- お役立ち記事
- Fundamentals of model predictive control technology and key points for successful application
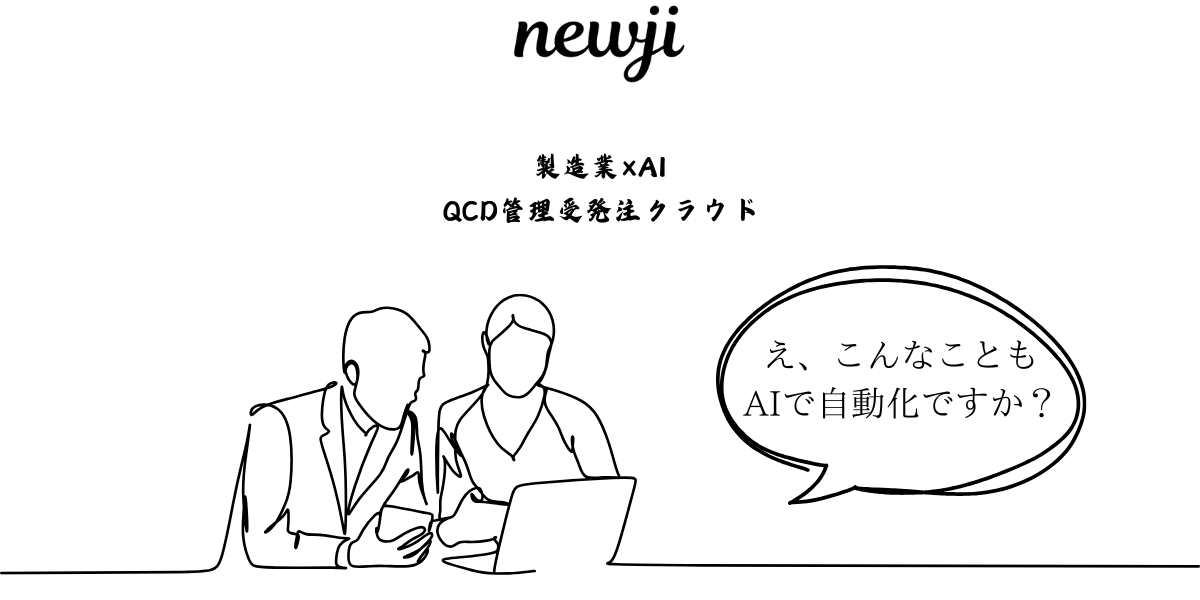
Fundamentals of model predictive control technology and key points for successful application
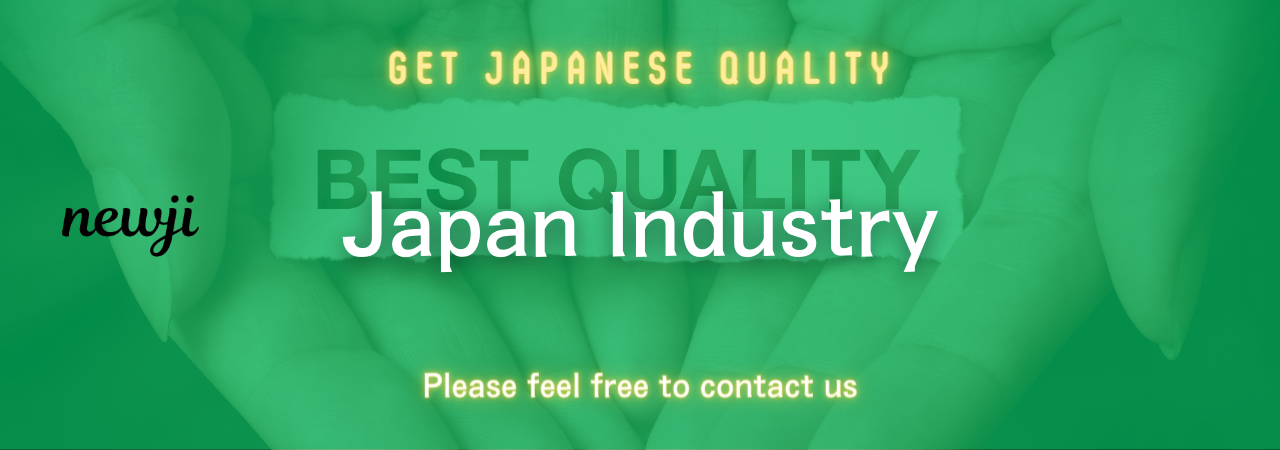
目次
What is Model Predictive Control?
Model Predictive Control (MPC) is a sophisticated control strategy used in various industries for managing multivariable processes.
It is a type of control algorithm that utilizes a mathematical model to predict the future behavior of a process and make real-time decisions to achieve desired outcomes.
The main advantage of MPC is its ability to handle complex systems with multiple inputs and outputs while considering constraints and optimizing performance.
Basic Principles of Model Predictive Control
The core concept of MPC revolves around the creation of a predictive model of the system being controlled.
This model is used to forecast future behavior over a predefined horizon.
Based on these predictions, the control algorithm determines the best course of actions by solving an optimization problem at each time step.
The optimization considers various constraints such as physical limitations, safety margins, and economic objectives.
Once the optimal control actions are determined, the first action is implemented in the system.
The process is then repeated at the next time step with updated measurements.
This closed-loop feedback mechanism ensures that the system continuously adapts to changing conditions.
Key Components of Model Predictive Control
There are several key components that are essential for the successful implementation of MPC:
1. **System Model**: A mathematical representation of the process dynamics is crucial for accurate predictions.
The model can be derived from first principles, data-driven methods, or a combination of both.
2. **Prediction Horizon**: This is the timeframe over which future predictions are made.
A longer horizon may provide better foresight but requires more computational resources.
3. **Control Horizon**: This represents the number of future control actions considered.
While a longer control horizon can improve performance, it increases complexity.
4. **Constraints**: MPC can handle constraints on inputs, outputs, and states, ensuring safe and efficient operation.
Constraints may include equipment limits, safety constraints, and environmental regulations.
5. **Objective Function**: This defines the performance criteria that the MPC aims to optimize, such as minimizing energy consumption or maximizing throughput.
Applications of Model Predictive Control
MPC is employed in a wide range of applications across different industries:
– **Chemical Processes**: In chemical and petrochemical industries, MPC is used to optimize the operation of reactors, distillation columns, and other unit operations for improved efficiency and reduced emissions.
– **Automotive**: MPC is used in advanced driver assistance systems (ADAS) for functions such as adaptive cruise control and lane-keeping, enhancing vehicle safety and comfort.
– **Aerospace**: In aerospace, MPC is applied in flight control systems to manage aircraft dynamics and ensure stable and efficient flight.
– **Energy**: MPC is instrumental in optimizing power generation and distribution systems, including wind farms and smart grids, to increase reliability and reduce costs.
– **Robotics**: MPC enables robots to perform complex tasks with precision by accounting for dynamic interactions and environmental constraints.
Benefits of Model Predictive Control
MPC offers numerous advantages that contribute to its widespread adoption:
– **Multivariable Control**: MPC handles multiple inputs and outputs simultaneously, making it suitable for complex processes with interdependent variables.
– **Constraint Handling**: The ability to incorporate constraints into the control formulation ensures that operational limits are respected, enhancing safety and compliance.
– **Optimization**: MPC optimizes the control actions to achieve the best possible performance, often leading to significant improvements in efficiency and productivity.
– **Adaptability**: MPC’s feedback mechanism allows it to adapt to changing conditions and disturbances, maintaining optimal performance in dynamic environments.
Challenges in Implementing Model Predictive Control
While MPC offers substantial benefits, its implementation requires careful consideration of several challenges:
– **Complexity**: The computational complexity of solving optimization problems in real-time can be demanding, necessitating advanced algorithms and hardware support.
– **Model Accuracy**: The predictive accuracy of MPC heavily relies on the quality of the system model.
Inaccuracies in the model can degrade control performance.
– **Tuning and Calibration**: Properly tuning MPC parameters, such as prediction horizon and control weights, is crucial to achieving desired performance.
– **Integration**: Integrating MPC with existing control systems and infrastructure may require adjustments and careful handling to ensure seamless operation.
Key Points for Successful Application of Model Predictive Control
To successfully apply MPC, it is essential to consider the following key points:
1. **Model Development**: Invest time and resources in developing an accurate and robust model that captures the essential dynamics of the system.
2. **Optimization Algorithms**: Employ efficient optimization algorithms that can handle the computational demands of MPC in real-time applications.
3. **Constraint Definition**: Clearly define constraints and performance objectives to align MPC behavior with the desired outcomes and regulatory requirements.
4. **Parameter Tuning**: Systematically tune the MPC parameters through simulation and iterative testing to achieve optimal performance.
5. **Monitoring and Maintenance**: Implement monitoring tools to track MPC performance and model accuracy over time, enabling timely updates and improvements.
MPC is a powerful technology that, when implemented correctly, can drive significant improvements in control performance and operational efficiency.
By understanding its principles, benefits, and challenges, industries can harness the potential of MPC for enhanced process management and decision-making.
資料ダウンロード
QCD調達購買管理クラウド「newji」は、調達購買部門で必要なQCD管理全てを備えた、現場特化型兼クラウド型の今世紀最高の購買管理システムとなります。
ユーザー登録
調達購買業務の効率化だけでなく、システムを導入することで、コスト削減や製品・資材のステータス可視化のほか、属人化していた購買情報の共有化による内部不正防止や統制にも役立ちます。
NEWJI DX
製造業に特化したデジタルトランスフォーメーション(DX)の実現を目指す請負開発型のコンサルティングサービスです。AI、iPaaS、および先端の技術を駆使して、製造プロセスの効率化、業務効率化、チームワーク強化、コスト削減、品質向上を実現します。このサービスは、製造業の課題を深く理解し、それに対する最適なデジタルソリューションを提供することで、企業が持続的な成長とイノベーションを達成できるようサポートします。
オンライン講座
製造業、主に購買・調達部門にお勤めの方々に向けた情報を配信しております。
新任の方やベテランの方、管理職を対象とした幅広いコンテンツをご用意しております。
お問い合わせ
コストダウンが利益に直結する術だと理解していても、なかなか前に進めることができない状況。そんな時は、newjiのコストダウン自動化機能で大きく利益貢献しよう!
(Β版非公開)