- お役立ち記事
- Fundamentals of time series data analysis and practical know-how for model identification and feature extraction
月間77,185名の
製造業ご担当者様が閲覧しています*
*2025年2月28日現在のGoogle Analyticsのデータより
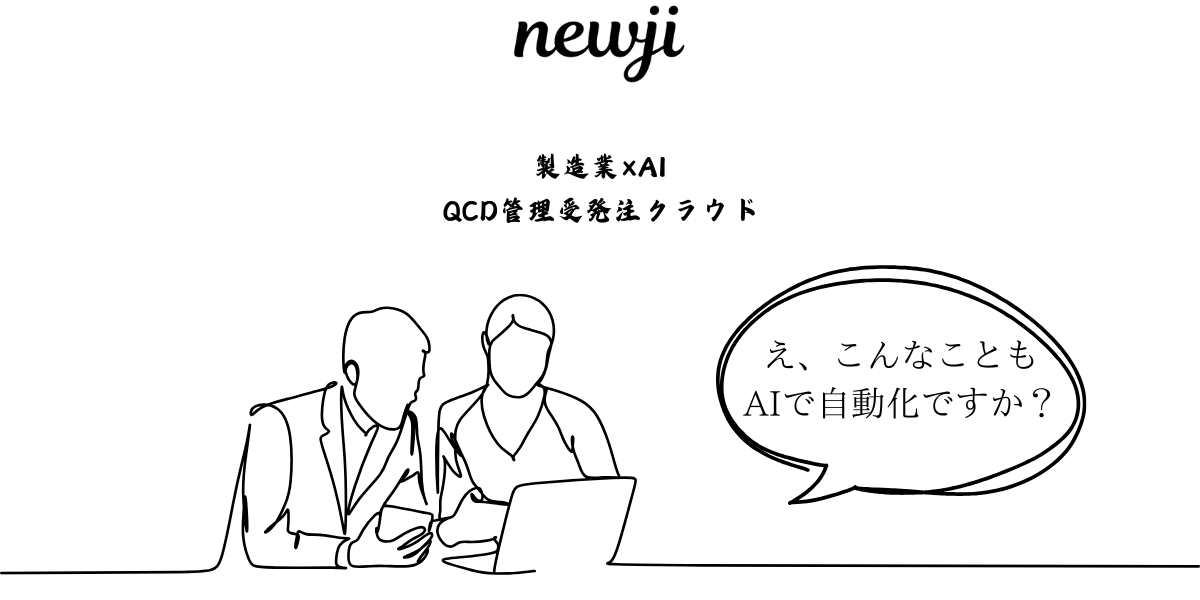
Fundamentals of time series data analysis and practical know-how for model identification and feature extraction
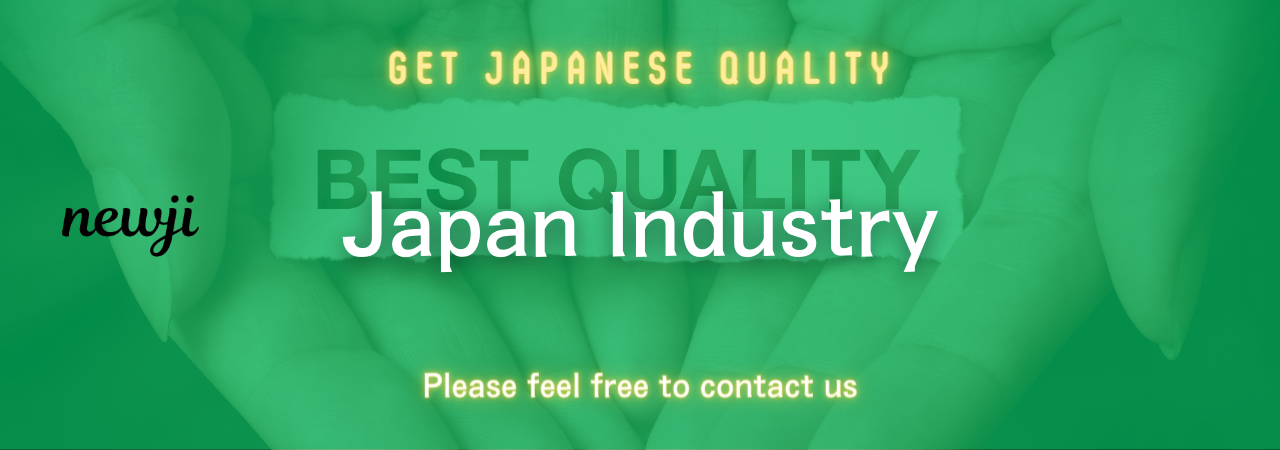
目次
Understanding Time Series Data
Time series data is a sequence of data points collected or recorded at successive points in time, often at regular intervals.
This type of data is incredibly prevalent and is used in a variety of fields such as economics, finance, environmental studies, and more.
From stock market prices to weather patterns and even website traffic, time series data allows us to track changes over time and make informed decisions based on observed trends.
A key characteristic of time series data is that observations are dependent on a temporal order.
This means that in order to understand the data, we must consider the time aspect, as this can influence the patterns and relationships we observe.
The Basics of Time Series Data Analysis
Time series data analysis involves a number of steps which help us understand and extract meaningful information from the data.
Here is a simplified roadmap:
1. Data Collection and Preparation
Before analysis can begin, data must be collected.
The data should be at regular intervals unless irregularity will be an aspect of the analysis.
Once collected, data cleaning is crucial.
This involves dealing with missing values, outliers, and ensuring consistency and accuracy.
2. Visualization
Visualizing time series data with plots is a powerful first step in analysis.
A time plot, with time on the x-axis and the variable of interest on the y-axis, can reveal trends, seasonal patterns, and potential anomalies.
3. Decomposition
Time series decomposition is the process of breaking down data into its components: trend, seasonality, and noise.
Decomposition helps to identify and isolate patterns which can improve further analysis.
4. Smoothing Techniques
Smoothing helps to remove noise from a time series and enhance understanding of its structure, primarily its trend.
Common methods include moving averages and exponential smoothing.
5. Identifying and Modeling Patterns
This is a crucial stage where you identify patterns and build models to describe them.
This can involve simple methods like linear regression or more complex statistical models like ARIMA (Auto-Regressive Integrated Moving Average).
Practical Know-How for Model Identification
Model identification is a step in the modeling process where you determine which type of model best represents your time series data.
Here’s how you can approach it:
1. Determine Stationarity
Stationarity means that the properties of a time series do not change over time.
ARIMA models, for example, require stationarity.
Use methods like the Augmented Dickey-Fuller test to check stationarity.
2. Use ACF and PACF
Autocorrelation Function (ACF) and Partial Autocorrelation Function (PACF) plots help in identifying the appropriate order of AR (Auto-Regressive) and MA (Moving Average) components for ARIMA models.
3. Employ Unit Root Testing
If your series is non-stationary, unit root tests like the Dickey-Fuller test can determine how many differencing steps are needed to achieve stationarity.
4. Evaluate Different Models
Fit various models and evaluate their performance using metrics such as AIC (Akaike Information Criterion) and BIC (Bayesian Information Criterion).
These metrics help to balance model complexity against goodness of fit.
Feature Extraction Techniques
To enhance the predictive power of time series models, feature extraction is critical.
This involves generating new features which help capture important aspects of the data.
1. Lag Features
Lag features involve using previous observations as predictors, which can be especially useful in forecasting.
2. Rolling Statistics
Calculating rolling means or rolling variance can help in capturing trends and potential seasonality.
3. Fourier Transforms
For series exhibiting complex periodic patterns, Fourier transforms can decompose the series into sinusoidal components.
This helps in capturing cyclicality.
4. Time-Based Features
Extract features based on the date or time component, such as day of the week, month, year, and holidays.
These can provide context and improve model performance.
Final Thoughts
Time series data analysis is a comprehensive process that involves detailed understanding and meticulous execution of various steps.
From collecting and preparing data to model identification and feature extraction, each stage builds upon the previous one to deliver insights and forecasts.
Having a strong grasp of these fundamentals allows for more effective data analysis and aids in making data-driven decisions across different sectors.
While the road from data to insight might be complex, following these best practices will help make your journey through time series analysis more successful and enlightening.
資料ダウンロード
QCD管理受発注クラウド「newji」は、受発注部門で必要なQCD管理全てを備えた、現場特化型兼クラウド型の今世紀最高の受発注管理システムとなります。
ユーザー登録
受発注業務の効率化だけでなく、システムを導入することで、コスト削減や製品・資材のステータス可視化のほか、属人化していた受発注情報の共有化による内部不正防止や統制にも役立ちます。
NEWJI DX
製造業に特化したデジタルトランスフォーメーション(DX)の実現を目指す請負開発型のコンサルティングサービスです。AI、iPaaS、および先端の技術を駆使して、製造プロセスの効率化、業務効率化、チームワーク強化、コスト削減、品質向上を実現します。このサービスは、製造業の課題を深く理解し、それに対する最適なデジタルソリューションを提供することで、企業が持続的な成長とイノベーションを達成できるようサポートします。
製造業ニュース解説
製造業、主に購買・調達部門にお勤めの方々に向けた情報を配信しております。
新任の方やベテランの方、管理職を対象とした幅広いコンテンツをご用意しております。
お問い合わせ
コストダウンが利益に直結する術だと理解していても、なかなか前に進めることができない状況。そんな時は、newjiのコストダウン自動化機能で大きく利益貢献しよう!
(β版非公開)