- お役立ち記事
- Fundamentals of system identification methods and applications to system modeling using Bayesian estimation
月間76,176名の
製造業ご担当者様が閲覧しています*
*2025年3月31日現在のGoogle Analyticsのデータより
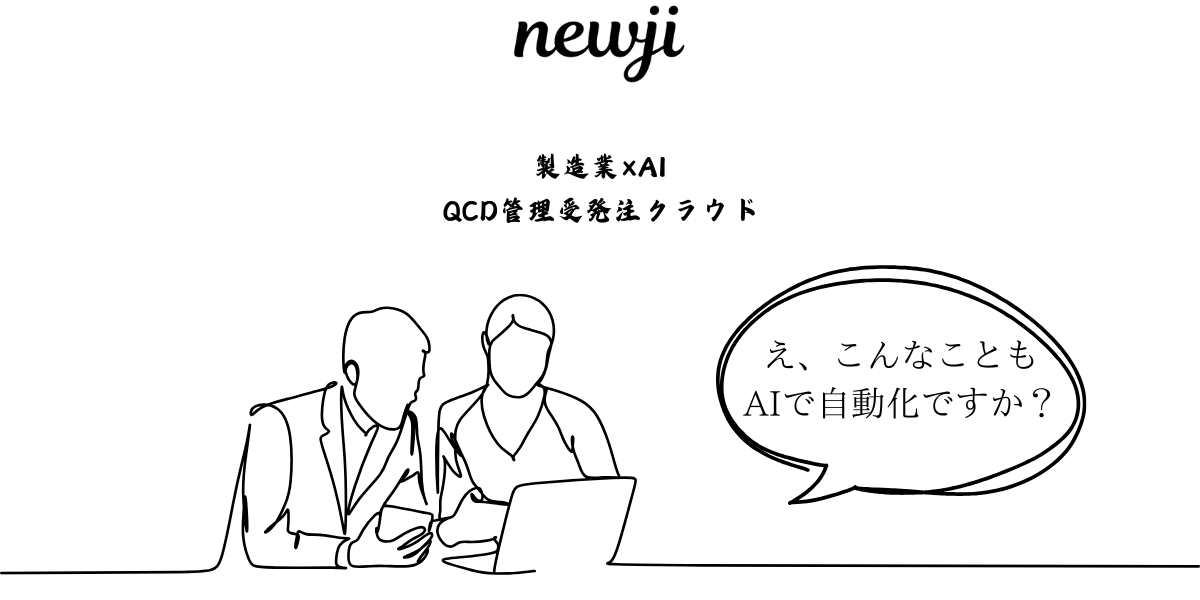
Fundamentals of system identification methods and applications to system modeling using Bayesian estimation
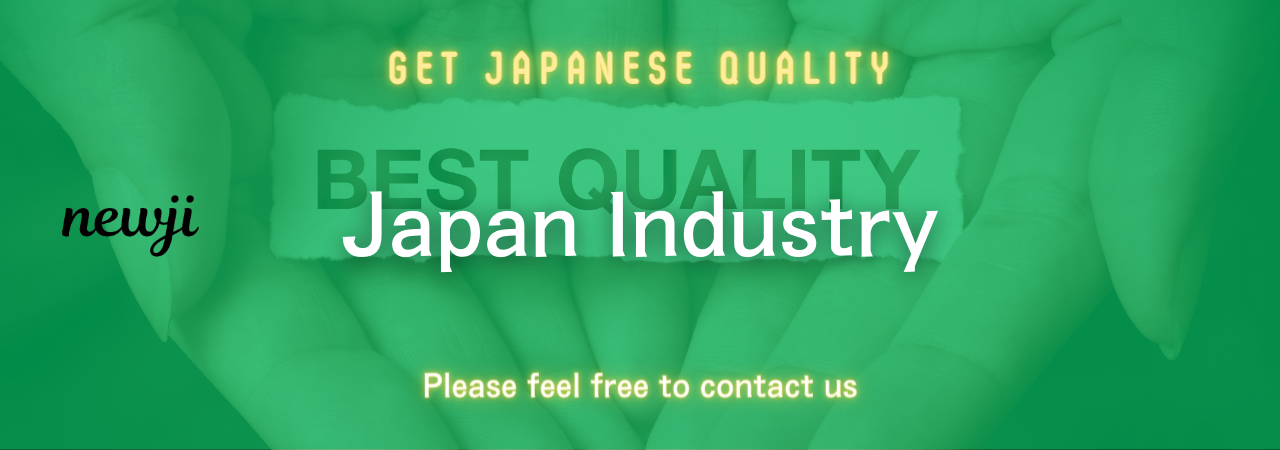
目次
Understanding System Identification
System identification is a fundamental aspect of control engineering and signal processing.
It involves constructing mathematical models of dynamic systems based on measured data.
The process helps engineers and researchers understand how a system behaves, predict future behavior, and design effective control systems.
In essence, it is the bridge that connects experimental data with theoretical models.
Why System Identification Matters
The importance of system identification cannot be overstated.
It is crucial in industries such as automotive, aerospace, manufacturing, and even finance, where dynamic systems are commonplace.
For example, in the automotive industry, system identification helps in designing better control systems for vehicle dynamics.
It allows engineers to understand how different components interact and how changes in one part affect the whole system.
Core Elements of System Identification
System identification involves several steps, each vital to creating an accurate model.
Data Collection
The first step in system identification is data collection.
This involves gathering input and output data from the system you intend to model.
It’s essential that this data accurately reflects the system’s behavior under normal operating conditions.
Poor quality data can lead to inaccurate models, which can result in ineffective or even harmful control systems.
Model Selection
Once data is collected, the next step is selecting an appropriate model structure.
There are several types of models, such as linear and nonlinear models, each suitable for different types of systems.
Linear models are often used for systems that have proportional relationships between inputs and outputs.
Nonlinear models are necessary when system behavior is more complex and cannot be approximated using linear relationships.
Parameter Estimation
Parameter estimation involves calculating the values of the parameters of the chosen model structure that best fit the collected data.
This step is crucial as it determines the accuracy of the model.
Several methods can be used for parameter estimation, including least squares method and maximum likelihood estimation.
The choice of method depends on the specific characteristics of the data and system.
Model Validation
After parameter estimation, the next step is model validation.
This involves testing the model to ensure it accurately represents the system.
Validation can be done by comparing the model’s output with actual system data not used during the estimation process.
If the model accurately predicts this data, it is considered valid.
If not, further refinement or an entirely different model structure may be necessary.
Introduction to Bayesian Estimation
Bayesian estimation is a powerful technique used in system identification and modeling.
It incorporates prior knowledge and observed data to estimate the probability distribution of model parameters.
This approach is particularly useful when there is uncertainty in data or when the system is complex.
Why Use Bayesian Estimation?
Bayesian estimation is favored in scenarios where traditional methods may fall short.
It allows for the incorporation of prior knowledge, which is essential when dealing with limited or noisy data.
This method provides a probabilistic approach, offering a complete view of parameter uncertainty rather than just point estimates.
This can be crucial for decision-making in complex systems where consequences of uncertainty can be significant.
Bayesian Estimation Process
The Bayesian estimation process involves the following steps:
1. **Define the Prior**: The prior represents existing beliefs or knowledge about the parameters before observing the data.
This could be based on previous experiments, theoretical knowledge, or expert opinion.
2. **Collect Data**: As in traditional system identification, the next step is to collect data from the system.
This data will be used in conjunction with the prior to estimate the parameters.
3. **Update Beliefs**: Bayesian estimation uses Bayes’ theorem to update prior beliefs based on the new data.
This results in a posterior distribution, which reflects updated knowledge about the parameters after considering the data.
4. **Posterior Analysis**: The final step involves analyzing the posterior distribution to make inferences about the parameters.
This could involve calculating the mean or mode of the distribution or assessing the uncertainty of parameter estimates.
Applications of Bayesian Estimation in System Modeling
Bayesian estimation is widely used across various industries for system modeling.
In the medical field, it helps in modeling the spread of diseases, allowing for more effective intervention strategies.
In finance, it is used for risk assessment and portfolio optimization, providing insight into the uncertainties involved in financial markets.
In engineering, Bayesian estimation assists in designing robust control systems that can operate effectively under uncertain conditions.
Conclusion
Understanding the fundamentals of system identification and the application of Bayesian estimation in system modeling is crucial in today’s data-driven world.
These processes enable us to create accurate models that help predict systems’ behaviors, leading to more efficient and effective designs in various fields.
By mastering these methodologies, engineers and researchers can better tackle the complexities of dynamic systems and enhance the performance and reliability of their designs.
資料ダウンロード
QCD管理受発注クラウド「newji」は、受発注部門で必要なQCD管理全てを備えた、現場特化型兼クラウド型の今世紀最高の受発注管理システムとなります。
ユーザー登録
受発注業務の効率化だけでなく、システムを導入することで、コスト削減や製品・資材のステータス可視化のほか、属人化していた受発注情報の共有化による内部不正防止や統制にも役立ちます。
NEWJI DX
製造業に特化したデジタルトランスフォーメーション(DX)の実現を目指す請負開発型のコンサルティングサービスです。AI、iPaaS、および先端の技術を駆使して、製造プロセスの効率化、業務効率化、チームワーク強化、コスト削減、品質向上を実現します。このサービスは、製造業の課題を深く理解し、それに対する最適なデジタルソリューションを提供することで、企業が持続的な成長とイノベーションを達成できるようサポートします。
製造業ニュース解説
製造業、主に購買・調達部門にお勤めの方々に向けた情報を配信しております。
新任の方やベテランの方、管理職を対象とした幅広いコンテンツをご用意しております。
お問い合わせ
コストダウンが利益に直結する術だと理解していても、なかなか前に進めることができない状況。そんな時は、newjiのコストダウン自動化機能で大きく利益貢献しよう!
(β版非公開)