- お役立ち記事
- Fundamentals of system identification methods and applications to dynamic system modeling using Bayesian estimation
月間76,176名の
製造業ご担当者様が閲覧しています*
*2025年3月31日現在のGoogle Analyticsのデータより
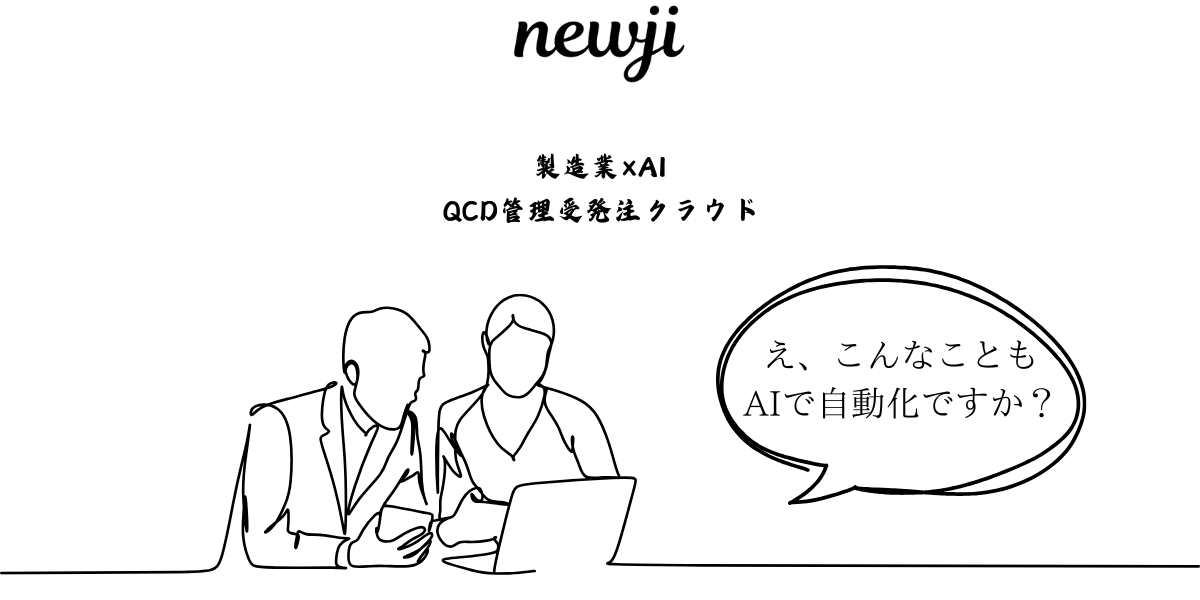
Fundamentals of system identification methods and applications to dynamic system modeling using Bayesian estimation
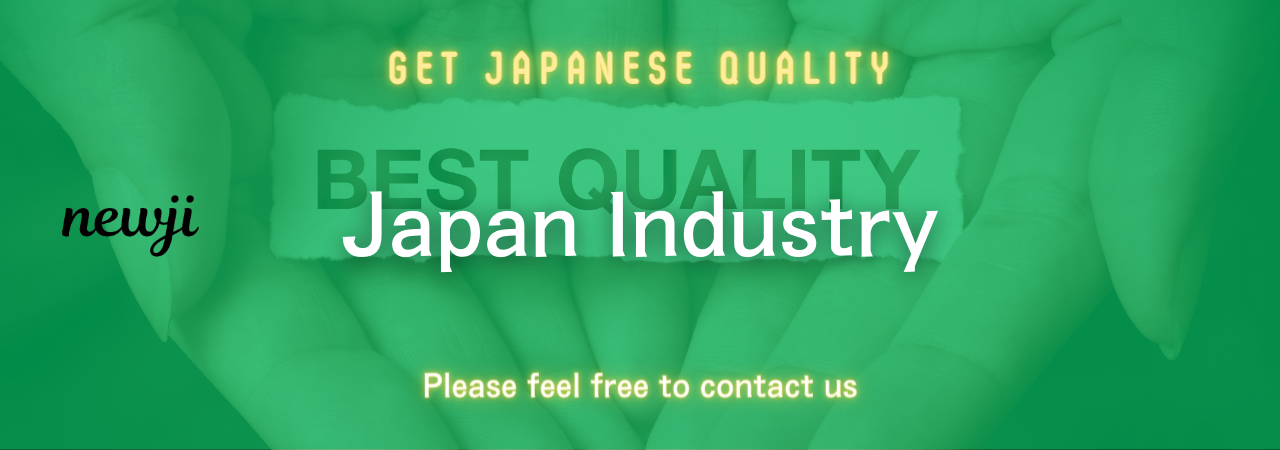
目次
Understanding System Identification
System identification is a fascinating area of study within the field of control engineering and applied mathematics.
It involves the development of mathematical models for dynamic systems through the use of measured data.
This process is crucial because accurate models help in predicting future behavior, controlling complex systems, and optimizing performance.
The fundamental idea behind system identification is to gather data from the system you want to model, analyze it, and then create a representation of the system that can be used for further development.
These models can be used in a variety of applications, from simple household gadgets to complex aerodynamics in aircraft.
Data Collection and Analysis
The first step in system identification is data collection.
It is important to gather data that accurately reflects the behavior of the system under various conditions.
This data can come from sensors, experiments, or historical records, depending on what is available and suitable for the specific system.
Once the data is collected, the next step is analysis.
This involves cleaning the data, dealing with any inconsistencies or noise, and then analyzing the patterns within it.
Techniques such as time-domain and frequency-domain analysis are commonly used in this stage to understand the dynamics and frequency response of the system.
Model Structure and Parameter Estimation
After analyzing the data, the next step is to choose an appropriate model structure.
This can range from simple linear models to complex nonlinear models, depending on the nature of the system.
Linear models are relatively straightforward and can be easy to work with, but they may not always capture the dynamics of complex systems.
Once a model structure is chosen, parameter estimation comes into play.
This involves finding the set of parameters that make the model fit the data as closely as possible.
Various techniques, such as least squares and maximum likelihood estimation, are used to find the optimal parameters.
Bayesian Estimation in Dynamic System Modeling
Bayesian estimation is a powerful tool used in system identification for dynamic system modeling.
It operates on the principles of Bayes’ theorem, which allows for the updating of the probability estimate for a hypothesis as additional data becomes available.
One of the key advantages of Bayesian estimation is its ability to incorporate prior knowledge into the modeling process.
This means that if there is existing information or expert knowledge about the system, it can be included in the estimation process to improve the accuracy of the model.
Incorporating Uncertainty
Dynamic systems often come with a high degree of uncertainty.
Bayesian estimation is particularly useful in such cases because it provides a probabilistic framework for dealing with these uncertainties.
By considering both the data and prior knowledge, Bayesian estimation can produce models that are robust even in the presence of noisy or incomplete data.
This ability to handle uncertainty makes Bayesian methods popular for a wide range of applications, including robotics, automotive systems, and even financial modeling.
Advantages Over Traditional Methods
Traditional methods like least squares are frequently used in system identification.
However, they lack the ability to incorporate prior knowledge and often fail when dealing with poor-quality data.
In contrast, Bayesian estimation not only utilizes prior information but also quantifies uncertainty, providing more reliable estimates.
Additionally, Bayesian methods offer a clearer insight into model parameters by generating a distribution of possible values rather than a single point estimate.
This distribution can then be used to understand the confidence we can have in the parameter estimates, which is crucial for decision-making processes.
Applications of System Identification
System identification is an essential tool in various industries.
In the automotive industry, it helps in the design of control systems that can improve the performance and safety of vehicles.
By developing accurate models of engine dynamics and other vehicle systems, engineers can better tune control strategies for optimal performance.
In the aerospace industry, system identification is used to model and simulate aircraft dynamics.
This assists in stability analysis, control system design, and pilot training simulations.
Moreover, it plays a critical role in the development of autonomous flight systems.
Another critical application is in the field of renewable energy, where system identification aids in the development of models for wind turbines and solar power systems.
These models help optimize energy conversion processes, predict maintenance needs, and improve the overall efficiency of power generation.
Future Trends and Innovations
The field of system identification is continuously evolving with advancements in technology and computing power.
Machine learning techniques are increasingly being integrated into system identification processes to handle large datasets and complex model structures.
The application of artificial intelligence and neural networks has led to more sophisticated models that can learn and adapt over time.
These advancements promise to enhance the accuracy and applicability of system identification, enabling its use in even more complex systems.
As industries continue to seek improvements in efficiency, safety, and performance, the demand for accurate system identification methods will undoubtedly grow.
Bayesian estimation will remain a valuable tool in this pursuit, providing robust and reliable modeling capabilities for a wide range of dynamic systems.
By understanding and implementing these concepts, engineers and researchers can create more precise and effective models, driving innovation and progress across numerous sectors.
資料ダウンロード
QCD管理受発注クラウド「newji」は、受発注部門で必要なQCD管理全てを備えた、現場特化型兼クラウド型の今世紀最高の受発注管理システムとなります。
ユーザー登録
受発注業務の効率化だけでなく、システムを導入することで、コスト削減や製品・資材のステータス可視化のほか、属人化していた受発注情報の共有化による内部不正防止や統制にも役立ちます。
NEWJI DX
製造業に特化したデジタルトランスフォーメーション(DX)の実現を目指す請負開発型のコンサルティングサービスです。AI、iPaaS、および先端の技術を駆使して、製造プロセスの効率化、業務効率化、チームワーク強化、コスト削減、品質向上を実現します。このサービスは、製造業の課題を深く理解し、それに対する最適なデジタルソリューションを提供することで、企業が持続的な成長とイノベーションを達成できるようサポートします。
製造業ニュース解説
製造業、主に購買・調達部門にお勤めの方々に向けた情報を配信しております。
新任の方やベテランの方、管理職を対象とした幅広いコンテンツをご用意しております。
お問い合わせ
コストダウンが利益に直結する術だと理解していても、なかなか前に進めることができない状況。そんな時は、newjiのコストダウン自動化機能で大きく利益貢献しよう!
(β版非公開)