- お役立ち記事
- Fundamentals of sparse modeling and applications to multivariate analysis
月間76,176名の
製造業ご担当者様が閲覧しています*
*2025年3月31日現在のGoogle Analyticsのデータより
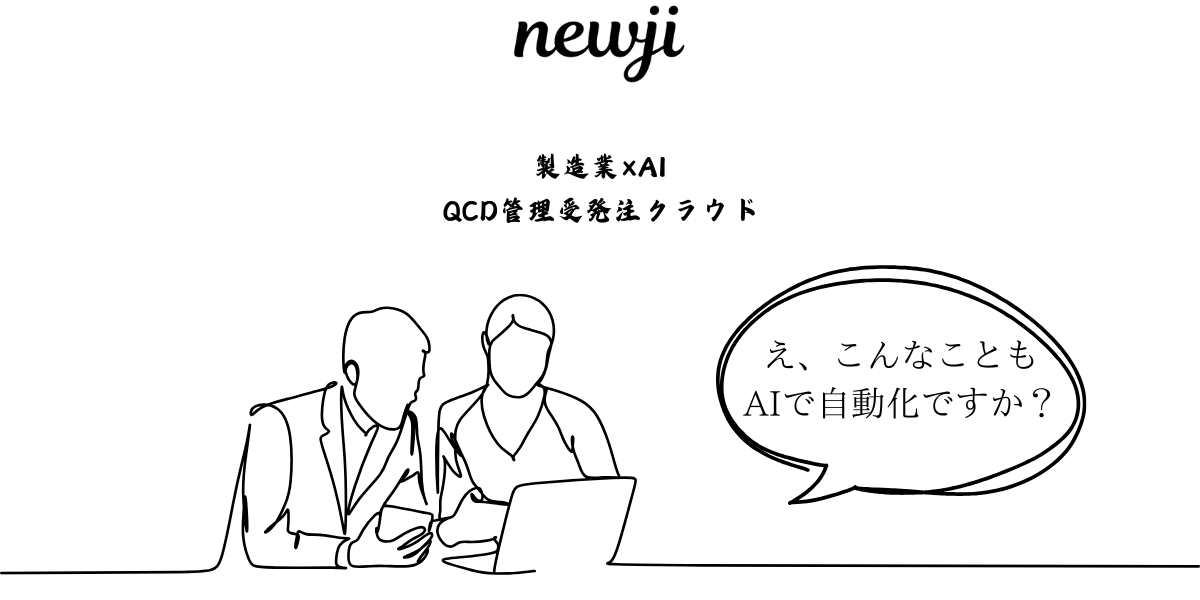
Fundamentals of sparse modeling and applications to multivariate analysis
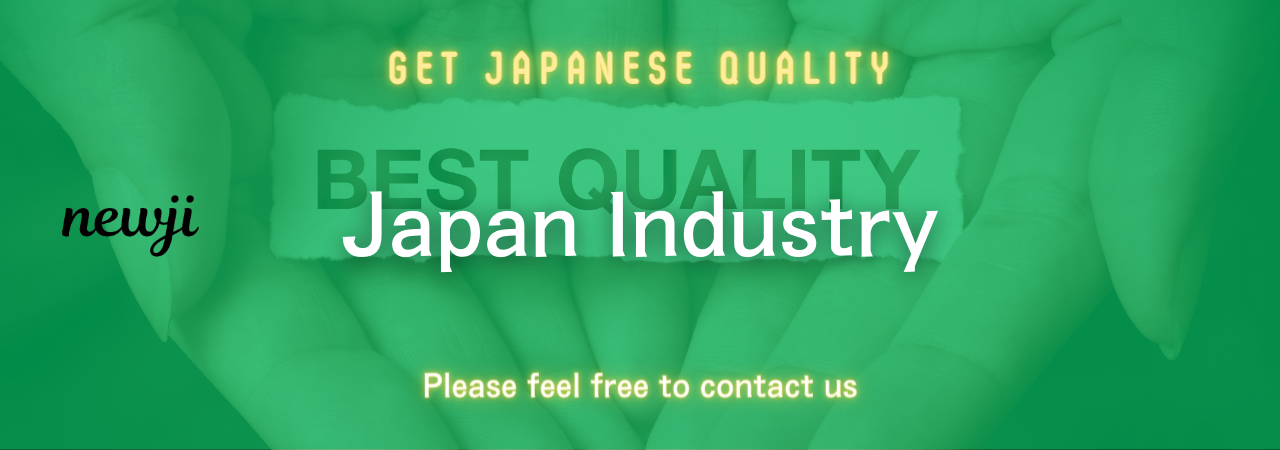
目次
Introduction to Sparse Modeling
Sparse modeling is a technique used in data analysis and statistics to simplify complex datasets by identifying and focusing on only the most important variables or features.
This approach can significantly enhance the efficiency and accuracy of data analysis, as it reduces the dimensionality of the data and highlights the most relevant information.
In the context of multivariate analysis, sparse modeling becomes particularly valuable because it can help to manage the complexity and high dimensionality often present in such datasets.
By focusing on a smaller subset of variables, analysts can gain clearer insights and make more accurate predictions.
What Is Sparse Modeling?
Sparse modeling refers to any technique designed to create simpler models that capture essential information from large datasets.
These models use a minimal number of features or components to achieve good predictive performance.
One of the primary goals of sparse modeling is to enhance interpretability by reducing the noise inherent in high-dimensional data.
This simplification process also helps to prevent overfitting, a common problem in complex models where the model fits the training data too well, often at the expense of generality and accuracy in testing or real-world scenarios.
Sparse modeling methods include techniques like LASSO (Least Absolute Shrinkage and Selection Operator), forward selection, backward elimination, and principal component analysis (PCA).
These methods are adept at identifying which features or attributes are most important for predicting a target variable.
Importance of Sparse Modeling in Analysis
Sparse modeling offers numerous benefits, making it a preferred approach in multivariate analysis:
Improved Interpretability
By reducing the number of variables in a dataset, sparse models provide a clearer and more understandable interpretation of the relationships between variables.
This clarity is critical in fields where decision-making relies on transparent models, such as finance, medicine, and social sciences.
Enhanced Predictive Performance
Sparse models are less prone to overfitting, which can happen when models are too complex.
By concentrating on the most significant variables, sparse models often deliver better predictive performance and generalize more effectively to new data.
Computational Efficiency
Sparse modeling reduces the computational burden associated with processing large datasets.
This efficiency is particularly beneficial when working with big data, where processing resources and time constraints can be significant factors.
Robustness to Noise
Removing irrelevant or noisy variables makes the model more robust to noise, improving the reliability of the analysis results.
This robustness is especially advantageous in environments where data quality may be an issue.
Applications of Sparse Modeling in Multivariate Analysis
Sparse modeling techniques have found widespread application across diverse fields involving multivariate analysis:
Machine Learning and Artificial Intelligence
In machine learning, sparse modeling is used to select features that contribute most to the predictive accuracy of models.
This feature selection is crucial for reducing the complexity of models, making them more efficient and easier to generalize.
Problems such as image recognition, natural language processing, and predictive analytics use sparse models to enhance algorithm performance.
Genomics and Bioinformatics
In bioinformatics, sparse modeling is used to identify genes or markers associated with specific diseases or biological traits.
Given the large volumes of data in genomic studies, sparse modeling is critical in isolating significant genetic information from vast datasets, facilitating better understanding and advances in medical research.
Finance and Economics
In finance, sparse models are employed to predict stock prices, interest rates, and economic indicators by focusing on the most impactful economic factors.
By refining the analysis to key indicators, financial analysts can more accurately predict market trends and economic movements.
Signal Processing and Telecommunications
In signal processing, sparse techniques improve the clarity and quality of signals by removing unnecessary or irrelevant information.
This application is vital in telecommunications, where signal transmission and reception depend on the integrity and clarity of the signal.
Challenges in Sparse Modeling
While sparse modeling offers many advantages, it also presents certain challenges:
Variable Selection
Determining which variables to include in a sparse model can be complex.
While algorithms like LASSO help automate feature selection, the process can still be computationally difficult and requires careful tuning of parameters.
Interpretability vs. Performance Trade-off
A sparse model’s simplicity can sometimes lead to reduced predictive accuracy if essential variables are omitted.
Analysts must balance the trade-off between model interpretability and performance.
Handling Non-linear Relationships
Sparse models usually assume linear relationships among variables.
However, real-world data often exhibit non-linear dynamics, necessitating sophisticated techniques or hybrid models to address this complexity.
Data Quality
Sparse modeling techniques are sensitive to data quality.
Outliers and missing values can skew results, so it’s essential to preprocess and clean data before analysis.
Conclusion
Sparse modeling is a powerful approach in the array of techniques available for multivariate analysis.
Its focus on reducing complexity and highlighting significant variables makes it invaluable across various fields, from finance to genomics.
As the volume and complexity of available data continue to grow, the demand for efficient and effective data reduction techniques like sparse modeling will only increase.
Understanding the fundamentals and applications of sparse modeling equips analysts and researchers with the tools needed to extract meaningful insights from complex datasets, leading to more informed decisions and discoveries.
資料ダウンロード
QCD管理受発注クラウド「newji」は、受発注部門で必要なQCD管理全てを備えた、現場特化型兼クラウド型の今世紀最高の受発注管理システムとなります。
ユーザー登録
受発注業務の効率化だけでなく、システムを導入することで、コスト削減や製品・資材のステータス可視化のほか、属人化していた受発注情報の共有化による内部不正防止や統制にも役立ちます。
NEWJI DX
製造業に特化したデジタルトランスフォーメーション(DX)の実現を目指す請負開発型のコンサルティングサービスです。AI、iPaaS、および先端の技術を駆使して、製造プロセスの効率化、業務効率化、チームワーク強化、コスト削減、品質向上を実現します。このサービスは、製造業の課題を深く理解し、それに対する最適なデジタルソリューションを提供することで、企業が持続的な成長とイノベーションを達成できるようサポートします。
製造業ニュース解説
製造業、主に購買・調達部門にお勤めの方々に向けた情報を配信しております。
新任の方やベテランの方、管理職を対象とした幅広いコンテンツをご用意しております。
お問い合わせ
コストダウンが利益に直結する術だと理解していても、なかなか前に進めることができない状況。そんな時は、newjiのコストダウン自動化機能で大きく利益貢献しよう!
(β版非公開)