- お役立ち記事
- Fundamentals of reservoir computing and points of application to systems
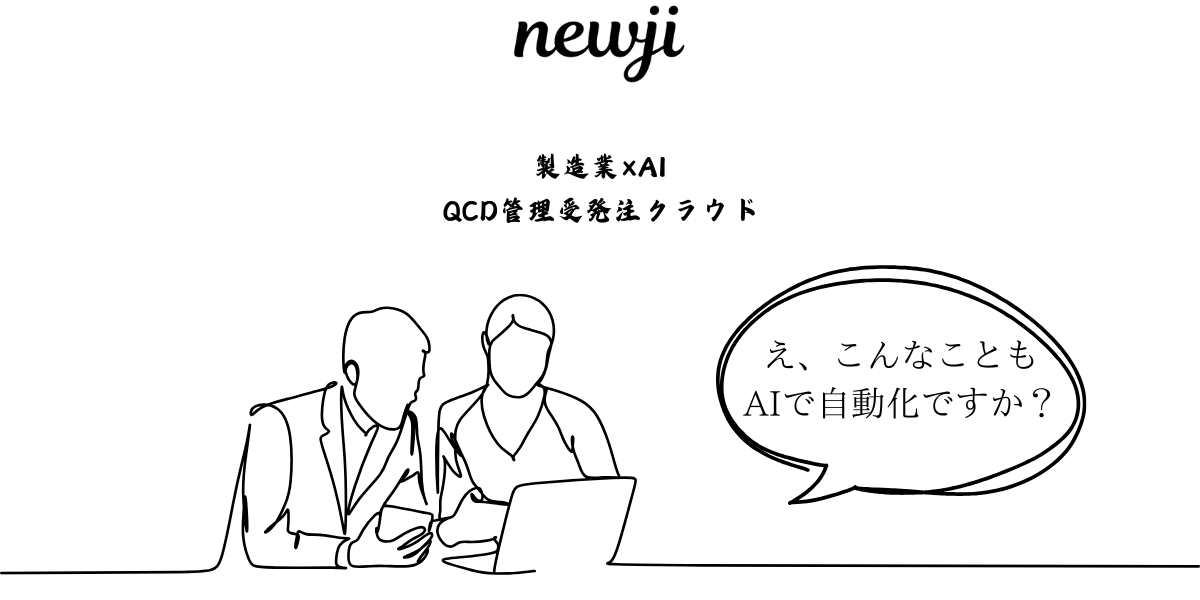
Fundamentals of reservoir computing and points of application to systems
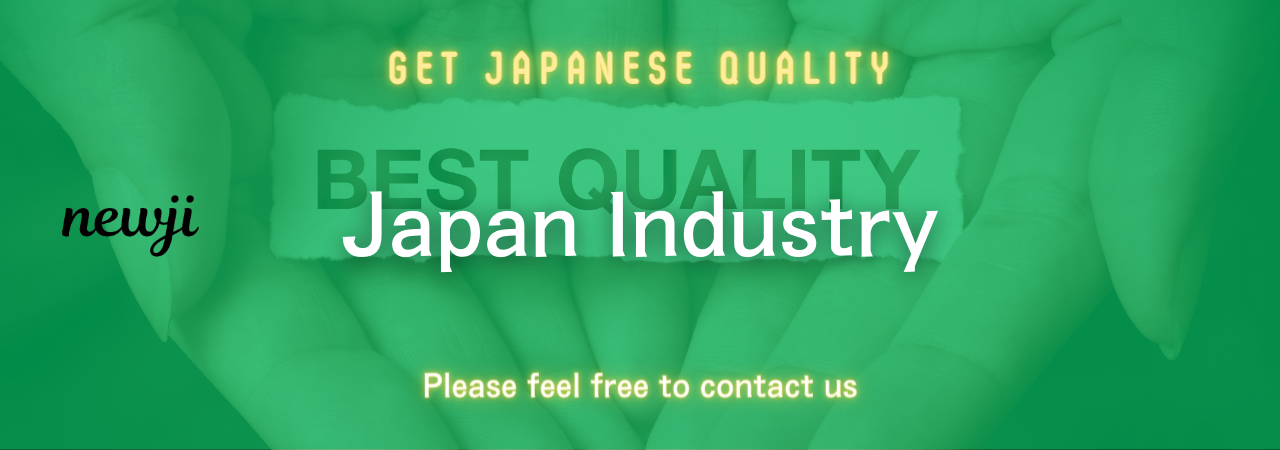
目次
Understanding the Basics of Reservoir Computing
Reservoir computing is an innovative and fascinating branch of machine learning that has been gaining popularity due to its unique approach to processing temporal data.
It works by employing a fixed, dynamic reservoir, which is unlike the traditional neural networks that require significant training and adjustment of parameters.
This paradigm mimics the recurrent neural network’s architecture but simplifies the learning process, thereby offering computational efficiency and flexibility.
At its core, reservoir computing utilizes a network called the ‘reservoir’ that is made up of recurrent connections.
These connections generate a diverse set of temporal dynamics, which can represent complex data patterns.
The reservoir, filled with randomly initialized nodes and weights, remains unchanged during the training phase.
This aspect is what primarily differentiates it from other neural network models that require backpropagation throughout the network.
Data inputs are fed into the reservoir, which transforms them into a higher dimensionality through its dynamic activities.
This transformation enables the extraction of features that would be difficult to delineate using ordinary methods.
Subsequently, only the final layer, known as the readout layer, needs to be trained.
This layer is tasked with interpreting the dynamic patterns created by the reservoir to provide useful output or predictions.
Key Advantages of Reservoir Computing
One of the main advantages of reservoir computing is its simplicity and ease of implementation.
Since only the output layer is trained, the computational cost is significantly reduced compared to traditional methods.
This makes it an attractive option for tasks that involve real-time processing or require low-power calculations.
Another considerable advantage is its robustness to noise and perturbations in data.
Because the reservoir computing model deals with the temporal relationship and patterns rather than individual data points, it maintains a high level of prediction accuracy even in the presence of noisy inputs.
Furthermore, reservoir computing can model complex chaotic or time-varying systems without requiring a detailed understanding of the system’s dynamics.
This characteristic allows for greater flexibility and adaptability in diverse applications ranging from weather forecasting to speech recognition.
Applications of Reservoir Computing in Various Systems
Signal Processing
Reservoir computing has shown promising results in the field of signal processing, particularly for tasks involving time-series data.
For instance, it can be employed in the real-time analysis of financial data where stock prices and market trends need to be predicted.
The ability to handle noisy inputs and process data efficiently makes reservoir computing ideal for these financial forecasting tasks.
Similarly, it finds application in audio signal processing where it aids in improving speech recognition systems.
By converting speech signals into meaningful patterns, reservoir computing can enhance the accuracy of voice-activated devices and transcription services.
Control Systems
The integration of reservoir computing in control systems is another area of interest.
Due to its strength in managing dynamic systems, it can efficiently model and predict the behavior of complex control systems.
For example, it can improve the performance of robotic systems by providing precise control commands adapted to real-time conditions, enabling smoother operations in varying environments.
In addition, reservoir computing can support automotive control systems, contributing to the development of advanced driver-assistance systems (ADAS) and autonomous vehicles.
By anticipating changes in driving conditions, these systems can better predict and adapt to ensure safer navigation.
Energy Management
In the context of energy management, reservoir computing offers capabilities for optimizing the distribution and consumption of energy resources.
It can be integrated into smart grid systems to predict electricity demand and supply imbalances, thus helping in the efficient allocation of resources and reducing wastage.
Furthermore, it can assist in renewable energy management where weather conditions significantly impact energy generation.
By forecasting solar or wind energy potential, reservoir computing enables more reliable integration of renewable energy sources into the power grid.
Biomedical Field
Reservoir computing is also making strides in the biomedical field, particularly in modeling neural information processing.
It provides insights into brain signal dynamics, which can aid in the development of advanced prosthetics and brain-computer interfaces (BCIs).
Additionally, it offers potential in predictive diagnostics by analyzing physiological signals to identify early signs of health conditions.
Such applications could lead to innovative diagnostic tools that improve patient care by providing timely and accurate health assessments.
Challenges and Future Directions
Despite its advantages, reservoir computing is not without challenges.
Determining an optimal reservoir size and architecture can be difficult and often requires empirical testing.
Moreover, while the random initialization of weights provides diversity, it may also lead to variability in performance.
Future research aims to address these challenges by exploring adaptive reservoirs and advanced training algorithms.
One area of potential is the development of biologically inspired reservoirs that mimic human brain functioning more closely, which may enhance its capacity to learn and adapt.
Additionally, expanding the integration of reservoir computing with other machine learning techniques, such as deep learning, could open new avenues for improved efficiency and performance.
As our understanding continues to advance, reservoir computing is poised to play an integral role in the ever-evolving landscape of artificial intelligence and computational neuroscience.
By focusing on both theoretical refinement and practical application, the field promises to contribute significantly to the development of intelligent, adaptive systems that can meet the complex challenges of tomorrow.
資料ダウンロード
QCD調達購買管理クラウド「newji」は、調達購買部門で必要なQCD管理全てを備えた、現場特化型兼クラウド型の今世紀最高の購買管理システムとなります。
ユーザー登録
調達購買業務の効率化だけでなく、システムを導入することで、コスト削減や製品・資材のステータス可視化のほか、属人化していた購買情報の共有化による内部不正防止や統制にも役立ちます。
NEWJI DX
製造業に特化したデジタルトランスフォーメーション(DX)の実現を目指す請負開発型のコンサルティングサービスです。AI、iPaaS、および先端の技術を駆使して、製造プロセスの効率化、業務効率化、チームワーク強化、コスト削減、品質向上を実現します。このサービスは、製造業の課題を深く理解し、それに対する最適なデジタルソリューションを提供することで、企業が持続的な成長とイノベーションを達成できるようサポートします。
オンライン講座
製造業、主に購買・調達部門にお勤めの方々に向けた情報を配信しております。
新任の方やベテランの方、管理職を対象とした幅広いコンテンツをご用意しております。
お問い合わせ
コストダウンが利益に直結する術だと理解していても、なかなか前に進めることができない状況。そんな時は、newjiのコストダウン自動化機能で大きく利益貢献しよう!
(Β版非公開)