- お役立ち記事
- Fundamentals of physical reservoir computing and applications to data analysis and edge device/sensor implementation
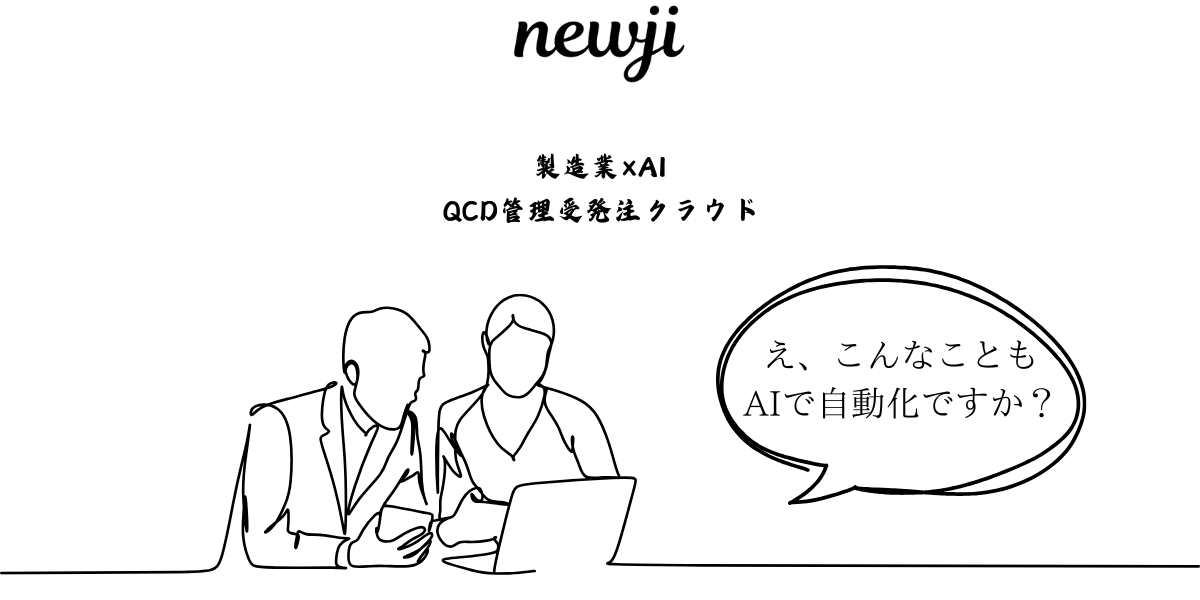
Fundamentals of physical reservoir computing and applications to data analysis and edge device/sensor implementation
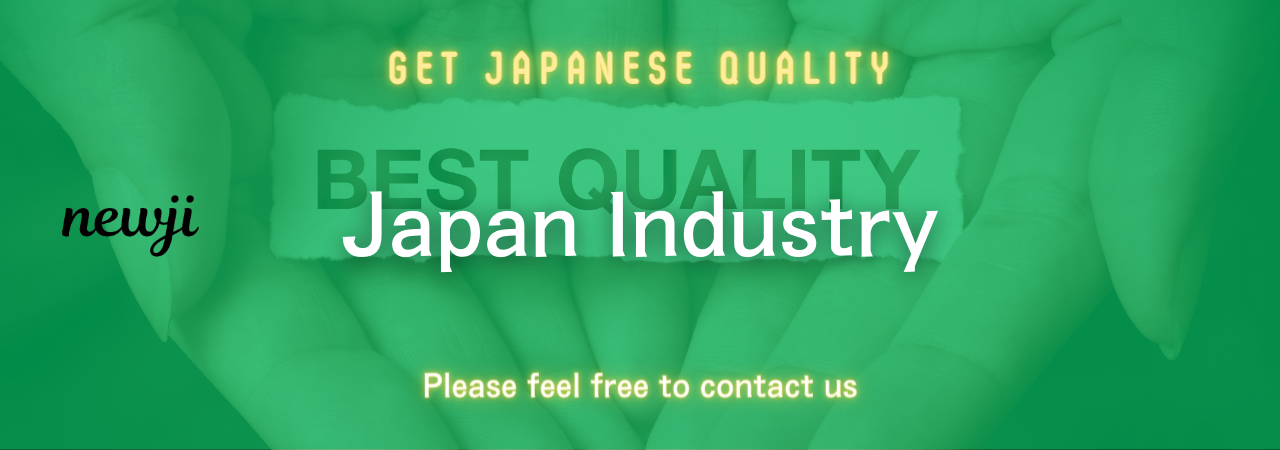
目次
Introduction to Physical Reservoir Computing
Physical reservoir computing is a fascinating area of study that bridges the gap between traditional computing and the dynamic systems found in nature.
This innovative approach leverages the natural dynamics of physical systems to perform computations in real-time.
The fundamental idea is to harness the intrinsic properties of a physical medium, which can process information non-linearly and memorize past inputs, making it suitable for complex data-processing tasks.
Reservoir computing emerged from the field of machine learning and neural networks.
Originally, it was designed to simplify training in recurrent neural networks by keeping the internal weights fixed and only training the output layer.
The “reservoir” in this context refers to a vast, dynamic system that transforms inputs into a high-dimensional representation.
This approach allows for efficient training and processing, which has profound implications for real-world applications.
How Physical Reservoir Computing Works
At its core, physical reservoir computing uses a physical system—like a fluid, optical, or mechanical medium—as the reservoir.
The input data are fed into this reservoir, which processes the data through its internal dynamics.
These dynamics naturally generate a rich set of features from the input data.
By observing the reservoir’s response, we can interpret complex patterns or solutions from the processed data.
The key advantage is that the reservoir itself performs powerful computations with minimal energy consumption.
After the reservoir has processed the data, a simple readout layer interprets the results.
This readout typically involves linear combinations of the features generated by the reservoir, making it computationally light.
The beauty of physical reservoir computing lies in its simplicity and the ability to make use of a wide variety of materials and systems as reservoirs.
Materials with complex time-dependent behaviors, such as certain chemicals, laser systems, and even biological substrates, have successfully been used to create reservoir computers.
The Role of Non-Linearity and Memory
Two pivotal features of a reservoir are its non-linearity and memory.
Non-linearity allows the system to handle complex, non-linear relationships within data, a task that linear systems struggle with.
Memory, or the reservoir’s ability to retain past input information, allows it to make temporal predictions.
This makes the system particularly useful for data streams that require context or history to make accurate predictions, such as speech recognition or dynamic system simulations.
Applications of Physical Reservoir Computing
The diverse applications of physical reservoir computing highlight its versatility in problem-solving across different industries.
Data Analysis
In data analysis, physical reservoir computing can be used to handle massive datasets with complex patterns.
Its efficiency in extracting non-linear relationships and temporal patterns makes it ideal for applications in financial data analysis, audio processing, and sensor data interpretation.
For instance, in financial markets, the ability to predict market trends relies heavily on understanding non-linear and often chaotic patterns.
Physical reservoir computing can provide real-time analysis and forecasting by leveraging historical data and integrating it with current market conditions.
Speech and Image Recognition
Another promising application is in the fields of speech and image recognition.
The complex patterns and contextual dependencies inherent in languages and visual data are well-suited to the strengths of reservoir computing.
Its capacity for real-time processing and pattern recognition allows for significant advancements in developing more responsive and adaptable recognition systems.
Edge Device and Sensor Implementation
Physical reservoir computing shines in edge device and sensor implementations.
These applications demand low-power, high-efficiency solutions capable of processing data locally rather than relying on centralized data centers.
Edge devices equipped with physical reservoir computing capabilities can perform complex data processing tasks directly on-device.
This enables faster decision-making, enhanced privacy, and reduced data transmission costs.
Applications range from smart home devices to autonomous vehicles, where real-time data processing is crucial for functionality and efficiency.
The Future of Physical Reservoir Computing
The future of physical reservoir computing is bright, with ongoing research exploring new materials and configurations to enhance its capabilities.
Emerging areas include the use of quantum systems and nanomaterials to create reservoirs that can process more complex computations with even greater efficiency.
Research is also focused on optimizing the scalability of reservoir computers so they can handle increasingly large and complex datasets.
By integrating breakthroughs from material science, machine learning, and computational theory, physical reservoir computing is poised to tackle some of the most challenging problems in data processing and edge computing.
Challenges and Considerations
Despite its potential, implementing physical reservoir computing brings challenges that researchers and developers must address.
Selecting the right physical medium is crucial to the reservoir’s performance and applicability.
Factors such as the system’s stability, ease of integration, and the ability to scale need careful consideration.
Moreover, interpreting the results from the reservoir requires robust methodologies, ensuring that the readout layer is capable of accurately extracting useful information.
Finally, as with any emerging technology, standardization and establishing protocols for integration into existing systems remain key hurdles to widespread adoption.
Conclusion
Physical reservoir computing offers an exciting avenue for advanced data processing solutions, combining the advantages of natural dynamic systems with modern computational needs.
Its promise in data analysis and edge device implementation signals a shift towards more integrated, efficient, and adaptive technologies.
As research and development continue to push the boundaries of what’s possible, the potential applications and implications of this innovative computing paradigm are limited only by imagination.
資料ダウンロード
QCD調達購買管理クラウド「newji」は、調達購買部門で必要なQCD管理全てを備えた、現場特化型兼クラウド型の今世紀最高の購買管理システムとなります。
ユーザー登録
調達購買業務の効率化だけでなく、システムを導入することで、コスト削減や製品・資材のステータス可視化のほか、属人化していた購買情報の共有化による内部不正防止や統制にも役立ちます。
NEWJI DX
製造業に特化したデジタルトランスフォーメーション(DX)の実現を目指す請負開発型のコンサルティングサービスです。AI、iPaaS、および先端の技術を駆使して、製造プロセスの効率化、業務効率化、チームワーク強化、コスト削減、品質向上を実現します。このサービスは、製造業の課題を深く理解し、それに対する最適なデジタルソリューションを提供することで、企業が持続的な成長とイノベーションを達成できるようサポートします。
オンライン講座
製造業、主に購買・調達部門にお勤めの方々に向けた情報を配信しております。
新任の方やベテランの方、管理職を対象とした幅広いコンテンツをご用意しております。
お問い合わせ
コストダウンが利益に直結する術だと理解していても、なかなか前に進めることができない状況。そんな時は、newjiのコストダウン自動化機能で大きく利益貢献しよう!
(Β版非公開)