- お役立ち記事
- Fundamentals of multivariate analysis, applications to data analysis, and its key points
月間76,176名の
製造業ご担当者様が閲覧しています*
*2025年3月31日現在のGoogle Analyticsのデータより
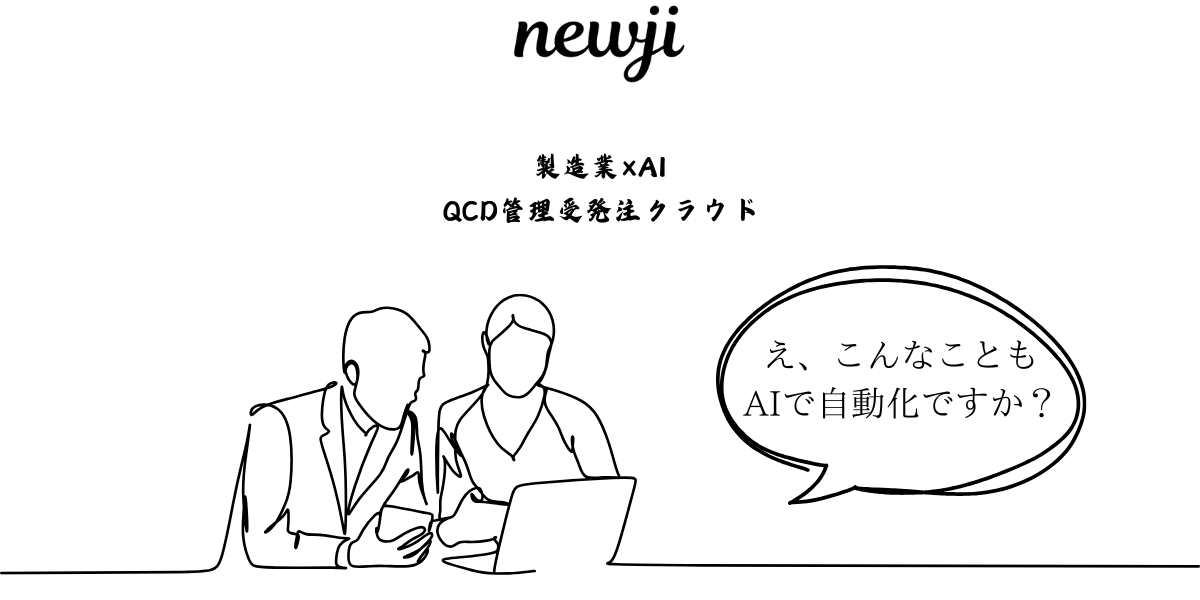
Fundamentals of multivariate analysis, applications to data analysis, and its key points
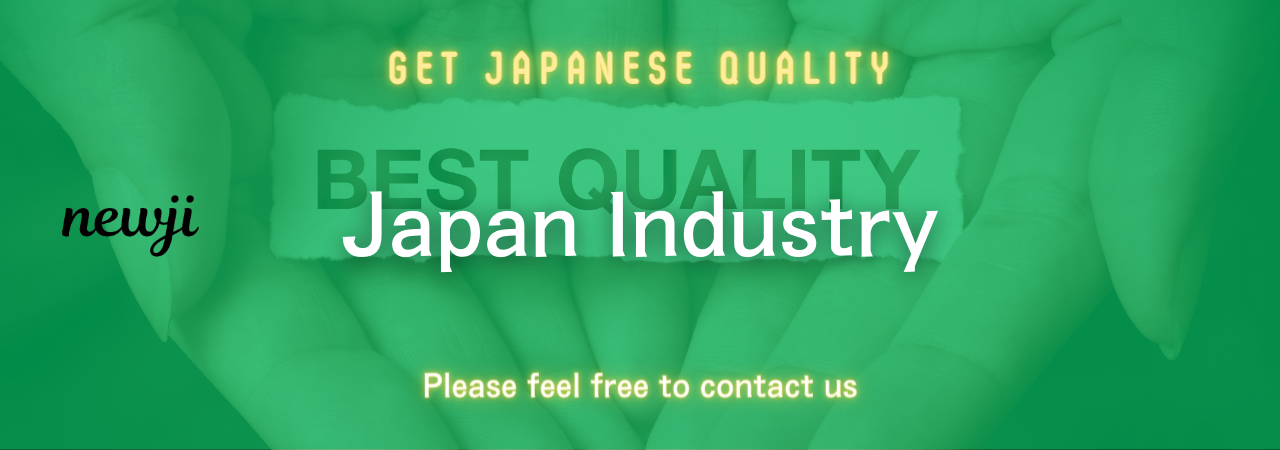
目次
Understanding Multivariate Analysis
Multivariate analysis is a powerful statistical tool used to understand patterns and relationships among several variables simultaneously.
It extends beyond simple analyses, such as univariate and bivariate methods, providing deeper insights by considering multiple variables together.
This approach is particularly useful when dealing with complex datasets, revealing hidden patterns and making informed predictions.
Multivariate analysis plays a critical role in various fields including finance, medicine, and social sciences.
Its key is to analyze multiple variables to determine how they interact with each other and how these interactions lead to certain outcomes.
Types of Multivariate Analysis
There are several types of multivariate analysis techniques, each serving different analytical purposes.
One common technique is Principal Component Analysis (PCA), which reduces dimensionality by transforming the original variables into a new set of variables called principal components.
PCA is primarily used to simplify the complexity of high-dimensional data while retaining its essential characteristics.
Another technique is Cluster Analysis, which groups a set of objects in such a way that objects in the same group (or cluster) are more similar to each other than those in other groups.
This method is frequently used in market segmentation and genetics.
Factor Analysis is another popular method, helping in identifying the underlying relationships between measured variables.
It reduces data to a lesser number of factors, aiding in simplifying the data’s structure.
Discriminant Analysis is used to classify a set of observations into predefined classes, predicting which group a new observation belongs to.
This is particularly useful in fields like customer segmentation and credit score modeling.
Applications of Multivariate Analysis in Data Analysis
Multivariate analysis is widely applied across different domains due to its robustness and ability to handle complexity in data.
In finance, it helps in risk management by analyzing and predicting financial markets, portfolio optimization, and credit scoring.
Traders and analysts use it to identify trends, correlations, and anomalies in financial data, aiding in better decision-making.
In the field of marketing, multivariate analysis assists companies in understanding consumer behavior.
Through segmentation, targeting, and customer profiling, businesses can tailor their strategies to different consumer groups, enhancing customer satisfaction and loyalty.
Healthcare is another field where multivariate analysis proves vital.
It is used to identify risk factors for diseases, understand symptoms correlations, and predict medical outcomes.
Analyzing patient data through multivariate methods helps in developing personalized treatments and improving patient care.
Additionally, in the realm of social sciences, researchers employ multivariate analysis to study complex phenomena such as social networks and behavioral patterns.
This can uncover relationships and predictors that are not apparent when evaluating data through simpler analyses.
Key Points for Effective Multivariate Analysis
To effectively conduct multivariate analysis, certain key points should be considered for optimal results.
First, it’s crucial to have a clear understanding of the dataset.
This includes knowing the type of variables present, their distribution, and any potential correlations that may exist.
Pre-processing the data is essential to handle missing values, outliers, and ensure that the data conforms to the assumptions of the chosen techniques.
This step often involves data cleaning, normalization, and transformation.
Selecting the appropriate multivariate technique is also critical.
This decision should be guided by the analysis objectives and the characteristics of the data.
For instance, if the goal is dimensionality reduction, PCA might be suitable; whereas for classification tasks, discriminant analysis may be ideal.
Model validation is a vital step that involves testing the model’s accuracy and reliability.
Techniques such as cross-validation help ensure the robustness of the analysis results.
Lastly, interpretation of the results is where insights are drawn from the analysis.
Understanding the output requires a good grasp of the method used and the domain expertise to make meaningful inferences.
Challenges in Multivariate Analysis
While multivariate analysis is incredibly useful, it also poses challenges that analysts and researchers must address.
The complexity of dealing with many variables can lead to issues such as multicollinearity, where two or more variables are highly correlated, potentially distorting the analysis results.
Careful diagnostic checks and advanced techniques can be leveraged to mitigate such problems.
Another challenge is ensuring the dataset is adequately large and representative for the analysis to be valid.
Insufficient or biased data can lead to inaccurate conclusions.
In some cases, the computational cost of performing multivariate analysis on large datasets can be significant.
This requires efficient algorithms and adequate computational resources to manage.
Finally, clear communication of results can be difficult due to the complex nature of multivariate analysis outputs.
Presenting findings in a way that is comprehensible to stakeholders who may not be familiar with statistical nuances requires thoughtful data visualization and narrative skills.
The Future of Multivariate Analysis
As the amount of data collected continues to grow exponentially, the importance of multivariate analysis will only increase.
With advancements in computational power and machine learning algorithms, the capacity to process more complex models is becoming more accessible.
Integrating artificial intelligence with multivariate techniques is likely to enhance predictive accuracy and uncover previously unknown patterns in data.
Automation of multivariate analysis processes is also becoming more prevalent, making these techniques more accessible to non-experts.
While challenges persist, the evolution and application of multivariate analysis in data analysis hold significant potential for further breakthroughs in understanding the complexities of the world through data.
This powerful approach will continue to evolve, providing valuable insights and driving informed decision-making across various sectors.
資料ダウンロード
QCD管理受発注クラウド「newji」は、受発注部門で必要なQCD管理全てを備えた、現場特化型兼クラウド型の今世紀最高の受発注管理システムとなります。
ユーザー登録
受発注業務の効率化だけでなく、システムを導入することで、コスト削減や製品・資材のステータス可視化のほか、属人化していた受発注情報の共有化による内部不正防止や統制にも役立ちます。
NEWJI DX
製造業に特化したデジタルトランスフォーメーション(DX)の実現を目指す請負開発型のコンサルティングサービスです。AI、iPaaS、および先端の技術を駆使して、製造プロセスの効率化、業務効率化、チームワーク強化、コスト削減、品質向上を実現します。このサービスは、製造業の課題を深く理解し、それに対する最適なデジタルソリューションを提供することで、企業が持続的な成長とイノベーションを達成できるようサポートします。
製造業ニュース解説
製造業、主に購買・調達部門にお勤めの方々に向けた情報を配信しております。
新任の方やベテランの方、管理職を対象とした幅広いコンテンツをご用意しております。
お問い合わせ
コストダウンが利益に直結する術だと理解していても、なかなか前に進めることができない状況。そんな時は、newjiのコストダウン自動化機能で大きく利益貢献しよう!
(β版非公開)