- お役立ち記事
- Fundamentals of time series data analysis, predictive model construction methods, and applications to detection and factor analysis
月間76,176名の
製造業ご担当者様が閲覧しています*
*2025年3月31日現在のGoogle Analyticsのデータより
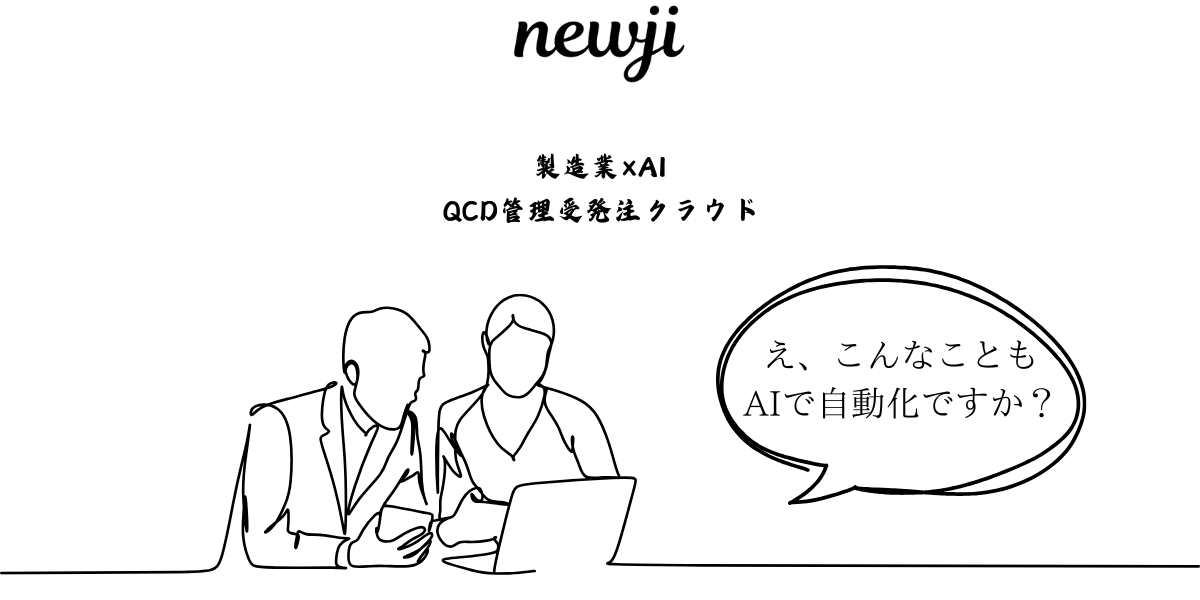
Fundamentals of time series data analysis, predictive model construction methods, and applications to detection and factor analysis
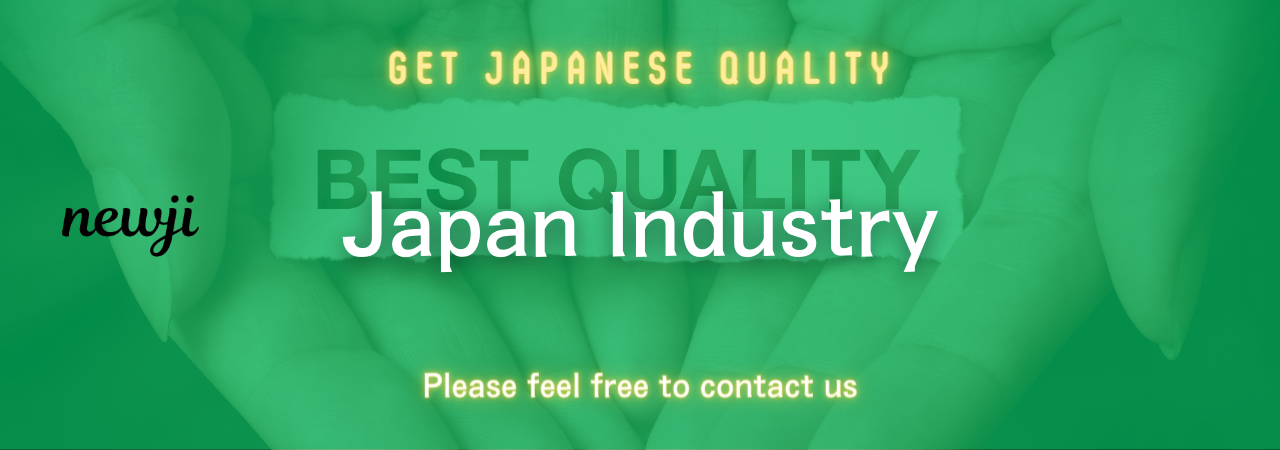
目次
What is Time Series Data?
Time series data is a collection of observations recorded sequentially over time.
This kind of data is characterized by the time component, which means that each data point is associated with a specific timestamp.
Time series can display trends, patterns, and cycles, which makes them vital in numerous fields including economics, finance, environmental science, and more.
The beauty of time series data lies in its capacity to provide insights into past dynamics and future predictions.
Stock market prices, temperature records, and sales data are all examples of time series data.
Understanding time series is pivotal because it helps to grasp how different factors evolve over time and how they relate to one another.
Fundamentals of Time Series Data Analysis
The fundamentals of time series data analysis revolve around handling and decoding data sets to unlock their inherent information.
This analysis usually starts with data visualization, allowing you to visually inspect and identify trends, seasonal patterns, or irregularities in the data.
Graphing the time series data often involves plotting it on a graph to see how it moves over time.
Decomposition of Time Series
Decomposition involves breaking down a time series into its components, namely trend, seasonality, and noise.
The trend shows the long-term movement in the data, seasonality shows regular patterns or cycles at specific intervals, and noise accounts for random variations with no identifiable pattern.
By understanding each of these components, analysts can create models that better represent the data.
Stationarity and Differencing
A stationary time series is one where statistical properties such as mean and variance remain constant over time.
Stationarity simplifies the modeling process while highlighting significant aspects of the data.
Differencing means subtracting a data point from the previous data point to stabilize the data over time.
Achieving stationarity often requires differentiating the data, making it easier to apply further analysis tools.
Predictive Model Construction Methods
Once you understand the time series data’s characteristics, constructing predictive models becomes feasible.
Several methods can be employed to build models that anticipate future trends based on historical data.
Moving Average and Exponential Smoothing
Moving average involves calculating an average of the data points over a defined period, smoothing out short-term fluctuations.
A variation of this approach is weighted moving average, with more recent data points given higher importance.
Exponential smoothing assigns exponentially decreasing weights as the observations get older, which allows it to give more importance to recent trends.
This method is useful for creating smoother and more reliable forecasting models.
ARIMA Models
ARIMA stands for AutoRegressive Integrated Moving Average.
This model combines autoregression (AR), differencing (I), and moving average (MA) to build robust predictive models.
AR models use dependencies between observations, MA models use past forecast errors, and integration refers to making a time series stationary.
ARIMA models are versatile as they cater to stationary data sets, providing an essential toolkit for time series forecasting.
Seasonal Decomposition of Time Series (SARIMA)
SARIMA extends the ARIMA model by adding seasonal components, making it apt for time series data with a seasonal pattern.
Seasonal Decomposition provides a fuller picture by addressing both the regular and seasonal operations within the dataset.
By incorporating these seasonal factors, SARIMA models refine the accuracy and reliability of predictions.
Applications in Detection and Factor Analysis
Applying time series analysis reaches far beyond forecasting, playing a crucial role in detecting anomalies and understanding underlying factors affecting data.
Anomaly Detection
Detecting anomalies or outliers allows for quick identification of unexpected events or deviations.
These could be changes in consumer behavior, economic downturns, or equipment failures.
A multitude of industries depends on anomaly detection to ensure system health and performance remain within acceptable boundaries.
Time series models help detecting anomalies by setting prediction intervals or control limits.
When data points exceed these thresholds, they signal potential anomalies that require further inspection.
Factor Analysis
Factor analysis delves deep into identifying the factors that significantly affect a time series.
This means extracting hidden variables or influences that impact the observed data.
Effectively, factor analysis helps streamline complex data into simpler, more manageable dimensions.
Through factor analysis, businesses can understand which variables drive sales volume, for example, or which environmental factors significantly affect crop yields.
This understanding then informs strategic decision-making processes.
The Future of Time Series Analysis
Time series analysis continues to evolve with advancements in machine learning and artificial intelligence.
These technological strides offer new possibilities for processing large sets of time series data with greater efficiency and accuracy.
The ability to automate model selection, hyperparameter tuning, and even anomaly detection is increasing.
Moreover, time series analysis is becoming more immersive, facilitating real-time data processing that translates into instantaneous business insights.
Whether it’s enhancing risk management in finance or optimizing demand forecasting in retail, time series analysis holds great promise for shaping the future.
In conclusion, mastering time series analysis enables significant contributions to various domains by unlocking the dormant potential within the data.
It rests on foundational principles of data decomposition, predictive modeling, and practical applications in detection and factor analysis.
By harnessing these insights, an array of industries stand to benefit through enhanced decision-making and strategic planning.
Understanding this compelling data form provides a competitive edge in any analytical endeavor.
資料ダウンロード
QCD管理受発注クラウド「newji」は、受発注部門で必要なQCD管理全てを備えた、現場特化型兼クラウド型の今世紀最高の受発注管理システムとなります。
ユーザー登録
受発注業務の効率化だけでなく、システムを導入することで、コスト削減や製品・資材のステータス可視化のほか、属人化していた受発注情報の共有化による内部不正防止や統制にも役立ちます。
NEWJI DX
製造業に特化したデジタルトランスフォーメーション(DX)の実現を目指す請負開発型のコンサルティングサービスです。AI、iPaaS、および先端の技術を駆使して、製造プロセスの効率化、業務効率化、チームワーク強化、コスト削減、品質向上を実現します。このサービスは、製造業の課題を深く理解し、それに対する最適なデジタルソリューションを提供することで、企業が持続的な成長とイノベーションを達成できるようサポートします。
製造業ニュース解説
製造業、主に購買・調達部門にお勤めの方々に向けた情報を配信しております。
新任の方やベテランの方、管理職を対象とした幅広いコンテンツをご用意しております。
お問い合わせ
コストダウンが利益に直結する術だと理解していても、なかなか前に進めることができない状況。そんな時は、newjiのコストダウン自動化機能で大きく利益貢献しよう!
(β版非公開)