- お役立ち記事
- Fundamentals of statistical causal inference and points of use
Fundamentals of statistical causal inference and points of use
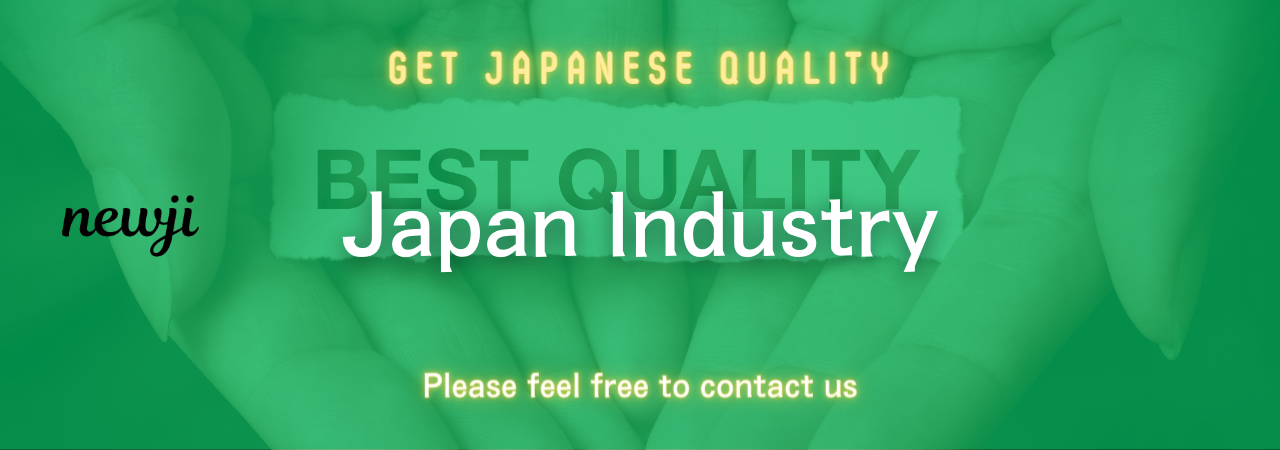
目次
Understanding Statistical Causal Inference
Statistical causal inference is a crucial aspect of research in various fields such as economics, medicine, and social sciences.
It refers to the process of identifying and quantifying the causal effect of one variable on another.
The key challenge in causal inference is to differentiate between correlation and causation.
While correlation implies a relationship between two variables, causation indicates that a change in one variable directly impacts the other.
To understand statistical causal inference, it is essential to recognize several approaches and frameworks.
These methodologies are designed to ensure that the association observed between variables is not merely coincidental or spurious.
Fundamentally, causal inference seeks to answer the question: “What would happen to the outcome if we changed the treatment or exposure?”
Key Concepts in Statistical Causal Inference
To apply causal inference effectively, one must be familiar with concepts such as:
1. **Causality and Association**:
– Causality implies a direct effect of one variable on another.
– Association indicates a relationship that is not necessarily causal.
2. **Confounding Variables**:
– These are variables that affect both the treatment and the outcome, potentially biasing the estimated causal effect.
– Controlling for confounding variables is crucial to establish a valid causal relationship.
3. **Counterfactuals**:
– The idea of counterfactuals is central to causal inference.
– It involves considering what would have happened to the same individual if they had received a different treatment.
4. **Randomization**:
– Randomization is often used to eliminate the influence of confounding variables.
– Through random assignment to treatment and control groups, the chances of confounding are minimized.
Popular Methods of Causal Inference
Several methods and techniques are employed to draw causal conclusions from data.
Some of the widely recognized methods include:
1. **Randomized Controlled Trials (RCTs)**:
– RCTs are considered the gold standard for causal inference.
– Participants are randomly assigned to either the treatment or control group, reducing the risk of bias.
2. **Observational Studies**:
– When RCTs are not feasible, researchers rely on observational studies.
– These studies require sophisticated statistical techniques to account for confounding factors and other biases.
3. **Instrumental Variables (IV)**:
– IV is used when randomization is not possible, but an external factor (instrument) affects the treatment.
– This method helps isolate the causal impact of the treatment on the outcome.
4. **Regression Discontinuity Design (RDD)**:
– RDD focuses on situations where treatment is assigned based on a cutoff point.
– It compares outcomes for observations just above and below the cutoff to estimate causal effects.
5. **Propensity Score Matching**:
– This technique involves pairing treated and untreated individuals with similar characteristics.
– It aims to mimic randomization by balancing confounding variables across groups.
Applications of Statistical Causal Inference
Statistical causal inference has numerous applications across different disciplines:
1. **Healthcare and Medicine**:
– In clinical trials, causal inference methods are used to evaluate the effectiveness of new treatments or interventions.
– Understanding the causal relationships helps in making informed medical decisions and developing healthcare policies.
2. **Economics**:
– Economists use causal inference to analyze the impact of policy changes, such as tax reforms or educational interventions.
– It is essential for assessing the effects on economic outcomes, such as employment or income levels.
3. **Social Sciences**:
– In sociology and psychology, researchers explore the causal impacts of social programs or psychological treatments.
– Causal inference aids in understanding complex social structures and human behavior.
4. **Public Policy**:
– Policymakers apply causal inference techniques to evaluate the success of public policies and programs.
– By understanding causality, they can implement effective solutions to societal issues.
Challenges in Statistical Causal Inference
Despite its utility, statistical causal inference presents several challenges:
1. **Identifying Confounding Variables**:
– It is often difficult to identify and control all potential confounding variables.
– Failure to account for these can lead to biased estimations.
2. **Data Limitations**:
– Causal analysis requires large and high-quality datasets.
– Data limitations, such as missing values or measurement errors, can impact the validity of causal conclusions.
3. **Model Assumptions**:
– Many causal inference methods rely on strong assumptions which might not hold in all scenarios.
– Violations of these assumptions can lead to incorrect inferences.
4. **Complex Interactions**:
– In real-world settings, variables may interact in complex ways.
– Capturing these interactions in a causal framework can be highly challenging.
Conclusion
Understanding and applying statistical causal inference is vital for deriving meaningful insights from data.
While numerous methods and frameworks help address causal questions, researchers must remain vigilant about the assumptions underlying these methods.
By carefully designing studies and selecting appropriate techniques, it’s possible to uncover causal relationships that drive informed decision-making across a wide range of fields.
Whether in healthcare, economics, or public policy, mastering the fundamentals of statistical causal inference empowers researchers and practitioners to impact personal and societal outcomes positively.
資料ダウンロード
QCD調達購買管理クラウド「newji」は、調達購買部門で必要なQCD管理全てを備えた、現場特化型兼クラウド型の今世紀最高の購買管理システムとなります。
ユーザー登録
調達購買業務の効率化だけでなく、システムを導入することで、コスト削減や製品・資材のステータス可視化のほか、属人化していた購買情報の共有化による内部不正防止や統制にも役立ちます。
NEWJI DX
製造業に特化したデジタルトランスフォーメーション(DX)の実現を目指す請負開発型のコンサルティングサービスです。AI、iPaaS、および先端の技術を駆使して、製造プロセスの効率化、業務効率化、チームワーク強化、コスト削減、品質向上を実現します。このサービスは、製造業の課題を深く理解し、それに対する最適なデジタルソリューションを提供することで、企業が持続的な成長とイノベーションを達成できるようサポートします。
オンライン講座
製造業、主に購買・調達部門にお勤めの方々に向けた情報を配信しております。
新任の方やベテランの方、管理職を対象とした幅広いコンテンツをご用意しております。
お問い合わせ
コストダウンが利益に直結する術だと理解していても、なかなか前に進めることができない状況。そんな時は、newjiのコストダウン自動化機能で大きく利益貢献しよう!
(Β版非公開)