- お役立ち記事
- Fundamentals of sensor fusion
月間76,176名の
製造業ご担当者様が閲覧しています*
*2025年3月31日現在のGoogle Analyticsのデータより
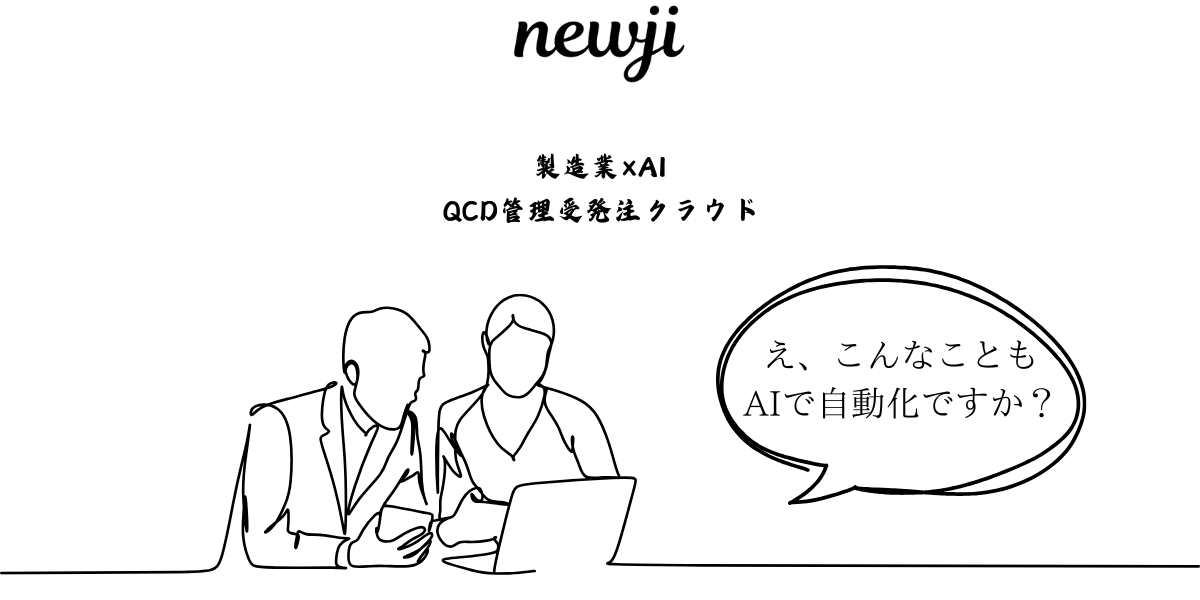
Fundamentals of sensor fusion
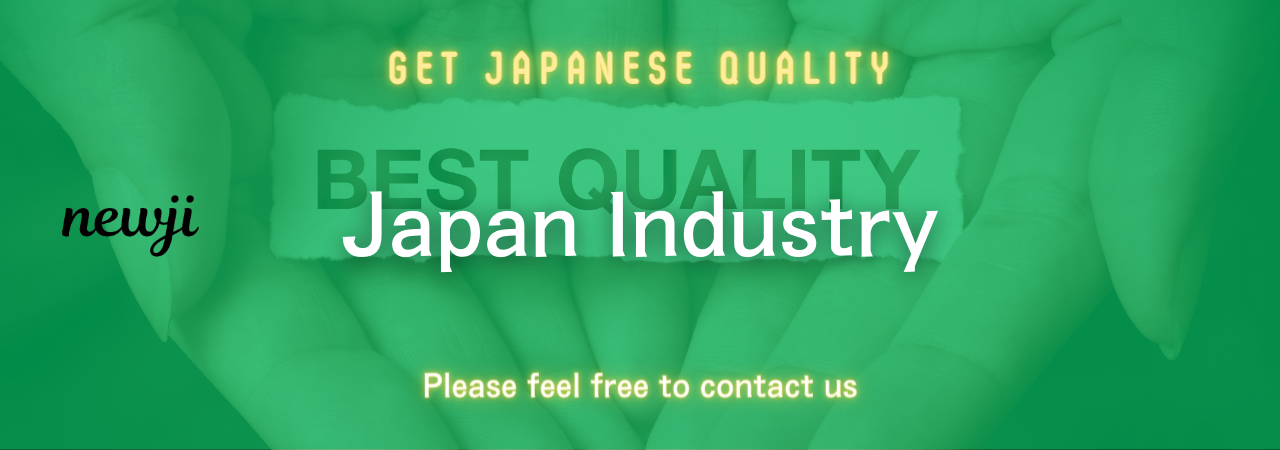
Understanding Sensor Fusion
Sensor fusion is an intriguing concept that forms the backbone of many advanced technologies we encounter today.
In its simplest form, sensor fusion refers to the process of combining data from multiple sensors to produce more accurate, reliable, or comprehensive information than could be obtained from a single sensor.
This is a crucial technique in various applications including autonomous vehicles, robotics, and even smartphones.
Why Sensor Fusion is Important
Sensors, by their nature, provide limited views of an environment or condition.
Each sensor might have its strengths and weaknesses based on its design, its position, and environmental factors.
For instance, a camera might offer rich visual information but could struggle in low-light conditions.
An infrared sensor, on the other hand, could provide useful data in those same scenarios.
By fusing information from both types of sensors, we gain a more rounded understanding of the situation.
The importance of sensor fusion also lies in its ability to create redundancy.
If one sensor fails or provides faulty data, the system can rely on information from other sensors.
This leads to more robust and resilient applications, which are quintessential in critical situations such as autonomous driving or medical diagnostics.
Basic Concepts of Sensor Fusion
At its core, sensor fusion involves three key concepts: data association, data filtering, and data integration.
– **Data Association:** This involves aligning the data from different sensors.
Different sensors have varied scales and units of measurement.
Data association ensures that data points from each sensor are properly correlated with one another.
– **Data Filtering:** Sensor data often contains noise, which could be due to environmental conditions, sensor quality, or other factors.
Filtering processes, like the Kalman filter, are often used to clean the data, ensuring the information used in fusion is accurate.
– **Data Integration:** This is the actual combining of data from the various sensors.
The integrated data provides a more detailed and informative result than any single sensor output by itself.
Applications of Sensor Fusion
Sensor fusion is used in a wide array of fields and industries.
Understanding some of its key applications can help us appreciate its ubiquitous nature.
– **Autonomous Vehicles:** Self-driving cars rely heavily on sensor fusion.
Cameras, radars, ultrasonic sensors, and LiDARs provide varied data inputs that need to be integrated to understand the vehicle’s environment and make safe decisions.
For example, a camera might detect obstacles visually, while a radar measures their distance using radio waves.
– **Robotics:** In robotics, sensor fusion is crucial for navigation and interaction with environments.
A robot might use multiple sensors like gyroscopes, accelerometers, and cameras for spatial awareness.
This helps robots perform tasks such as picking and placing objects or navigating complex terrains.
– **Smartphones:** Mobile phones utilize sensor fusion for features like augmented reality and fitness tracking.
Gyroscopes, accelerometers, and GPS data are combined to enhance user experience and deliver precise information.
– **Healthcare:** In medical devices, sensor fusion can help monitor vital signs more accurately.
Data from heart rate monitors, temperature sensors, and motion sensors can be integrated to provide a comprehensive health profile of patients.
Methods and Techniques in Sensor Fusion
There are several methods and techniques used in sensor fusion to achieve optimal results.
– **Weighted Averaging:** This is one of the simplest methods where different data inputs are averaged, assigning more weight to the more reliable or accurate sensors.
– **Bayesian Inference:** This method uses probability distributions to combine data.
It updates the probability estimate for a state as more information becomes available.
Bayesian methods are powerful in situations where sensor data correlation is probabilistic.
– **Kalman Filters:** Widely used for systems that are partially observable, Kalman filters provide estimates of unknown variables by predicting a series of measurements over time.
They are particularly useful in applications like navigation and tracking.
– **Particle Filters:** These are more flexible than Kalman filters, especially in non-linear systems.
They use a set of particles, each representing a possible state, and update these hypotheses with the arrival of new data.
Challenges in Sensor Fusion
While the benefits of sensor fusion are significant, several challenges must be addressed to fully capitalize on this technology.
– **Data Synchronization:** Different sensors may transmit data at different rates or intervals, leading to synchronization issues that can affect the accuracy of the fused data.
– **Computational Demand:** Processing and integrating large volumes of data from multiple sensors require substantial computational power, which can be challenging for devices with limited resources.
– **Sensor Calibration:** Ensuring that all sensors provide accurate data requires regular calibration.
This can become complex, especially in dynamic environments where conditions change frequently.
– **Handling Uncertainty:** Sensor fusion must account for uncertainties in sensor measurements.
Developing robust algorithms that can handle these uncertainties is crucial for reliable fusion outcomes.
The Future of Sensor Fusion
As technology continues to advance, the role of sensor fusion is set to expand even further.
With the growth of Internet of Things (IoT) devices, the demand for integrating data from diverse sources is becoming more prevalent.
Future advancements in artificial intelligence and machine learning may lead to more sophisticated sensor fusion techniques, enabling smarter applications and systems.
In essence, sensor fusion will continue to play a vital role in the development of new technologies and improvements in existing ones.
By overcoming its current challenges and embracing innovation, sensor fusion holds the potential to transform many aspects of daily life and industrial processes.
資料ダウンロード
QCD管理受発注クラウド「newji」は、受発注部門で必要なQCD管理全てを備えた、現場特化型兼クラウド型の今世紀最高の受発注管理システムとなります。
ユーザー登録
受発注業務の効率化だけでなく、システムを導入することで、コスト削減や製品・資材のステータス可視化のほか、属人化していた受発注情報の共有化による内部不正防止や統制にも役立ちます。
NEWJI DX
製造業に特化したデジタルトランスフォーメーション(DX)の実現を目指す請負開発型のコンサルティングサービスです。AI、iPaaS、および先端の技術を駆使して、製造プロセスの効率化、業務効率化、チームワーク強化、コスト削減、品質向上を実現します。このサービスは、製造業の課題を深く理解し、それに対する最適なデジタルソリューションを提供することで、企業が持続的な成長とイノベーションを達成できるようサポートします。
製造業ニュース解説
製造業、主に購買・調達部門にお勤めの方々に向けた情報を配信しております。
新任の方やベテランの方、管理職を対象とした幅広いコンテンツをご用意しております。
お問い合わせ
コストダウンが利益に直結する術だと理解していても、なかなか前に進めることができない状況。そんな時は、newjiのコストダウン自動化機能で大きく利益貢献しよう!
(β版非公開)