- お役立ち記事
- Fundamentals of model predictive control technology, model creation methods, and introduction points
月間73,982名の
製造業ご担当者様が閲覧しています*
*2025年1月31日現在のGoogle Analyticsのデータより
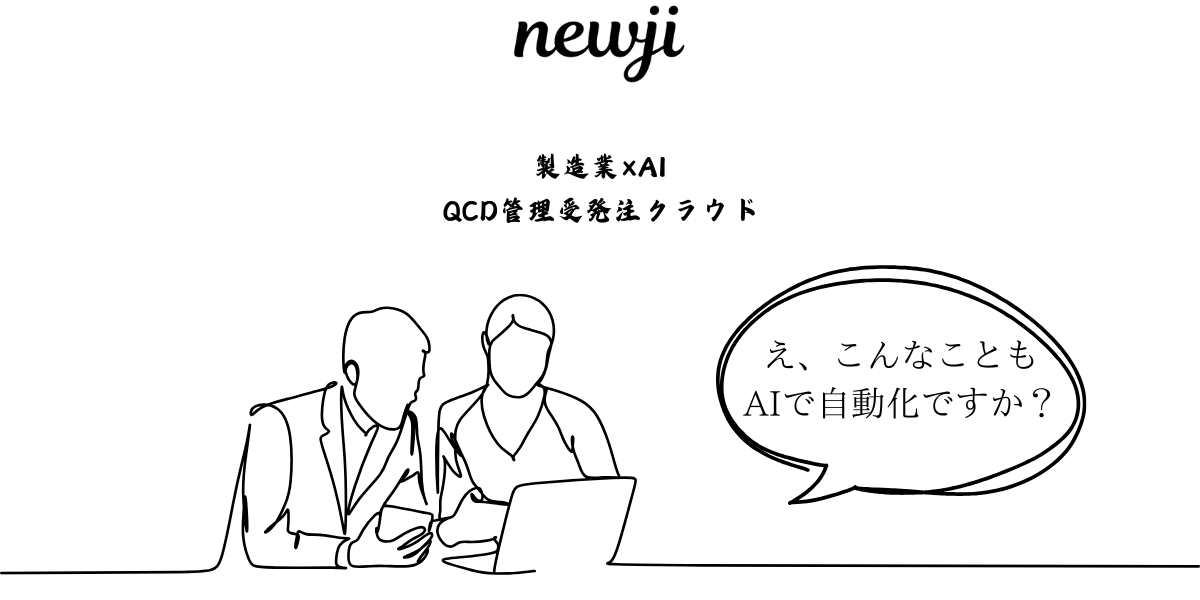
Fundamentals of model predictive control technology, model creation methods, and introduction points
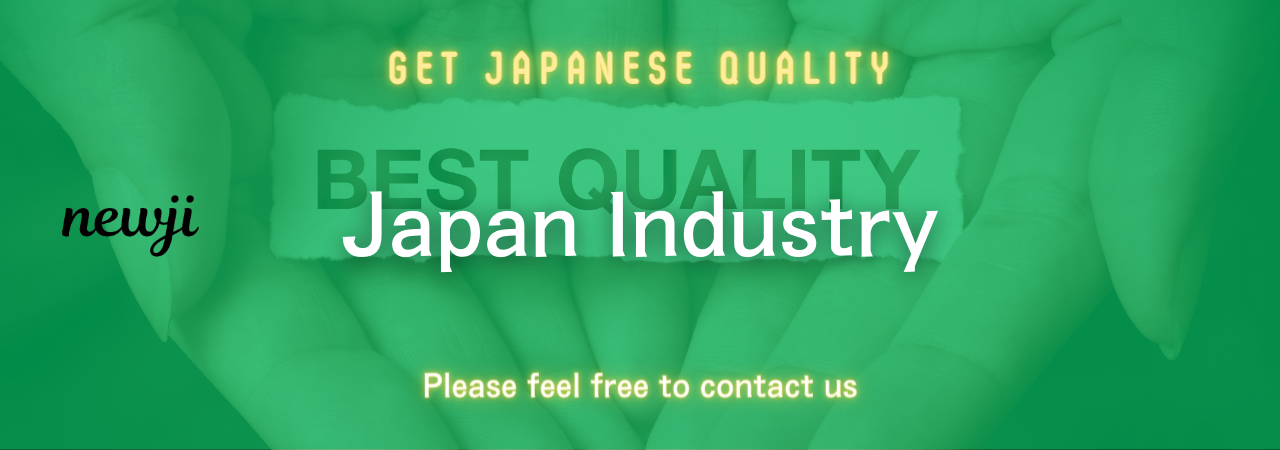
目次
Understanding Model Predictive Control (MPC)
Model Predictive Control (MPC) is a powerful control strategy used extensively in various industries to manage and control complex systems.
Unlike traditional control methods, MPC considers future states of a system, using a dynamic model to predict and optimize control actions.
By evaluating multiple future states, MPC can provide more robust and accurate control solutions, ideal for industries where precision is crucial.
Core Components of Model Predictive Control
At its core, MPC involves three main components: the dynamic model, the prediction horizon, and the optimization algorithm.
The dynamic model represents the system’s behavior over time.
It can be a mathematical model or derived from empirical data, capturing the essential dynamics and interactions within the system.
The prediction horizon is the time frame over which predictions are made.
It is usually measured in discrete time steps.
During each time step, the MPC makes predictions about future states and determines the optimal control actions to meet desired objectives.
The optimization algorithm works to find the best set of control actions that will optimize the objective function, such as minimizing energy consumption or maximizing production efficiency, while adhering to constraints.
These constraints could include limitations on system inputs and outputs, ensuring that safe and feasible solutions are found.
Model Creation Methods
Creating a reliable model is critical for effective MPC implementation.
There are several methods for model creation, each suited for different types of systems and data availability.
First-Principles Modeling
First-principles modeling involves using fundamental physical laws and equations to describe the system.
This method is often used when a deep understanding of the physical processes involved is available.
Such models provide valuable insights and allow for high accuracy but can be complex and require detailed knowledge of the system.
Data-Driven Modeling
When first-principles models are impractical, data-driven modeling can be employed.
This approach relies on empirical data to develop models using techniques like machine learning and system identification.
While easier to implement when vast amounts of data are available, it generally results in models that are specific to the conditions under which the data was collected.
Hybrid Modeling
Hybrid modeling combines elements of both first-principles and data-driven methods.
By integrating the strengths of each, hybrid models can offer a balance between accuracy and flexibility.
They provide a powerful tool for systems where both physical and empirical insights are essential.
Benefits of Model Predictive Control
Model Predictive Control offers numerous advantages over traditional control methods, making it a popular choice in industries such as chemical processes, automotive, aerospace, and manufacturing.
Improved Performance
MPC optimizes control actions by considering future states, allowing for precise adjustments that improve overall system performance.
This predictive approach reduces variability and enhances stability, resulting in better product quality and reduced waste.
Greater Flexibility
MPC’s ability to handle multi-variable systems and complex dynamics provides unmatched flexibility.
It can easily adapt to changes in operating conditions or system configuration, ensuring consistent performance without extensive re-tuning.
Cost Efficiency
By optimizing resource usage and minimizing energy consumption, MPC contributes to reduced operational costs.
Efficient control reduces wear and tear on equipment, prolonging life cycles and minimizing maintenance expenses.
Safety and Compliance
Incorporating constraints into MPC ensures that system operations remain within safe limits, abiding by regulatory standards.
This feature is particularly important in industries where safety and compliance are of utmost importance.
When to Introduce Model Predictive Control
Deciding when to implement MPC in a given system depends on several factors, including system complexity, performance requirements, and cost considerations.
Complex Systems
MPC is often introduced in systems with complex interactions and dynamics that are difficult to control with traditional methods.
Examples include chemical reactors, advanced manufacturing processes, and aerospace systems.
High Precision Needs
Industries with tight precision requirements, such as semiconductor manufacturing or pharmaceuticals, benefit from MPC’s ability to maintain stringent quality controls.
Model predictive control ensures products meet exact specifications, reducing defects and errors.
Cost-Benefit Analysis
Before introducing MPC, conduct a cost-benefit analysis to assess potential gains in efficiency and performance against implementation costs.
MPC may be particularly beneficial when the projected savings from improved performance outweigh the costs of modeling and controller design.
Existing Control Challenges
If traditional control strategies are insufficient in managing system behavior, resulting in frequent operational issues or performance bottlenecks, the introduction of MPC could provide the necessary enhancements.
Conclusion
Model Predictive Control is an advanced strategy that offers significant benefits in controlling complex systems.
By leveraging dynamic models, optimization algorithms, and a predictive approach, MPC improves system performance, flexibility, and safety.
Whether facing complex system dynamics, high precision demands, or seeking operational efficiency, introducing MPC can elevate your control strategy, leading to substantial gains in productivity and cost savings.
資料ダウンロード
QCD管理受発注クラウド「newji」は、受発注部門で必要なQCD管理全てを備えた、現場特化型兼クラウド型の今世紀最高の受発注管理システムとなります。
ユーザー登録
受発注業務の効率化だけでなく、システムを導入することで、コスト削減や製品・資材のステータス可視化のほか、属人化していた受発注情報の共有化による内部不正防止や統制にも役立ちます。
NEWJI DX
製造業に特化したデジタルトランスフォーメーション(DX)の実現を目指す請負開発型のコンサルティングサービスです。AI、iPaaS、および先端の技術を駆使して、製造プロセスの効率化、業務効率化、チームワーク強化、コスト削減、品質向上を実現します。このサービスは、製造業の課題を深く理解し、それに対する最適なデジタルソリューションを提供することで、企業が持続的な成長とイノベーションを達成できるようサポートします。
製造業ニュース解説
製造業、主に購買・調達部門にお勤めの方々に向けた情報を配信しております。
新任の方やベテランの方、管理職を対象とした幅広いコンテンツをご用意しております。
お問い合わせ
コストダウンが利益に直結する術だと理解していても、なかなか前に進めることができない状況。そんな時は、newjiのコストダウン自動化機能で大きく利益貢献しよう!
(β版非公開)