- お役立ち記事
- Fundamentals of time series data analysis and applications to data analysis and prediction
月間76,176名の
製造業ご担当者様が閲覧しています*
*2025年3月31日現在のGoogle Analyticsのデータより
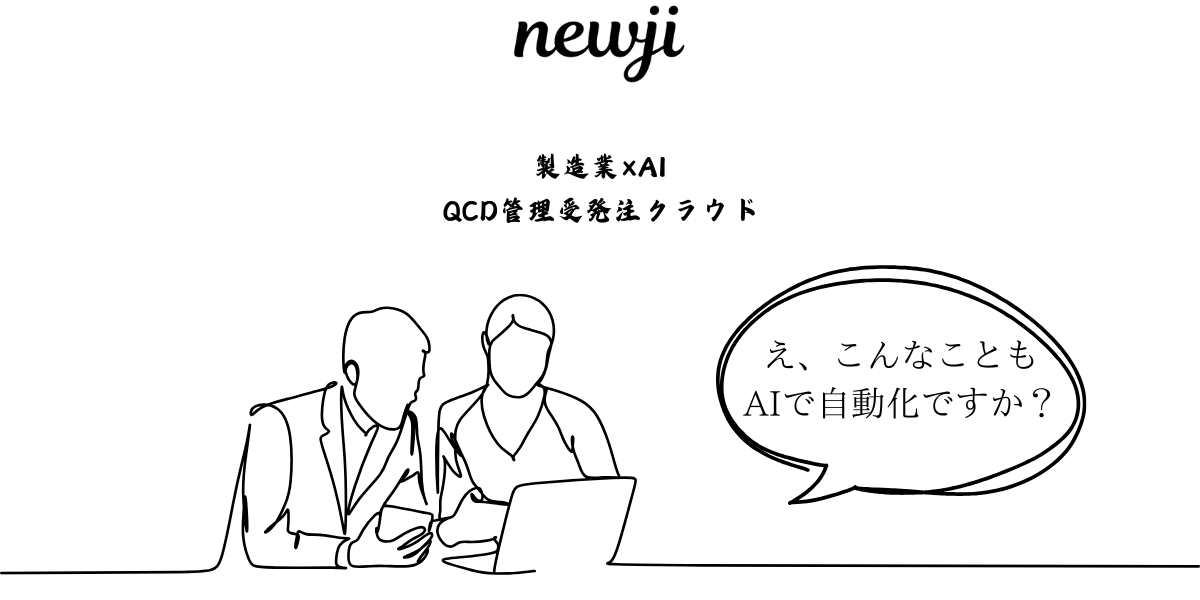
Fundamentals of time series data analysis and applications to data analysis and prediction
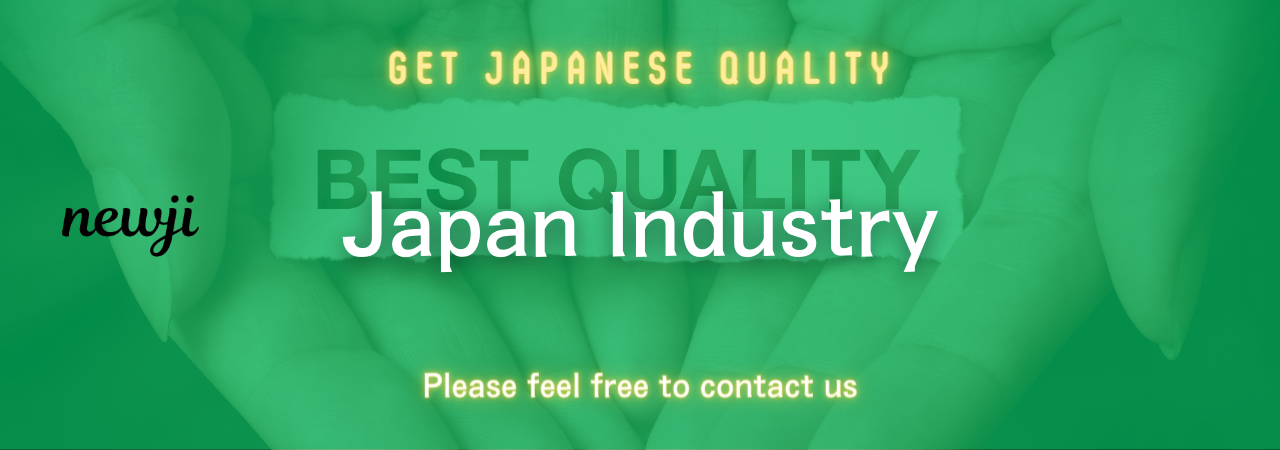
目次
Understanding Time Series Data
Time series data is a sequence of data points collected over time intervals.
These intervals could range from seconds, minutes, hours, or even years, depending on the field of study or business application.
In essence, any data that is time-stamped can be considered time series data.
One of the most relatable examples of time series data is daily temperature readings.
Every day’s temperature is recorded, and over time, you can observe patterns such as seasonal changes.
In business, time series data includes stock prices, sales numbers, or website traffic, recorded over specific periods.
Components of Time Series Data
Time series data often comprises several components that help in understanding the underlying patterns:
1. **Trend**: The overall direction in which the data is moving, either upwards or downwards, over a long period.
2. **Seasonality**: Recurring patterns or cycles over specific intervals, like weekly, monthly, or yearly.
3. **Cyclic**: Similar to seasonality, but cycles don’t occur with a fixed period and are generally influenced by economic conditions.
4. **Irregular/Random Variations**: Unpredictable changes or fluctuations in data due to random or unforeseen factors.
Understanding these components helps in decomposing the data and analyzing each aspect independently.
Applications of Time Series Data Analysis
Economic Forecasting
Time series data is extensively used in economic forecasting.
By analyzing trends and cycles in economic data like GDP, unemployment rates, and inflation, economists can predict future economic conditions.
This helps governments and businesses in planning and policy-making.
Stock Market Analysis
Investors and analysts use time series data to understand stock price movements.
By analyzing historical price data, it becomes easier to predict future price movements, identify buy or sell opportunities, and optimize portfolios.
Weather Prediction
Meteorologists use time series data of past weather conditions to forecast future weather events.
Based on patterns in temperature, humidity, and pressure data, predictions about weather conditions can be made with a certain degree of accuracy.
Sales and Demand Forecasting
Companies use time series data to predict future sales and demand.
By understanding past sales patterns and seasonal trends, businesses can manage inventory, staff, and resources more efficiently.
Techniques for Time Series Analysis
Several techniques are used to analyze time series data:
Exploratory Data Analysis (EDA)
EDA involves visualizing time series data to identify patterns, trends, and anomalies.
Techniques like line plots and heat maps are used to gain an initial understanding of the data.
Smoothing Methods
Smoothing techniques like Moving Averages and Exponential Smoothing help in eliminating noise from the data.
This makes trends and cycles more apparent.
Decomposition
Decomposition involves breaking down time series into its components: trend, seasonality, and residuals.
This aids in understanding the impact of each component on the data set.
ARIMA Models
AutoRegressive Integrated Moving Average (ARIMA) models are popular for forecasting time series data.
They consider both autoregression and moving averages, offering flexible approaches for different types of data.
Machine Learning Techniques
Advanced machine learning algorithms such as LSTM (Long Short-Term Memory) networks are used to handle complex time series data.
These models are particularly beneficial for datasets with multiple variables and complex structures.
Challenges in Time Series Analysis
Time series analysis comes with its set of challenges:
Data Quality
Poor quality data can lead to inaccurate analysis and predictions.
Data collection errors, missing data points, and noise can skew results, thus data preprocessing is essential.
Complex Patterns
Different series may exhibit very complex and multi-component patterns, making it difficult to disentangle trend and seasonality from cyclic patterns.
External Factors
Time series data might be influenced by external factors such as political events, economic crises, or technological changes which are hard to predict.
Conclusion
Time series data analysis is a powerful tool that provides insights across various fields.
With the right approach and techniques, it has the potential to reveal patterns and trends that inform decision-making and forecasting.
Understanding the fundamentals and challenges of time series analysis prepares us for effective application in predicting future events, ultimately aiding in strategic planning and resource optimization.
資料ダウンロード
QCD管理受発注クラウド「newji」は、受発注部門で必要なQCD管理全てを備えた、現場特化型兼クラウド型の今世紀最高の受発注管理システムとなります。
ユーザー登録
受発注業務の効率化だけでなく、システムを導入することで、コスト削減や製品・資材のステータス可視化のほか、属人化していた受発注情報の共有化による内部不正防止や統制にも役立ちます。
NEWJI DX
製造業に特化したデジタルトランスフォーメーション(DX)の実現を目指す請負開発型のコンサルティングサービスです。AI、iPaaS、および先端の技術を駆使して、製造プロセスの効率化、業務効率化、チームワーク強化、コスト削減、品質向上を実現します。このサービスは、製造業の課題を深く理解し、それに対する最適なデジタルソリューションを提供することで、企業が持続的な成長とイノベーションを達成できるようサポートします。
製造業ニュース解説
製造業、主に購買・調達部門にお勤めの方々に向けた情報を配信しております。
新任の方やベテランの方、管理職を対象とした幅広いコンテンツをご用意しております。
お問い合わせ
コストダウンが利益に直結する術だと理解していても、なかなか前に進めることができない状況。そんな時は、newjiのコストダウン自動化機能で大きく利益貢献しよう!
(β版非公開)