- お役立ち記事
- Fundamentals of topology optimization and its application to higher efficiency and performance in motor design using machine learning
Fundamentals of topology optimization and its application to higher efficiency and performance in motor design using machine learning
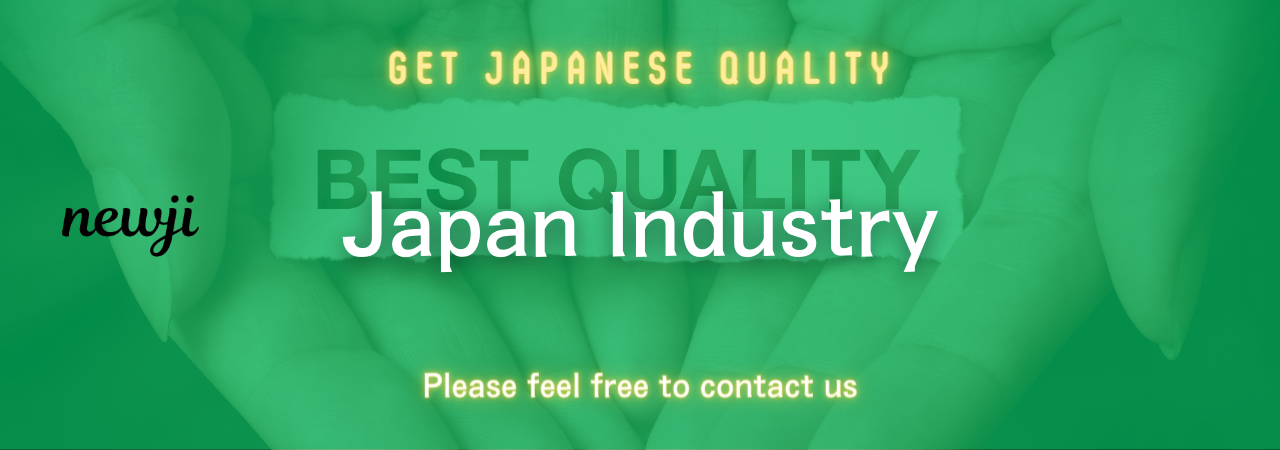
目次
Introduction to Topology Optimization
Topology optimization is an advanced method that assists in creating designs by optimizing the material layout within a given design space for a specified set of loads and boundary conditions.
It employs mathematical models to determine the optimal distribution of material, maximizing performance according to the given constraints.
This approach is crucial in many industries, including aerospace, automotive, and biomedical engineering, due to its ability to enhance efficiency and effectiveness in product design.
Topology optimization involves converting the design problem into an optimization problem where the objective is to improve performance.
It often involves defining a cost function, which could be related to stiffness, weight, compliance, or any other previously determined criterion.
The ultimate goal is to identify the best material layout that meets the performance goals and constraints.
The Role of Machine Learning in Topology Optimization
Machine learning has emerged as a powerful tool in reimagining topology optimization, making it faster and more effective than traditional methods.
By integrating machine learning algorithms, designers can identify patterns and make predictions that guide the optimization process more efficiently.
This integration helps reduce the computation time and enhances the ability to navigate large design spaces.
Machine learning models can be trained to predict the performance of different design configurations, thus streamlining the process of design evaluation and selection.
With the capacity to analyze vast amounts of data, machine learning supports the exploration of a wider range of design possibilities, improving the chances of finding the most optimal design for any given set of parameters.
Machine Learning Models in Topology Optimization
Various machine learning models, such as neural networks, support vector machines, and decision trees, play a significant role in topology optimization.
Neural networks, due to their ability to approximate complex, non-linear functions, are often employed for predicting optimal material layouts.
These networks can learn from existing optimized designs and apply that knowledge to new scenarios, improving the speed and accuracy of design predictions.
Support vector machines and decision trees also contribute to the process by classifying and filtering feasible design options.
These models can categorize potential designs based on their performance characteristics, allowing designers to focus on the most promising options.
Application in Motor Design
In the field of motor design, topology optimization and machine learning combine to enhance motor efficiency and performance.
Electric motors, pivotal in many modern applications, require designs that maximize power density while minimizing losses.
By using topology optimization, engineers can create motors that are lightweight yet robust, achieving better performance parameters.
Machine learning further refines this process by predicting outcomes of various design iterations quickly and reliably.
It helps in identifying the best design configurations by learning from existing motor designs, thereby reducing the need for costly and time-consuming trials and errors.
Improving Motor Efficiency
The application of topology optimization in motor design is particularly relevant for improving efficiency.
By optimizing the material distribution in components like rotors and stators, engineers can reduce energy losses and improve cooling efficiency.
This results in motors that run cooler, have longer service lives, and consume less energy, aligning with sustainability goals.
The incorporation of machine learning expedites the evaluation of these optimized designs, ensuring that the best possible configuration is chosen.
This approach allows for rapid prototyping and testing, accelerating the innovation cycle in motor design.
Challenges and Future Directions
While topology optimization and machine learning have significantly advanced product design and development, they present several challenges.
One prominent challenge is the complexity involved in modeling and simulating real-world physical phenomena accurately.
This requires high computational power and can sometimes lead to approximations, potentially affecting the optimization outcomes.
Furthermore, the integration of machine learning introduces challenges related to data quality and management.
Ensuring that the training data is accurate and representative of real-world conditions is crucial for the reliability of machine learning models.
Despite these hurdles, the future of topology optimization in motor design appears promising.
With advancements in computational power and more sophisticated algorithms, the ability to produce increasingly complex and efficient designs is expected to rise.
Continued research and development in machine learning methodologies will likely lead to improved precision in optimization processes and greater adaptability to various design challenges.
The Road Ahead
Looking ahead, the combination of topology optimization and machine learning is poised to disrupt traditional design methodologies across industries.
Advances in artificial intelligence are driving more intelligent, adaptive design processes, enabling engineers to push the boundaries of innovation further than ever before.
As these technologies continue to evolve, they promise not only to enhance product performance and efficiency but also to contribute to more sustainable design practices.
In motor design, this evolution could lead to the creation of more energy-efficient motors, playing a critical role in reducing global energy consumption and emissions.
Ultimately, the synergy between topology optimization and machine learning is reshaping the future of engineering, offering new possibilities for optimized, high-performance designs across diverse applications.
資料ダウンロード
QCD調達購買管理クラウド「newji」は、調達購買部門で必要なQCD管理全てを備えた、現場特化型兼クラウド型の今世紀最高の購買管理システムとなります。
ユーザー登録
調達購買業務の効率化だけでなく、システムを導入することで、コスト削減や製品・資材のステータス可視化のほか、属人化していた購買情報の共有化による内部不正防止や統制にも役立ちます。
NEWJI DX
製造業に特化したデジタルトランスフォーメーション(DX)の実現を目指す請負開発型のコンサルティングサービスです。AI、iPaaS、および先端の技術を駆使して、製造プロセスの効率化、業務効率化、チームワーク強化、コスト削減、品質向上を実現します。このサービスは、製造業の課題を深く理解し、それに対する最適なデジタルソリューションを提供することで、企業が持続的な成長とイノベーションを達成できるようサポートします。
オンライン講座
製造業、主に購買・調達部門にお勤めの方々に向けた情報を配信しております。
新任の方やベテランの方、管理職を対象とした幅広いコンテンツをご用意しております。
お問い合わせ
コストダウンが利益に直結する術だと理解していても、なかなか前に進めることができない状況。そんな時は、newjiのコストダウン自動化機能で大きく利益貢献しよう!
(Β版非公開)