- お役立ち記事
- Fundamentals of system identification and model validation for control
月間77,185名の
製造業ご担当者様が閲覧しています*
*2025年2月28日現在のGoogle Analyticsのデータより
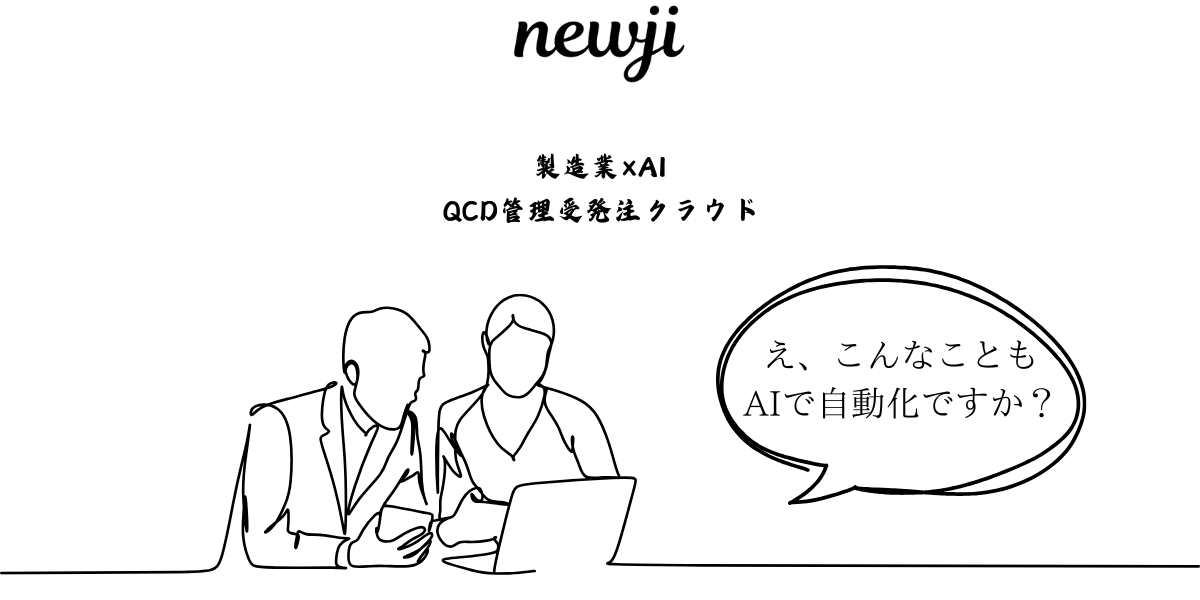
Fundamentals of system identification and model validation for control
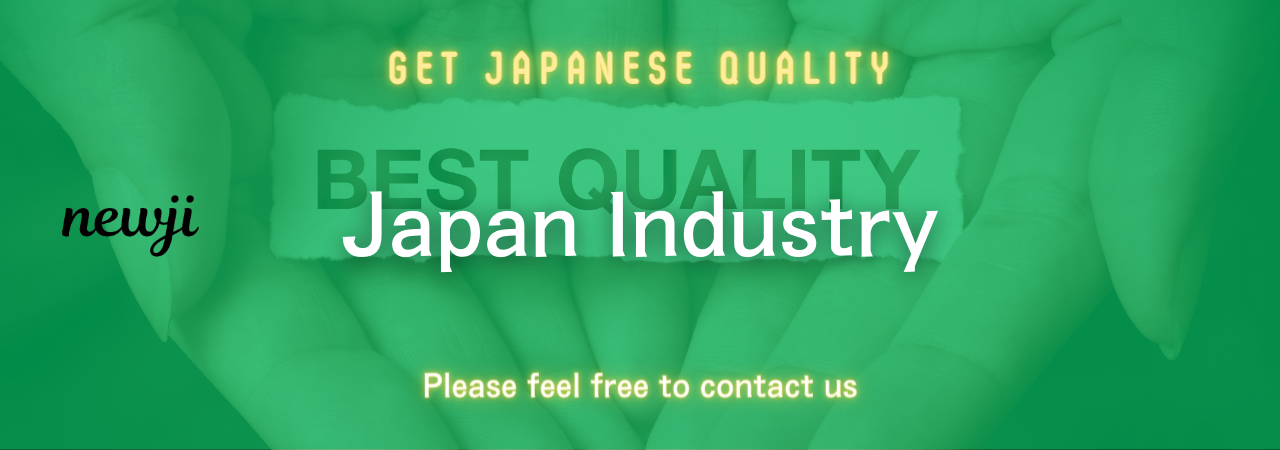
目次
Understanding System Identification
System identification is a crucial process in control engineering and signal processing.
It refers to the development of mathematical models of dynamic systems from measured data.
The primary goal is to predict future states of the system or design a control algorithm that regulates this system effectively.
The process begins by selecting a suitable model structure.
This can be achieved either through physical relationships or empirical data analysis.
The models can be linear or non-linear, continuous or discrete, depending on the system’s characteristics and the desired outcome.
Once a model structure is chosen, the task is to estimate the model parameters.
Techniques such as least squares estimation, maximum likelihood estimation, and Bayesian estimation are commonly used for parameter estimation.
These techniques rely on minimizing the difference, known as the error, between the model output and the measured data.
A well-identified model will have parameters that replicate the system behavior accurately.
Importance of Model Validation
Model validation is an essential step that follows system identification.
It ensures that the mathematical model accurately represents the real-world system for which it was developed.
Without model validation, there is a risk of implementing a control system that could lead to system inefficiencies or failures.
Validation involves verifying that the model can predict future data it has not been trained on.
This is typically performed by dividing the data into a training set and a validation set.
The model is developed using the training set and tested against the validation set to determine its predictive capabilities.
Statistical methods, such as cross-validation and residual analysis, are employed to assess model validity.
Residuals, which are errors between observed and modeled outputs, should resemble white noise—random and possessing no patterns.
This indicates that the model captures the system’s dynamics accurately without leaving out essential behaviors.
Types of Models in System Identification
Several types of models can be employed in system identification.
Selecting the appropriate model is contingent upon the system’s nature and the specific purpose of the identification.
Linear Time-Invariant (LTI) Models
LTI models are the simplest form of dynamic models and are used for systems that exhibit linear behaviors that remain consistent over time.
This form includes transfer functions, state-space representations, and frequency response models.
They are well-suited to systems with constant parameters and predictable inputs.
Non-Linear Models
Non-linear models are necessary when the system exhibits complex behaviors that change with time or input conditions.
These include Volterra series, Wiener models, and neural networks.
Identifying non-linear models requires additional techniques, such as polynomial approximations or piecewise linearization.
Continuous and Discrete-Time Models
Models can also be categorized by their representation of time.
Continuous-time models describe systems in an ongoing time frame, while discrete-time models capture systems at distinct time intervals.
The selection between continuous and discrete depends on the system’s operating environment and the sampling process.
Steps in System Identification
Successful system identification encompasses multiple steps.
Each step is crucial in ensuring an accurate and effective model.
Data Collection
The first step is collecting data that adequately represents the system’s behavior.
This data should be accurate, comprehensive, and collected under various operating conditions to provide a broad understanding of the system dynamics.
Model Selection
Next, a model structure is selected based on system characteristics and identification requirements.
This decision influences the complexity of the model and the estimation techniques to be used.
Parameter Estimation
Once a model structure is selected, the next step is estimating its parameters using collected data.
This requires choosing an appropriate estimation technique and evaluating its effectiveness in reducing prediction errors.
Model Validation
After parameter estimation, the model must undergo validation to ensure reliability.
This involves comparing the model’s predictions with actual system data and analyzing the residuals.
Implementation and Refinement
Upon validation, the model can be implemented in a control system.
Ongoing refinement may be necessary to adapt to changing system conditions or improve model performance.
Challenges in System Identification
Despite advancements in technology and methodology, system identification poses challenges.
Noise and Disturbances
Data collection is often hampered by noise and external disturbances, making it difficult to capture accurate system behaviors.
Complex System Dynamics
Many systems display complex, non-linear dynamics that require sophisticated modeling techniques and advanced mathematical tools.
Data Limitations
In some cases, the data obtained may not be sufficient to portray the full scope of system dynamics, limiting the accuracy of the model.
Conclusion
System identification and model validation form the backbone of modern control systems.
Understanding these fundamentals is essential for developing reliable and effective models that ensure optimal system performance.
By properly collecting data, selecting appropriate models, estimating parameters, and validating outputs, engineers can create robust control solutions that address various system challenges.
As technology evolves, continued research and development in this field will drive further advancements, empowering even more precise system control applications.
資料ダウンロード
QCD管理受発注クラウド「newji」は、受発注部門で必要なQCD管理全てを備えた、現場特化型兼クラウド型の今世紀最高の受発注管理システムとなります。
ユーザー登録
受発注業務の効率化だけでなく、システムを導入することで、コスト削減や製品・資材のステータス可視化のほか、属人化していた受発注情報の共有化による内部不正防止や統制にも役立ちます。
NEWJI DX
製造業に特化したデジタルトランスフォーメーション(DX)の実現を目指す請負開発型のコンサルティングサービスです。AI、iPaaS、および先端の技術を駆使して、製造プロセスの効率化、業務効率化、チームワーク強化、コスト削減、品質向上を実現します。このサービスは、製造業の課題を深く理解し、それに対する最適なデジタルソリューションを提供することで、企業が持続的な成長とイノベーションを達成できるようサポートします。
製造業ニュース解説
製造業、主に購買・調達部門にお勤めの方々に向けた情報を配信しております。
新任の方やベテランの方、管理職を対象とした幅広いコンテンツをご用意しております。
お問い合わせ
コストダウンが利益に直結する術だと理解していても、なかなか前に進めることができない状況。そんな時は、newjiのコストダウン自動化機能で大きく利益貢献しよう!
(β版非公開)