- お役立ち記事
- Fundamentals of multivariate analysis and application to data analysis practice
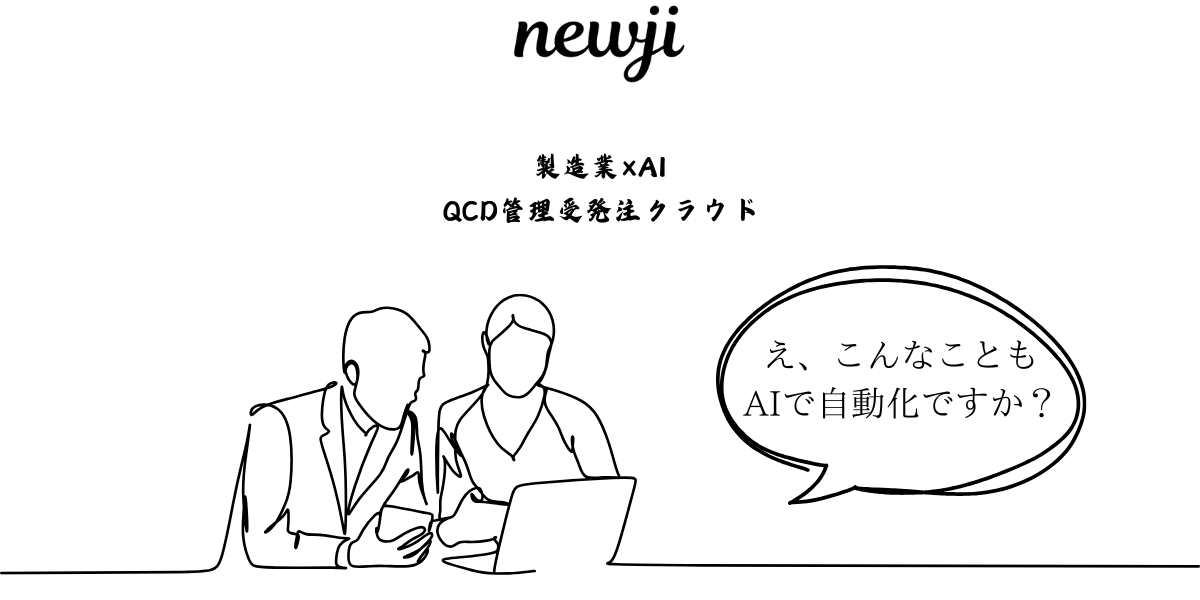
Fundamentals of multivariate analysis and application to data analysis practice
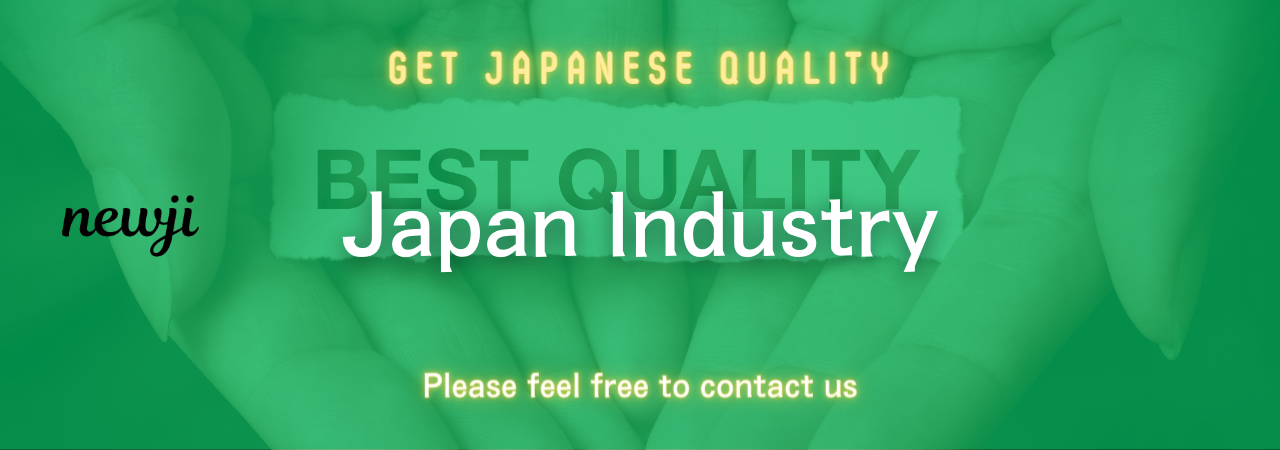
目次
Understanding Multivariate Analysis
Multivariate analysis is a pivotal concept in the field of statistics and data science.
It involves observing and analyzing more than one statistical outcome variable at a time.
This approach is essential when dealing with complex data, where relationships between variables can significantly impact results.
At its core, multivariate analysis allows researchers and data scientists to understand patterns and relationships between various variables.
By considering multiple factors simultaneously, it’s possible to derive more comprehensive insights and make informed decisions based on complex datasets.
The Importance of Multivariate Analysis
In real-world data scenarios, multiple variables often influence outcomes.
For instance, in marketing, customer behavior is affected by numerous factors like age, income, preferences, and more.
Analyzing these variables in isolation can lead to inaccurate conclusions.
This is where multivariate analysis comes into play, offering a complete picture by evaluating all relevant variables together.
Furthermore, this analysis helps in reducing data dimensionality, identifying influential factors, and determining the relationships (correlations) between different variables.
It is also useful in predictive modeling to forecast future outcomes based on current data.
Types of Multivariate Analysis
There are several techniques within multivariate analysis, each serving different purposes based on the nature of the data and the research objectives.
Principal Component Analysis (PCA)
PCA is a technique used to reduce the dimensionality of a data set.
It transforms a large set of variables into a smaller one that still contains most of the information of the large set.
It’s particularly useful for simplifying complex datasets while retaining their essential information.
Factor Analysis
This method is used to identify underlying relationships between variables.
Factor analysis looks to uncover latent structures within a set of observed variables, allowing researchers to understand the factors influencing them.
Cluster Analysis
Cluster analysis is used to group a set of objects in such a way that objects in the same group, or cluster, are more similar to each other than to those in other clusters.
This technique is commonly used in market segmentation, social network analysis, and image processing.
Discriminant Analysis
Discriminant analysis is used to predict a categorical dependent variable by one or more continuous or binary independent variables.
It is often employed to classify a dataset into two or more groups based on their attributes.
Multivariate Regression Analysis
This is an extension of the linear regression model that allows for multiple dependent variables to be predicted by a single set of independent variables.
It provides a more holistic approach to understanding relationships and predicting outcomes.
Applications in Data Analysis Practice
The use of multivariate analysis extends across various domains and industries, providing valuable insights into different areas of interest.
Healthcare
In healthcare, multivariate analysis helps professionals understand the effects of multiple risk factors on health outcomes.
For example, it can help in assessing the impact of lifestyle, genetics, and environment on diseases, concluding better-informed healthcare interventions.
Finance
In the finance industry, analysts use multivariate analysis to manage risk and forecast financial trends.
By analyzing multiple financial metrics simultaneously, it’s possible to create more accurate predictive models.
Marketing
Marketers use multivariate analysis to understand customer preferences and behaviors.
By evaluating various customer-related data, businesses can target specific demographic segments more effectively, tailoring their marketing strategies for better results.
Environmental Studies
Environmental scientists employ multivariate analysis to study the interaction between different environmental factors.
This can include examining how pollution levels, climate conditions, and human activities collectively impact ecosystems.
Challenges and Considerations
While multivariate analysis is robust and informative, it comes with its own set of challenges.
One of the primary concerns is the complexity involved in interpreting results.
With several variables in play, drawing clear conclusions can be challenging.
There’s also the risk of multicollinearity, where independent variables in a model are correlated, potentially leading to unreliable estimates of their effects.
Proper data preprocessing and model validation are crucial to ensure the accuracy and reliability of findings.
The Need for Specialized Software
Handling multivariate data often requires specialized statistical software.
Tools like R, Python, SPSS, and SAS are commonly used.
They offer powerful functionalities for running complex analyses and visualizing results effectively.
Interdisciplinary Collaboration
Professionals from different disciplines often need to collaborate when applying multivariate analysis.
For example, data scientists might work with subject matter experts to ensure that the models are correctly specified and to interpret results accurately.
Conclusion
Multivariate analysis is an invaluable tool for uncovering deeper insights in data analysis.
By considering many variables at once, it allows researchers and analysts to make more informed decisions, identify trends, and predict future outcomes effectively.
Despite its complexity, with the right tools and understanding, multivariate analysis empowers industries to harness the full potential of their data.
As the amount of data continues to grow, the ability to analyze it through multivariate techniques will become increasingly crucial in driving innovation and achieving insights that inform strategy and decision-making across various fields.
資料ダウンロード
QCD調達購買管理クラウド「newji」は、調達購買部門で必要なQCD管理全てを備えた、現場特化型兼クラウド型の今世紀最高の購買管理システムとなります。
ユーザー登録
調達購買業務の効率化だけでなく、システムを導入することで、コスト削減や製品・資材のステータス可視化のほか、属人化していた購買情報の共有化による内部不正防止や統制にも役立ちます。
NEWJI DX
製造業に特化したデジタルトランスフォーメーション(DX)の実現を目指す請負開発型のコンサルティングサービスです。AI、iPaaS、および先端の技術を駆使して、製造プロセスの効率化、業務効率化、チームワーク強化、コスト削減、品質向上を実現します。このサービスは、製造業の課題を深く理解し、それに対する最適なデジタルソリューションを提供することで、企業が持続的な成長とイノベーションを達成できるようサポートします。
オンライン講座
製造業、主に購買・調達部門にお勤めの方々に向けた情報を配信しております。
新任の方やベテランの方、管理職を対象とした幅広いコンテンツをご用意しております。
お問い合わせ
コストダウンが利益に直結する術だと理解していても、なかなか前に進めることができない状況。そんな時は、newjiのコストダウン自動化機能で大きく利益貢献しよう!
(Β版非公開)