- お役立ち記事
- Fundamentals of multivariate analysis and application to data analysis practice
月間77,185名の
製造業ご担当者様が閲覧しています*
*2025年2月28日現在のGoogle Analyticsのデータより
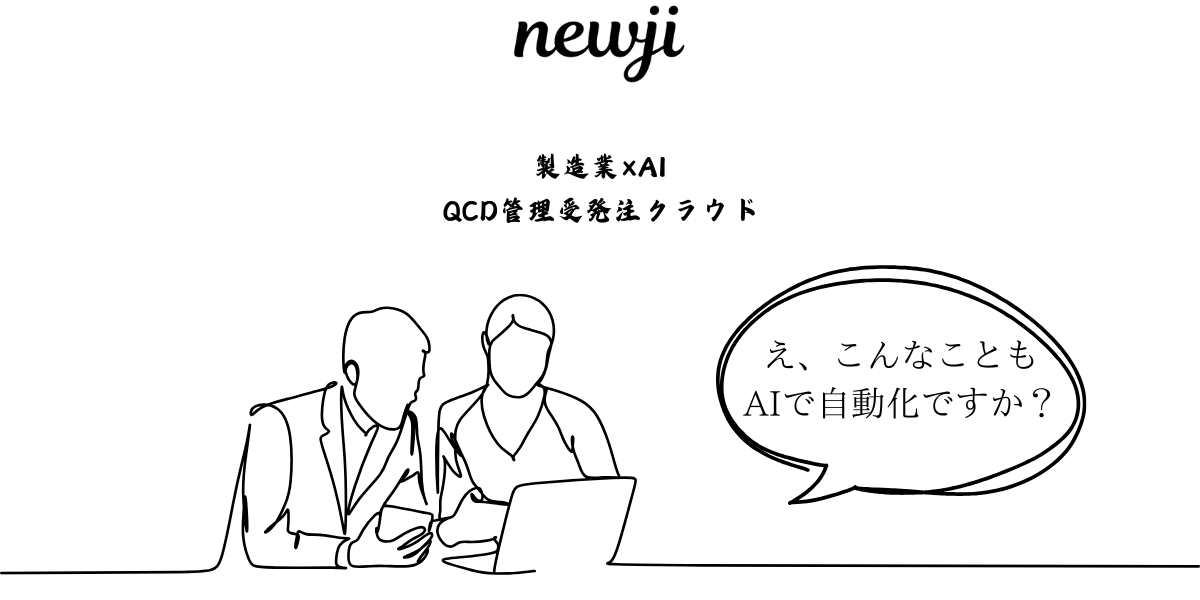
Fundamentals of multivariate analysis and application to data analysis practice
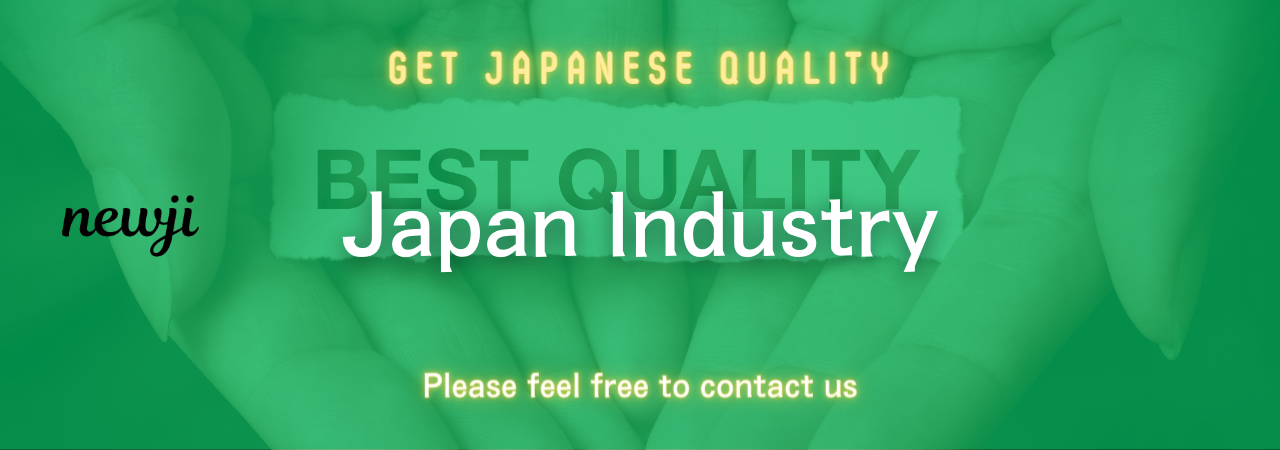
目次
Understanding Multivariate Analysis
Multivariate analysis is a powerful statistical approach widely used in interpreting complex data sets.
It involves observing and analyzing more than one statistical outcome variable at a time.
Understanding the fundamentals of multivariate analysis is crucial because it allows researchers and analysts to investigate relationships between multiple variables simultaneously, offering deeper insights into the data.
At its core, multivariate analysis takes into account multiple measurements and their interrelationships all at once.
This is different from univariate or bivariate analyses where only one or two variables are examined at a time.
By doing so, it embraces the complexity and richness of real-world data where variables often do not operate in isolation but interact with each other.
In many practical scenarios, especially in fields like marketing, finance, biology, and social sciences, datasets are inherently multivariate.
For instance, a market researcher may want to understand how several factors such as age, income, and social influence affect consumer purchasing decisions all at once.
With multivariate analysis, these relationships can be simultaneously examined, leading to more comprehensive insights.
Types of Multivariate Analysis
Multivariate analysis consists of various methods, each suited for different types of data and specific objectives.
Principal Component Analysis (PCA)
Principal Component Analysis is a method used to reduce the dimensionality of a dataset, while retaining most of the variability present in the dataset.
It does so by transforming original variables into a new set of variables, known as principal components.
These components are orthogonal and are ordered in such a way that the first few retain most of the variation present in all of the original variables.
PCA is particularly useful in data compression and visualization, helping to simplify datasets with many variables while minimizing information loss.
Factor Analysis
Factor Analysis is a technique that seeks to identify underlying relationships between varied variables.
It does so by modeling observed variables and their influences from a smaller number of unobserved variables known as factors.
This method is typically employed in fields like psychology and social sciences to identify latent constructs.
Cluster Analysis
Cluster Analysis is a classification approach that divides a dataset into groups or clusters.
The goal is to ensure that objects within a cluster have greater similarity to each other than to those in other clusters.
Applications of cluster analysis include market segmentation, social network analysis, and pattern recognition, among others.
Discriminant Analysis
Discriminant Analysis is used to determine which variables discriminate between two or more naturally occurring groups.
It helps in classifying a set of observations into predefined classes.
Commonly used in marketing to profile consumers, discriminant analysis helps understanding which demographic or behavioral characteristics distinguish different buying habits.
Multiple Regression Analysis
Multiple Regression Analysis extends the basic idea of simple linear regression by allowing the model to include multiple predictor variables.
This analysis helps in understanding the relationship between one dependent variable and two or more independent variables, providing predictions and explanations for variable behaviors.
Applications in Data Analysis Practice
Multivariate analysis has a wide range of applications spanning numerous fields, due to its capability to handle and interpret complex datasets.
Healthcare and Medicine
In healthcare, multivariate analysis is crucial for understanding the relationships between various health indicators and patient outcomes.
This can aid in identifying risk factors associated with diseases, optimizing treatment plans, and predicting patient responses to treatments.
Finance and Economics
In the realm of finance, multivariate analysis is used for risk assessment and portfolio management.
It can evaluate the correlation between various financial instruments and macroeconomic indicators, facilitating more informed investment decisions.
Environmental Studies
Environmental scientists use multivariate analysis to study ecological and environmental data.
This helps in modeling climate change impacts, evaluating pollution levels, and understanding the dynamics of ecosystems.
Social Sciences
In social sciences, multivariate analysis is employed to examine survey data, model social behaviors, and analyze psychological traits.
This helps researchers understand complex social patterns and relationships more clearly.
Marketing and Consumer Research
Marketers use multivariate analysis to enhance consumer satisfaction and improve product positioning.
It helps identify target customer segments, uncover purchasing patterns, and evaluate marketing strategies.
Challenges and Considerations
While multivariate analysis provides powerful insights, it also poses its own set of challenges.
One major challenge is managing the complexity of the data and the potential for multicollinearity, where two or more variables in a model are highly correlated.
This can distort the results of the analysis if not handled properly.
Additionally, interpreting multivariate results can be complex, requiring expertise and a deep understanding of statistical principles.
Researchers must also ensure the quality and reliability of their data, as poor data quality can lead to misleading conclusions.
Despite these challenges, the application of multivariate analysis remains invaluable in today’s data-driven world, offering comprehensive solutions to complex problems by taking into account the interdependencies within the data.
Conclusion
In summary, mastering the fundamentals of multivariate analysis unlocks numerous possibilities for data analysis practice.
It provides a more nuanced understanding of complex datasets through its variety of methods—PCA, factor analysis, cluster analysis, discriminant analysis, and multiple regression analysis—each offering unique perspectives and insights.
The applications across different fields showcase its versatility and importance, reinforcing why it remains an essential tool for analysts and researchers alike.
Understanding and navigating its complexities promises rewarding outcomes in the quest to unlock deeper data insights.
資料ダウンロード
QCD管理受発注クラウド「newji」は、受発注部門で必要なQCD管理全てを備えた、現場特化型兼クラウド型の今世紀最高の受発注管理システムとなります。
ユーザー登録
受発注業務の効率化だけでなく、システムを導入することで、コスト削減や製品・資材のステータス可視化のほか、属人化していた受発注情報の共有化による内部不正防止や統制にも役立ちます。
NEWJI DX
製造業に特化したデジタルトランスフォーメーション(DX)の実現を目指す請負開発型のコンサルティングサービスです。AI、iPaaS、および先端の技術を駆使して、製造プロセスの効率化、業務効率化、チームワーク強化、コスト削減、品質向上を実現します。このサービスは、製造業の課題を深く理解し、それに対する最適なデジタルソリューションを提供することで、企業が持続的な成長とイノベーションを達成できるようサポートします。
製造業ニュース解説
製造業、主に購買・調達部門にお勤めの方々に向けた情報を配信しております。
新任の方やベテランの方、管理職を対象とした幅広いコンテンツをご用意しております。
お問い合わせ
コストダウンが利益に直結する術だと理解していても、なかなか前に進めることができない状況。そんな時は、newjiのコストダウン自動化機能で大きく利益貢献しよう!
(β版非公開)