- お役立ち記事
- Fundamentals of real-world sensor data processing and application to action recognition and indoor position estimation
月間76,176名の
製造業ご担当者様が閲覧しています*
*2025年3月31日現在のGoogle Analyticsのデータより
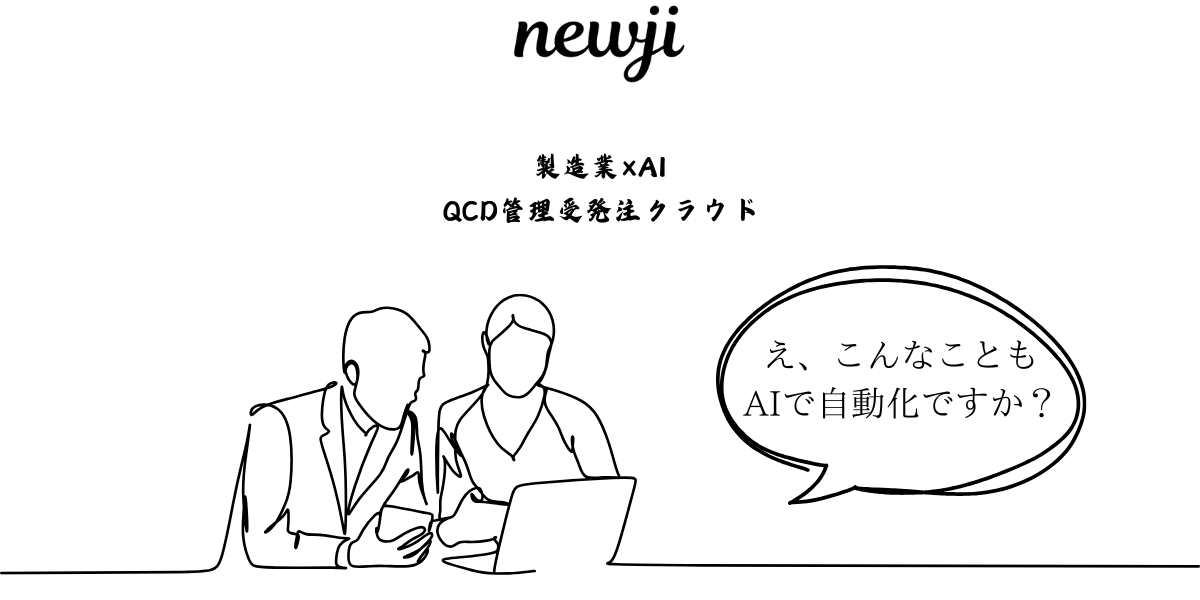
Fundamentals of real-world sensor data processing and application to action recognition and indoor position estimation
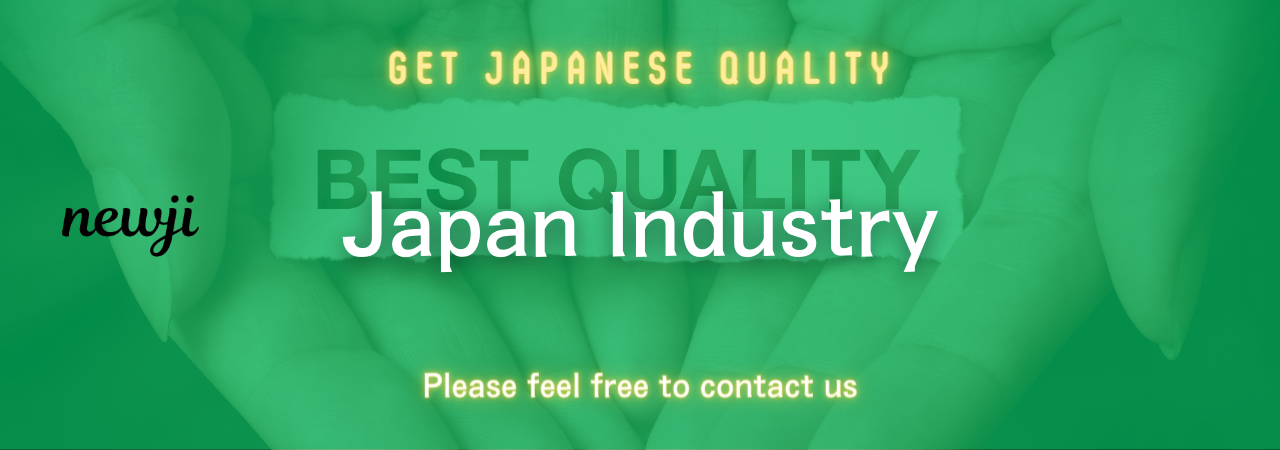
目次
Introduction to Real-World Sensor Data Processing
In today’s technologically advanced world, sensor data plays a crucial role in various applications, ranging from everyday consumer devices to specialized industrial systems.
Real-world sensor data processing involves collecting, cleaning, interpreting, and utilizing data captured by sensors.
These sensors are omnipresent, embedded in smartphones, smartwatches, fitness trackers, and an array of IoT devices that continuously send streams of data.
Sensor data processing is foundational for both action recognition and indoor position estimation.
Action recognition involves identifying specific activities performed by individuals, while indoor position estimation deals with determining a person’s or object’s location inside a building.
Both applications demand precise and efficient data processing to deliver reliable results.
Understanding the Basics of Sensor Data Collection
The first step in sensor data processing is data collection.
Various types of sensors, such as accelerometers, gyroscopes, magnetometers, and barometers, are utilized to capture different data points.
These sensors provide vital raw data that can be further processed for meaningful applications.
Acceleration sensors, or accelerometers, measure the rate of change of velocity, indicating movements made by a device.
Gyroscopes track orientation and angular velocity, offering insights into the direction of movements.
Magnetometers detect magnetic fields and help determine orientation by measuring the direction relative to the Earth’s magnetic field.
Barometers gauge air pressure, which can be used to estimate altitude changes.
Ensuring Data Quality and Cleaning
Once sensor data is collected, the next step is to ensure its quality.
Raw sensor data often contains noise, errors, and inconsistencies that must be addressed through data cleaning.
Noise in the data can result from environmental factors, sensor malfunctions, or transmission errors.
Effective data cleaning involves filtering out noise, correcting errors, and aligning data from multiple sensors.
This process requires sophisticated algorithms to differentiate between useful data and anomalies.
Filtering techniques like Kalman filters and moving averages are commonly employed to smooth the data.
Preprocessing for Improved Accuracy
Preprocessing involves transforming raw sensor data into a format suitable for analysis.
This includes normalization, feature extraction, and dimensionality reduction.
Normalization ensures that data is on the same scale, preventing any single sensor’s data from disproportionately influencing the results.
Feature extraction identifies relevant data characteristics to simplify the analysis process.
For instance, in action recognition, features such as mean, variance, and frequency components of sensor signals are extracted.
Dimensionality reduction techniques, like Principal Component Analysis (PCA), help in reducing data size while preserving essential features.
Applications in Action Recognition
After preprocessing, sensor data is primed for analysis and application in various domains, notably action recognition.
Action recognition systems use algorithms to interpret the processed data and classify different human activities, such as walking, running, sitting, or standing.
Machine learning models, particularly neural networks and decision trees, are widely utilized to enhance the accuracy of action recognition systems.
These models learn from labeled training data to distinguish between different activities.
The success of action recognition not only improves fitness monitoring applications but also enhances healthcare technologies by monitoring patient activities and facilitating independent living for the elderly.
Challenges in Action Recognition
Although action recognition offers many benefits, it presents challenges as well.
Complex and overlapping activities, variations in individual movement styles, and device placement all affect recognition accuracy.
Robust models need to be adaptive, capturing intricate patterns and acknowledging variations to minimize false detections.
Developing adaptable and generalizable models is key to refining action recognition systems.
Continued research and development in this field aim to overcome these challenges and expand the applications of action recognition in daily life.
Indoor Position Estimation Using Sensor Data
Another significant application of sensor data processing is indoor position estimation.
This area focuses on determining the location of people or objects within buildings, where GPS is often unreliable.
Indoor positioning is crucial for diverse applications including navigation in complex environments, asset tracking, and enhancing user experiences in smart buildings.
It employs techniques such as dead reckoning, trilateration, and fingerprinting using data from embedded sensors to estimate positions accurately.
Techniques for Indoor Position Estimation
Dead reckoning involves using data from accelerometers, gyroscopes, and magnetometers to calculate position changes from a known starting point.
By continuously updating position estimates based on movement data, dead reckoning can provide accurate and rea-time positioning inside buildings.
Trilateration uses the distance from multiple known reference points, determined through signal strength or time of flight, to estimate locations.
This method often requires integration with WiFi, Bluetooth, or ultra-wideband technologies for enhanced precision.
Fingerprinting involves creating a map of signal patterns, like WiFi signal strength, within a building.
By matching real-time sensor data against the map, the location of a device can be inferred.
Challenges and Innovations in Indoor Positioning
Indoor positioning systems face challenges, including signal interference, environmental changes, and the need for high accuracy.
Variations in building architecture and materials can significantly impact system performance, necessitating advanced algorithms for continuous adaptation.
Innovations in machine learning and data fusion are paving the way for more reliable indoor positioning solutions.
By combining data from multiple sensors and learning from diverse environments, these systems become more resilient to inconsistencies and deliver enhanced positioning accuracy.
Conclusion
The fundamentals of real-world sensor data processing extend into multifaceted applications in action recognition and indoor position estimation.
From collecting raw data through a vast array of sensors to refining that data into practical insights, each step is crucial for effective implementation.
Despite the challenges inherent in both action recognition and indoor positioning, advancements in technology and methodologies continue to improve their reliability and application scope.
These systems play vital roles in daily life, enhancing health monitoring, personal navigation, and automation in smart environments.
As sensor technology and data processing techniques evolve, we can anticipate even greater innovations and applications emerging from the foundation laid by sensor data processing.
資料ダウンロード
QCD管理受発注クラウド「newji」は、受発注部門で必要なQCD管理全てを備えた、現場特化型兼クラウド型の今世紀最高の受発注管理システムとなります。
ユーザー登録
受発注業務の効率化だけでなく、システムを導入することで、コスト削減や製品・資材のステータス可視化のほか、属人化していた受発注情報の共有化による内部不正防止や統制にも役立ちます。
NEWJI DX
製造業に特化したデジタルトランスフォーメーション(DX)の実現を目指す請負開発型のコンサルティングサービスです。AI、iPaaS、および先端の技術を駆使して、製造プロセスの効率化、業務効率化、チームワーク強化、コスト削減、品質向上を実現します。このサービスは、製造業の課題を深く理解し、それに対する最適なデジタルソリューションを提供することで、企業が持続的な成長とイノベーションを達成できるようサポートします。
製造業ニュース解説
製造業、主に購買・調達部門にお勤めの方々に向けた情報を配信しております。
新任の方やベテランの方、管理職を対象とした幅広いコンテンツをご用意しております。
お問い合わせ
コストダウンが利益に直結する術だと理解していても、なかなか前に進めることができない状況。そんな時は、newjiのコストダウン自動化機能で大きく利益貢献しよう!
(β版非公開)