- お役立ち記事
- Fundamentals of neural networks and application to automatic inspection system technology using deep learning
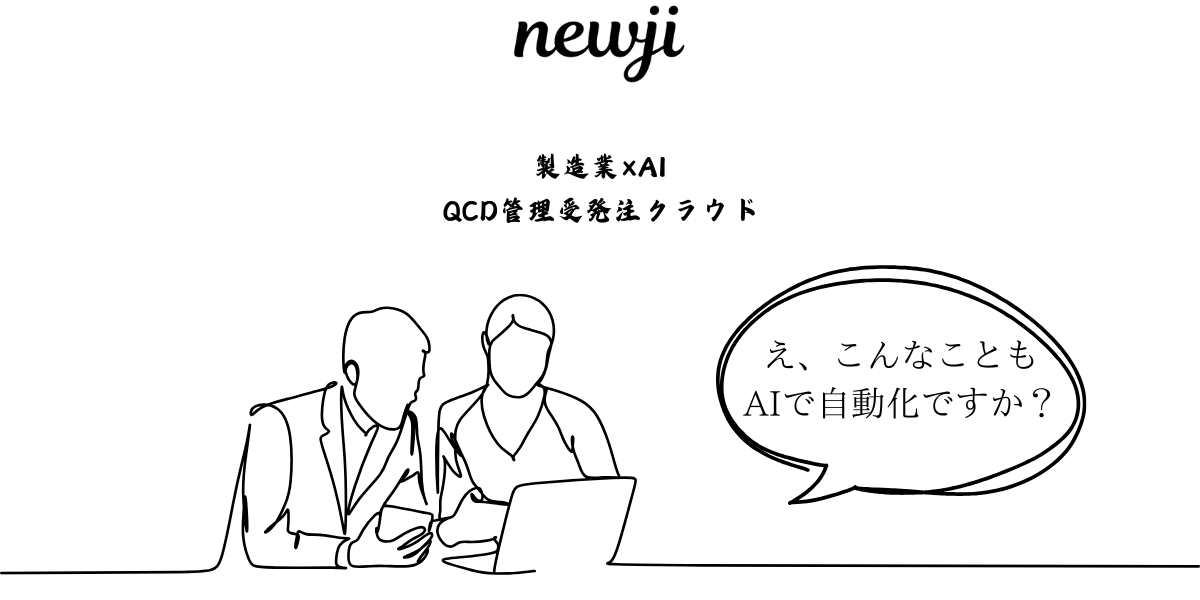
Fundamentals of neural networks and application to automatic inspection system technology using deep learning
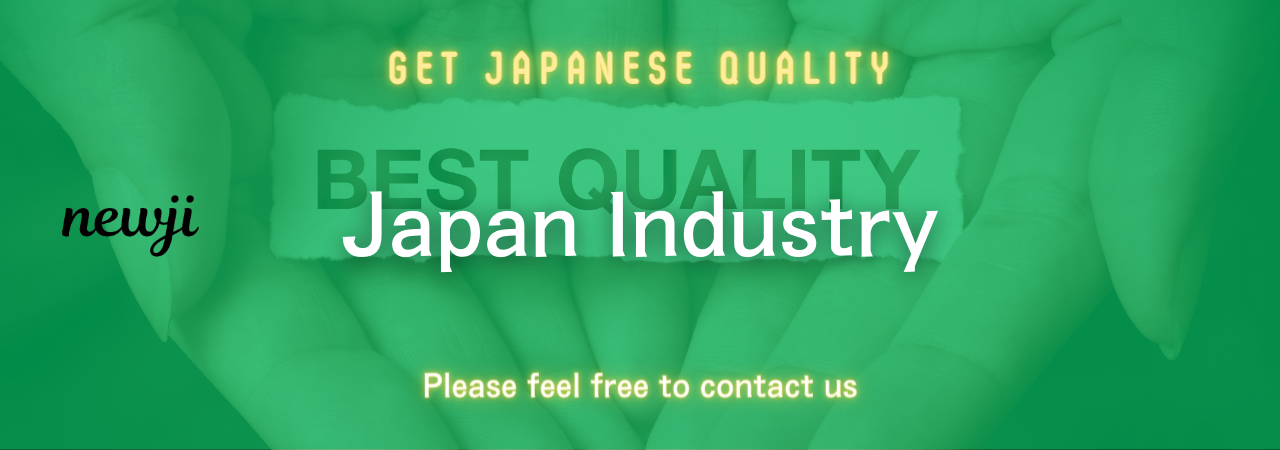
目次
Understanding Neural Networks
Neural networks are a fascinating aspect of artificial intelligence and form the foundation of many modern technologies.
They are computational models inspired by the human brain and consist of interconnected groups of artificial neurons.
These networks are designed to recognize patterns, classify data, and make decisions based on the information they have been trained on.
Structure of Neural Networks
At the core of neural networks are artificial neurons, also known as nodes or units.
These neurons are organized into layers: an input layer, one or more hidden layers, and an output layer.
Each neuron in a layer is connected to the neurons in the subsequent layer.
In the input layer, neurons receive data and pass it to the next layer.
The hidden layers process the data through weighted connections, where each connection has an associated weight.
These weights determine the strength and influence of the input data on the neurons.
Finally, the output layer produces the network’s prediction or classification.
Types of Neural Networks
There are several types of neural networks, each suited for different tasks.
One of the simplest types is the feedforward neural network, where data moves in a single direction from input to output without looping back.
They are primarily used for tasks such as image recognition and classification.
Another important type is the recurrent neural network (RNN).
RNNs are designed for sequential data, as they have connections that loop back, enabling them to retain information about previous inputs.
This makes them ideal for tasks like natural language processing and time-series analysis.
Convolutional neural networks (CNNs) are specialized for processing grid-like data structures, such as images.
They utilize convolutional layers to scan the input data and capture important features, making them highly effective in visual recognition tasks.
Deep Learning and Its Significance
Deep learning is a subset of machine learning that leverages neural networks with many layers.
These deep neural networks have dramatically improved the capability to analyze complex data patterns.
Benefits of Deep Learning
One of the primary benefits of deep learning is its ability to perform automatic feature extraction.
Unlike traditional machine learning algorithms, deep learning models do not require manual feature selection.
They automatically identify patterns and features that contribute to the desired output.
Deep learning models are highly scalable and can handle vast amounts of data.
As computing power and data availability have increased, these models have become more efficient and accurate.
Applications of Deep Learning
Deep learning has a wide range of applications across various industries.
In healthcare, it is used for medical image analysis, helping doctors diagnose diseases with high accuracy.
In the automotive industry, deep learning powers autonomous vehicles by enabling real-time object detection and decision-making.
In the entertainment industry, deep learning is used in recommendation systems to provide personalized content to users.
It is also used in speech recognition applications, translating spoken language into text efficiently.
Automatic Inspection Systems Using Deep Learning
One exciting application of neural networks and deep learning is in automatic inspection systems.
These systems are used to evaluate products for defects, ensuring quality control and compliance with industry standards.
How Automatic Inspection Systems Work
Automatic inspection systems utilize neural networks to analyze images or data from sensors.
The system is trained on a dataset containing both defective and non-defective samples.
During operation, the system analyzes the input data, typically in the form of images, to identify any anomalies or deviations from the norm.
These systems can detect minute flaws that might be missed by human inspectors.
Additionally, they offer faster processing times and can work around the clock without fatigue.
Industries Benefiting from These Systems
The manufacturing industry significantly benefits from automatic inspection systems.
For instance, in automotive manufacturing, these systems can inspect parts and components for defects, ensuring that only quality products reach the market.
In the electronics industry, automatic inspection systems examine circuit boards for minute defects that could affect performance.
Similarly, in food production, these systems ensure that products meet safety and quality standards by identifying foreign objects or contamination.
Challenges and Future Prospects
While automatic inspection systems using deep learning offer numerous advantages, they also present certain challenges.
One major challenge is the need for vast amounts of labeled data to train the system effectively.
Acquiring and annotating such data can be time-consuming and costly.
However, as research in deep learning continues to progress, novel methods such as transfer learning and unsupervised learning are being developed to address these challenges.
These methods reduce the dependency on large labeled datasets and improve the system’s adaptability to new inspection tasks.
The future of automatic inspection systems looks promising, with further advancements anticipated in accuracy and efficiency.
As deep learning technology continues to evolve, it holds the potential to revolutionize quality control processes across diverse sectors.
Conclusion
In summary, neural networks are a crucial technology in today’s world, offering powerful solutions for complex data analysis.
Deep learning, a progression of neural networks, is unlocking new possibilities and applications across industries.
With the integration of deep learning, automatic inspection systems are set to enhance manufacturing processes, ensuring higher quality and consistency.
As we continue to explore the capabilities of neural networks, they promise to drive innovation and efficiency in various domains, reshaping how we approach tasks that require precision and reliability.
資料ダウンロード
QCD調達購買管理クラウド「newji」は、調達購買部門で必要なQCD管理全てを備えた、現場特化型兼クラウド型の今世紀最高の購買管理システムとなります。
ユーザー登録
調達購買業務の効率化だけでなく、システムを導入することで、コスト削減や製品・資材のステータス可視化のほか、属人化していた購買情報の共有化による内部不正防止や統制にも役立ちます。
NEWJI DX
製造業に特化したデジタルトランスフォーメーション(DX)の実現を目指す請負開発型のコンサルティングサービスです。AI、iPaaS、および先端の技術を駆使して、製造プロセスの効率化、業務効率化、チームワーク強化、コスト削減、品質向上を実現します。このサービスは、製造業の課題を深く理解し、それに対する最適なデジタルソリューションを提供することで、企業が持続的な成長とイノベーションを達成できるようサポートします。
オンライン講座
製造業、主に購買・調達部門にお勤めの方々に向けた情報を配信しております。
新任の方やベテランの方、管理職を対象とした幅広いコンテンツをご用意しております。
お問い合わせ
コストダウンが利益に直結する術だと理解していても、なかなか前に進めることができない状況。そんな時は、newjiのコストダウン自動化機能で大きく利益貢献しよう!
(Β版非公開)