- お役立ち記事
- Fundamentals of sensor data processing technology and application to anomaly detection and prediction systems
月間76,176名の
製造業ご担当者様が閲覧しています*
*2025年3月31日現在のGoogle Analyticsのデータより
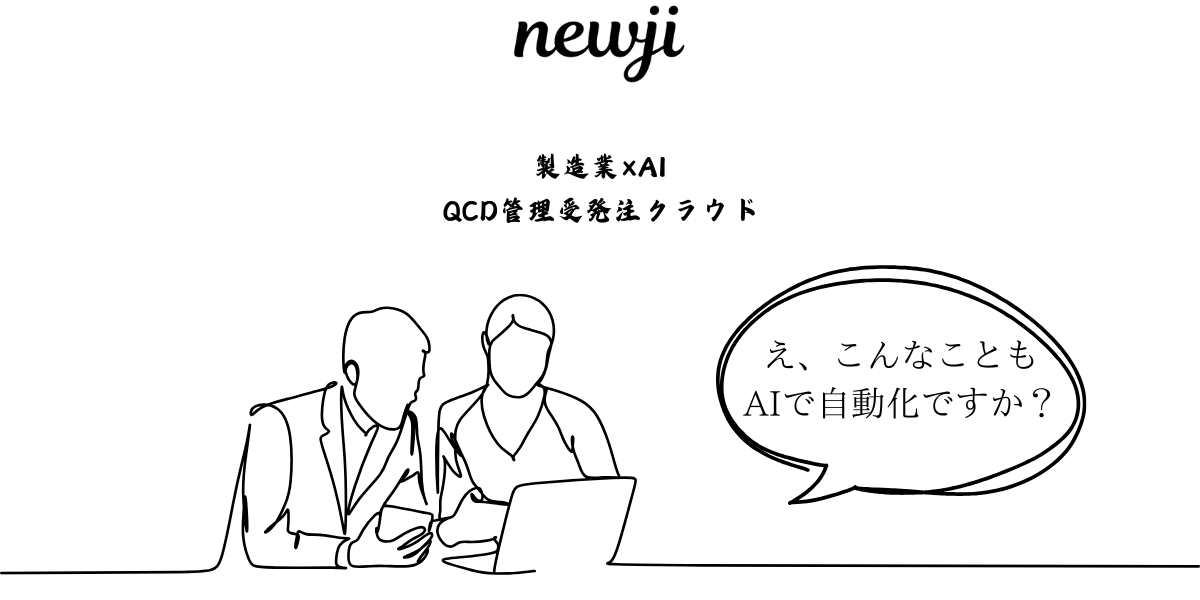
Fundamentals of sensor data processing technology and application to anomaly detection and prediction systems
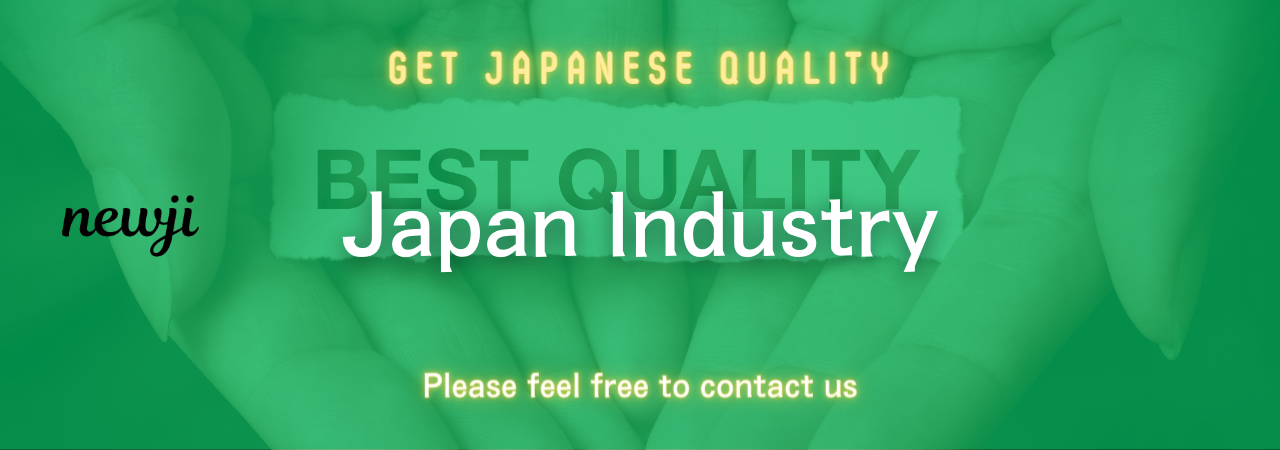
目次
Introduction to Sensor Data Processing
Sensor data processing plays a crucial role in modern technology, enabling various applications such as anomaly detection and predictive maintenance systems.
With the explosion of IoT devices and the vast array of sensors integrated into these systems, processing the collected data efficiently and accurately has become essential.
Understanding the fundamentals of sensor data processing is key to harnessing its potential in various industries.
What is Sensor Data?
Sensors are devices that detect and respond to changes in the environment and convert this information into data that can be analyzed.
Data sourced from sensors can range from temperature and humidity readings to motion and pressure measurements, depending on the sensor type.
Sensor data often forms the backbone of data-driven decision-making processes and helps automate many tasks in today’s smart systems.
Importance of Sensor Data Processing
Effective processing of sensor data allows organizations to gain insights that can lead to improved operational efficiency and decision-making.
By transforming raw data into actionable insights, businesses can enhance productivity and optimize processes.
Moreover, it paves the way for advanced applications like anomaly detection and predictive systems, which rely heavily on accurate data analysis.
Steps in Sensor Data Processing
Sensor data processing typically involves several sequential steps, each contributing to cleaning, interpreting, and analyzing data for better decision-making.
1. Data Collection
Data collection is the initial stage where sensor data is gathered from different sources.
It’s crucial to choose the appropriate sensors and ensure that they are properly calibrated to collect accurate data.
Common methods for data collection include wireless sensor networks and connected devices, enabling real-time streaming and storage for further processing.
2. Data Preprocessing
In this stage, raw data is cleaned and organized to prepare it for analysis.
Preprocessing involves filtering noise, handling missing values, and normalizing data for consistency.
The aim is to ensure the data is in a suitable format and quality, which is essential for accurate analysis.
3. Data Integration
Sensor data may be sourced from multiple devices or sensors.
Integrating this data into a single cohesive format is imperative to maintain data integrity.
Data integration involves combining datasets, correcting inconsistencies, and ensuring that data from different sensors is synchronized.
4. Data Analysis
Once the data is preprocessed and integrated, it’s ready for analysis.
Data analysis includes statistical methods and machine learning algorithms to extract patterns and insights.
Advanced analytic techniques like regression analysis, clustering, and time-series analysis can be employed to understand trends and anomalies.
Anomaly Detection with Sensor Data
Anomaly detection is a critical application of sensor data processing, which involves identifying unusual patterns that deviate from the expected norm.
It is particularly useful in areas like fault detection, quality assurance, and fraud prevention.
1. Types of Anomalies
Anomalies can be categorized into three types: point anomalies, contextual anomalies, and collective anomalies.
Point anomalies refer to individual data points that differ significantly from the rest of the data.
Contextual anomalies are context-specific, meaning the data may appear normal unless correlated with a specific context.
Collective anomalies are a group of data points that together represent an abnormal pattern.
2. Anomaly Detection Techniques
Several techniques can be used to detect anomalies in sensor data, each with its own strengths and weaknesses.
Common techniques include statistical methods such as Z-score analysis, machine learning approaches like isolation forests and clustering-based detection, and deep learning models that leverage neural networks.
Selecting the right technique often depends on the specifics of the dataset and the type of anomaly expected.
Prediction Systems Using Sensor Data
Predictive systems use sensor data to forecast future events and conditions, assisting in planning and proactive decision-making.
By analyzing historical data, these systems can predict trends and potential issues before they arise.
1. Use Cases of Predictive Systems
Predictive maintenance is a prominent use case, where sensor data helps predict when equipment is likely to fail, allowing for timely maintenance, reducing downtime and maintenance costs.
In the health sector, predictive systems can monitor patient vitals to anticipate health issues.
Smart cities utilize predictive systems to predict traffic patterns and manage resources efficiently.
2. Predictive Modeling Techniques
Predictive models often use machine learning algorithms to improve prediction accuracy.
Regression models, decision trees, and neural networks are popular choices for building predictive systems.
The choice of model depends on factors like data volume, complexity, and the specific prediction task.
Challenges in Sensor Data Processing
Despite its benefits, sensor data processing presents several challenges, such as data quality, storage concerns, and security issues.
Ensuring data accuracy is critical, as poor quality data can lead to incorrect predictions or decisions.
With the growing number of sensors, storage and management of vast data volumes are significant concerns that need robust solutions.
Moreover, securing sensor data from unauthorized access and breaches is a top priority.
Conclusion
Sensor data processing forms the foundation of innovative applications that drive efficiency and intelligence in today’s tech-driven world.
Understanding its fundamentals and addressing its challenges will empower industries to fully exploit the potentials of sensors.
As technology continues to advance, sensor data processing will remain integral to the development of smarter, more reliable systems.
資料ダウンロード
QCD管理受発注クラウド「newji」は、受発注部門で必要なQCD管理全てを備えた、現場特化型兼クラウド型の今世紀最高の受発注管理システムとなります。
ユーザー登録
受発注業務の効率化だけでなく、システムを導入することで、コスト削減や製品・資材のステータス可視化のほか、属人化していた受発注情報の共有化による内部不正防止や統制にも役立ちます。
NEWJI DX
製造業に特化したデジタルトランスフォーメーション(DX)の実現を目指す請負開発型のコンサルティングサービスです。AI、iPaaS、および先端の技術を駆使して、製造プロセスの効率化、業務効率化、チームワーク強化、コスト削減、品質向上を実現します。このサービスは、製造業の課題を深く理解し、それに対する最適なデジタルソリューションを提供することで、企業が持続的な成長とイノベーションを達成できるようサポートします。
製造業ニュース解説
製造業、主に購買・調達部門にお勤めの方々に向けた情報を配信しております。
新任の方やベテランの方、管理職を対象とした幅広いコンテンツをご用意しております。
お問い合わせ
コストダウンが利益に直結する術だと理解していても、なかなか前に進めることができない状況。そんな時は、newjiのコストダウン自動化機能で大きく利益貢献しよう!
(β版非公開)