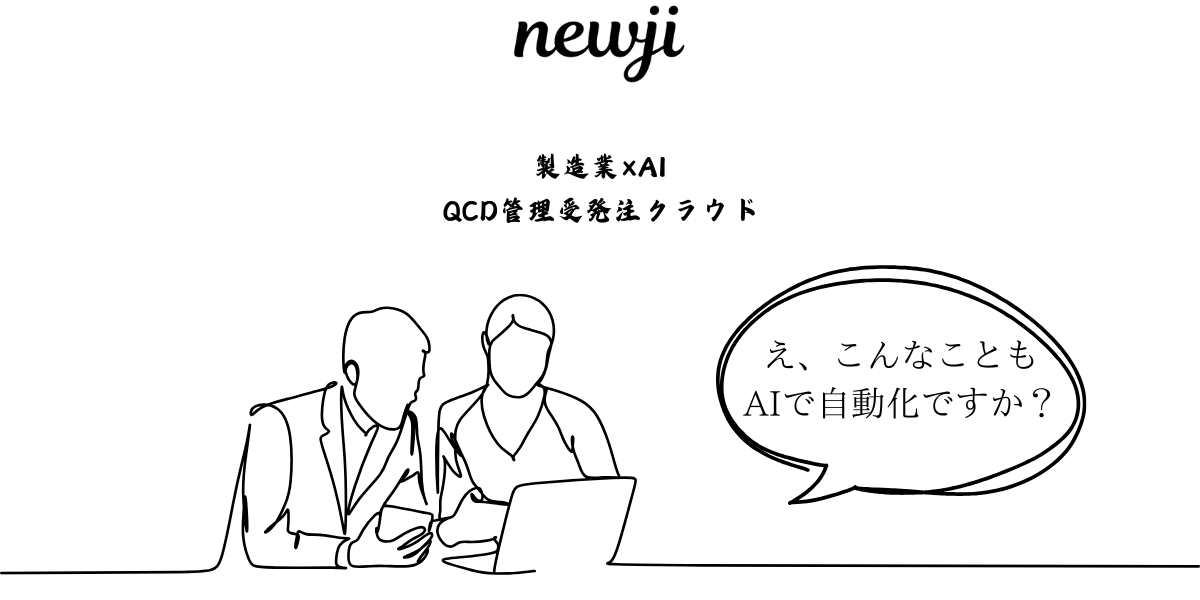
Fundamentals of model predictive control technology and effective application methods
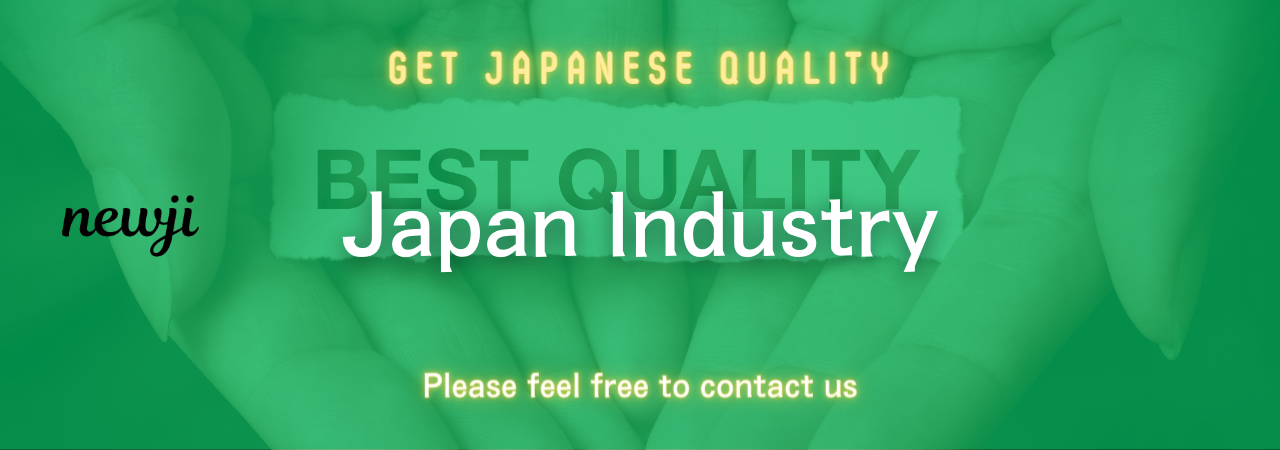
目次
Understanding Model Predictive Control
Model Predictive Control (MPC) is an advanced method of process control that has gained popularity in various industries.
MPC is innovative because it uses a model of the system to predict and optimize the future behavior of a control system.
Unlike traditional control methods, which often operate reactively, MPC uses prediction to take actions that steer the process towards optimal performance.
How Model Predictive Control Works
The fundamental principle of MPC is utilizing a mathematical model to forecast the future outcomes of a system.
By simulating potential scenarios, the controller can make informed decisions about the best course of action.
MPC calculates the best path by solving an optimization problem at each step.
This results in a sequence of control actions that can be applied to achieve the desired outcome while respecting constraints.
The Key Components of MPC
MPC systems consist of several key components.
Firstly, a mathematical model represents the physical processes within the control system.
This model is crucial because it predicts the future outcomes based on different control inputs.
Secondly, there is an optimizer, which is the computational algorithm responsible for determining the optimal control actions.
The optimizer works by minimizing a cost function, balancing performance with constraints.
Lastly, a feedback mechanism constantly checks the real system’s performance against predictions.
It helps adjust the model to stay accurate, which is essential for long-term effectiveness.
Benefits of Model Predictive Control
MPC has several advantages, making it an attractive option for complex control problems.
One significant benefit is its ability to handle multivariable systems efficiently.
MPC can manage interactions between multiple inputs and outputs, ensuring the system operates harmoniously.
Additionally, MPC can accommodate constraints within the control process, such as limits on inputs and outputs.
By considering these constraints, MPC ensures that the system operates safely and within the desired boundaries.
Another advantage of MPC is its predictive capability.
By predicting future system behavior, the controller can make proactive adjustments to avoid potential issues and improve performance.
Challenges and Considerations
Despite its benefits, MPC also has some challenges that need addressing for successful implementation.
One such challenge is the complexity involved in developing accurate mathematical models.
Creating and maintaining these models is essential, but it can be time-consuming and resource-intensive.
Another consideration is the computational demand of solving optimization problems in real-time.
High-speed computation and effective algorithms are necessary to ensure timely responses.
Furthermore, robust control is required when dealing with model inaccuracies or disturbances.
A balance between model accuracy and control robustness is critical for success.
Applications of Model Predictive Control
MPC is used across various industries to optimize operations and improve efficiency.
In the chemical processing industry, for instance, MPC helps control reactors and ensure optimal mixture conditions.
This results in better product quality and reduced energy consumption.
In the automotive industry, MPC is employed in automatic transmission systems to improve fuel efficiency and driving comfort.
It’s also used in vehicle dynamics to enhance safety and performance.
The energy sector benefits from MPC in power plant optimization, ensuring efficient power production while reducing emissions.
MPC is also applied in renewable energy management systems, optimizing the integration of solar and wind power into the grid.
Effective Application Methods
Implementing MPC effectively requires careful planning and execution.
Choosing the right model is critical, as it must accurately represent the system’s dynamics.
Inaccurate models can lead to poor performance, so investing time in model development pays off.
Additionally, defining appropriate constraints is vital.
Constraints should represent real-world limits and safety concerns, enabling the system to operate safely while maximizing performance.
It is also important to regularly update and validate models using actual system data.
Continuous validation ensures the model remains accurate and reliable, maintaining the effectiveness of the MPC strategy.
Conclusion
Model Predictive Control is a powerful tool for optimizing complex systems in various industries.
Its ability to predict and plan ahead offers significant advantages over traditional control methods.
However, successful implementation requires careful model development, robust computation, and effective handling of constraints.
As technology progresses and computational resources grow, MPC will continue to evolve and expand its applications across different fields.
Understanding the fundamentals and effective application methods of MPC can unlock its potential, driving efficiency and innovation in modern process control.
資料ダウンロード
QCD管理受発注クラウド「newji」は、受発注部門で必要なQCD管理全てを備えた、現場特化型兼クラウド型の今世紀最高の受発注管理システムとなります。
ユーザー登録
受発注業務の効率化だけでなく、システムを導入することで、コスト削減や製品・資材のステータス可視化のほか、属人化していた受発注情報の共有化による内部不正防止や統制にも役立ちます。
NEWJI DX
製造業に特化したデジタルトランスフォーメーション(DX)の実現を目指す請負開発型のコンサルティングサービスです。AI、iPaaS、および先端の技術を駆使して、製造プロセスの効率化、業務効率化、チームワーク強化、コスト削減、品質向上を実現します。このサービスは、製造業の課題を深く理解し、それに対する最適なデジタルソリューションを提供することで、企業が持続的な成長とイノベーションを達成できるようサポートします。
製造業ニュース解説
製造業、主に購買・調達部門にお勤めの方々に向けた情報を配信しております。
新任の方やベテランの方、管理職を対象とした幅広いコンテンツをご用意しております。
お問い合わせ
コストダウンが利益に直結する術だと理解していても、なかなか前に進めることができない状況。そんな時は、newjiのコストダウン自動化機能で大きく利益貢献しよう!
(β版非公開)