- お役立ち記事
- Fundamentals of transfer learning and application of deep learning to improve learning efficiency
月間76,176名の
製造業ご担当者様が閲覧しています*
*2025年3月31日現在のGoogle Analyticsのデータより
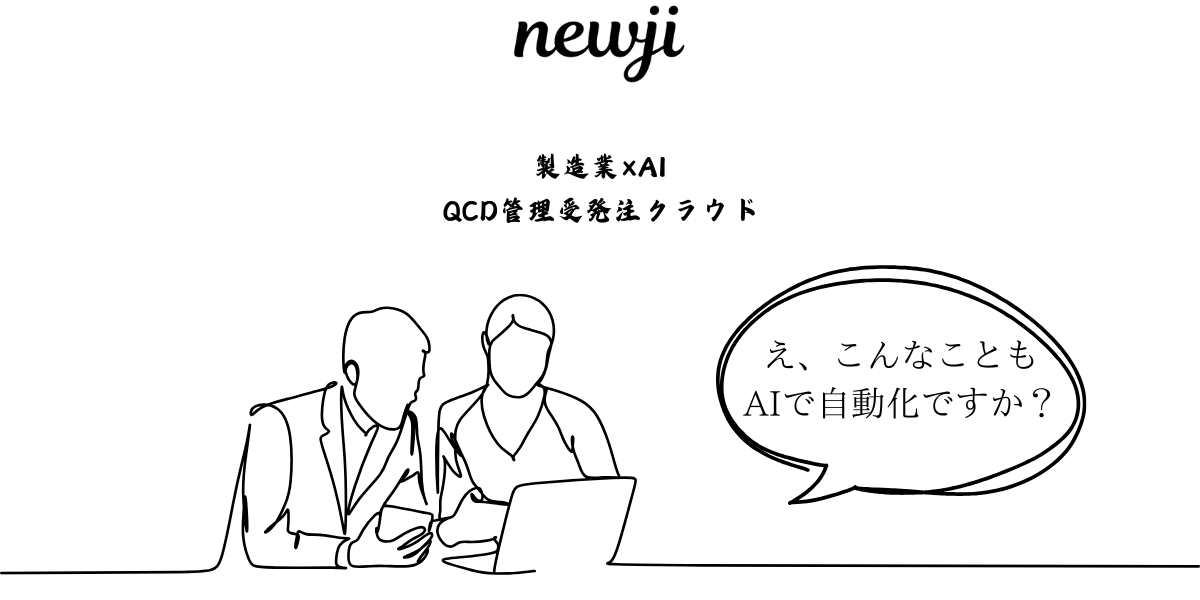
Fundamentals of transfer learning and application of deep learning to improve learning efficiency
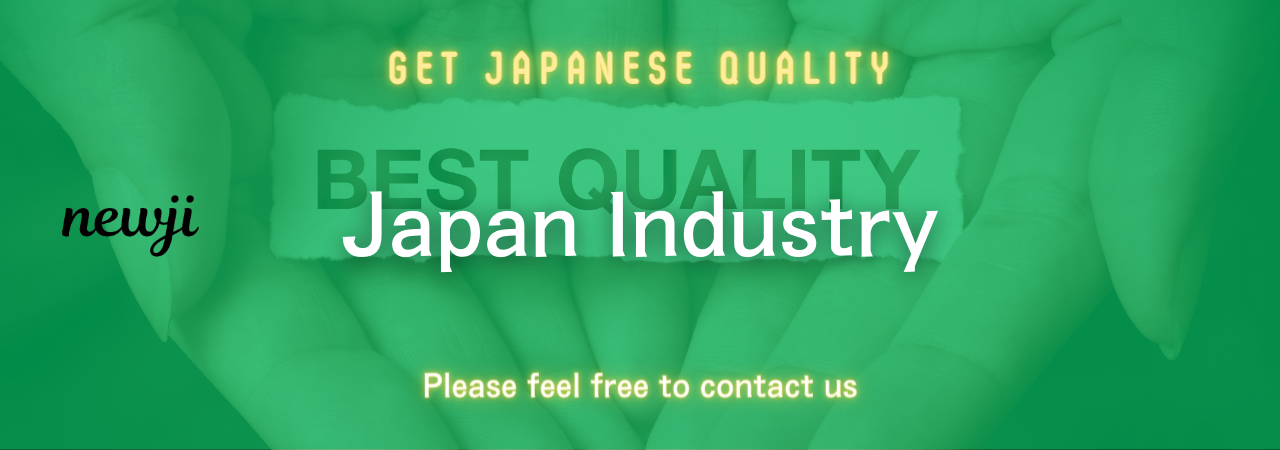
目次
What is Transfer Learning?
Transfer learning is a machine learning method where a model developed for a particular task is reused as a starting point for a model on a second task.
It is a popular approach in deep learning, where pre-trained models are often used for similar problems.
Think of it as a scenario where one day you’ve learned how to ride a bike, and the next, you’re able to learn how to ride a motorcycle much faster because some of the skills are transferable.
Transfer learning is beneficial because it requires fewer data for training, reduces training time, and is often more accurate.
The Basics of Deep Learning
Deep learning is a subset of machine learning characterized by its use of neural networks with many layers.
These models are capable of learning and making intelligent decisions on their own, surpassing traditional machine learning models in many aspects.
Deep learning works by mimicking the way the human brain operates, processing data with neurons organized in layers.
This intelligent structuring allows for better recognition of patterns in data, which makes deep learning techniques particularly successful in tasks such as image and speech recognition.
Transfer learning utilizes deep learning architectures to apply knowledge learned from previous tasks to novel ones.
This process enhances learning efficiency and effectiveness in solving complex problems.
How Transfer Learning Works
In transfer learning, a model’s knowledge from a base task is transferred to a target task.
There are several steps involved:
1. **Pre-train a Model:** Initially, a model is trained on a large, general dataset.
This model learns to extract basic features that are beneficial across various tasks.
2. **Fine-tuning:** The pre-trained model is then fine-tuned with data specifically from the target task.
This requires less data and computational resources since the model already knows the general features and only needs to adapt to the specificities of the new task.
3. **Feature Extraction:** Another approach is to use the pre-trained model as a feature extractor.
Instead of retraining, the pre-trained model processes new data and extracts useful features, which are then used by another model for the target task.
Applications of Transfer Learning
Transfer learning has vast applications across different industries and tasks:
Image Recognition
One of the most common uses of transfer learning is in image classification.
Pre-trained models like VGGNet, ResNet, and Inception are used for various tasks, such as identifying diseases in medical images, recognizing animals in wildlife photography, and categorizing products in online stores.
These models, pre-trained on large image databases, excel in tasks with smaller datasets by reusing learned representations of images.
NLP and Text Processing
In the realm of Natural Language Processing (NLP), transfer learning is also changing how we process and understand text data.
Models such as GPT-3 and BERT are pre-trained on vast amounts of text data and can be fine-tuned for specific tasks like sentiment analysis, translation, and text generation.
The ability to understand and leverage context from pre-trained models enhances performance on these tasks significantly.
Speech Recognition
Speech recognition tasks benefit from transfer learning by utilizing models originally trained on large speech datasets.
These pre-trained models can then be adapted to recognize specific accents or dialects with much less new data.
As a result, they offer efficient speech-to-text conversions across various languages and speaking styles.
Benefits of Transfer Learning
Here are some of the significant advantages of using transfer learning in deep learning models:
Improved Efficiency
By building on existing models, transfer learning saves time, effort, and computational resources.
Instead of training a model from scratch, developers start with a developed model and merely tweak it, which is much quicker.
Better Performance with Fewer Data
Transfer learning enables better performance even when the target dataset is small.
This is because the model uses information from a large dataset to understand complex data representations, which it can fine-tune for the smaller dataset of the target task.
Cost-Effective
Avoid the high costs of data labeling and extensive computational resources.
Transfer learning reduces the need for large amounts of labeled data, as the model already learned features in the pre-training stage.
Challenges in Transfer Learning
Even with its many benefits, transfer learning presents a few challenges:
Negative Transfer
Sometimes, transferring knowledge from one domain to another might not be beneficial and can lead to poorer performance than starting from scratch.
Careful selection of the source model and thorough testing is essential.
Choosing the Right Model
Selecting an appropriate model for transfer is crucial yet often challenging.
Different tasks and datasets require careful evaluation to ensure compatibility and effectiveness of the pre-trained model.
Conclusion
Transfer learning and its application in deep learning mark a significant advancement in machine learning capabilities.
By leveraging pre-trained models and adapting them for new tasks, we can improve learning efficiency, speed, and cost-effectiveness.
Despite some challenges, transfer learning remains a robust tool in developing intelligent systems capable of learning and performing complex tasks with fewer resources.
As research in this area continues to evolve, the potential for transfer learning across varied domains and industries is promising, paving the way for more sophisticated and integrated AI solutions.
資料ダウンロード
QCD管理受発注クラウド「newji」は、受発注部門で必要なQCD管理全てを備えた、現場特化型兼クラウド型の今世紀最高の受発注管理システムとなります。
ユーザー登録
受発注業務の効率化だけでなく、システムを導入することで、コスト削減や製品・資材のステータス可視化のほか、属人化していた受発注情報の共有化による内部不正防止や統制にも役立ちます。
NEWJI DX
製造業に特化したデジタルトランスフォーメーション(DX)の実現を目指す請負開発型のコンサルティングサービスです。AI、iPaaS、および先端の技術を駆使して、製造プロセスの効率化、業務効率化、チームワーク強化、コスト削減、品質向上を実現します。このサービスは、製造業の課題を深く理解し、それに対する最適なデジタルソリューションを提供することで、企業が持続的な成長とイノベーションを達成できるようサポートします。
製造業ニュース解説
製造業、主に購買・調達部門にお勤めの方々に向けた情報を配信しております。
新任の方やベテランの方、管理職を対象とした幅広いコンテンツをご用意しております。
お問い合わせ
コストダウンが利益に直結する術だと理解していても、なかなか前に進めることができない状況。そんな時は、newjiのコストダウン自動化機能で大きく利益貢献しよう!
(β版非公開)