- お役立ち記事
- GAN model structure and training method
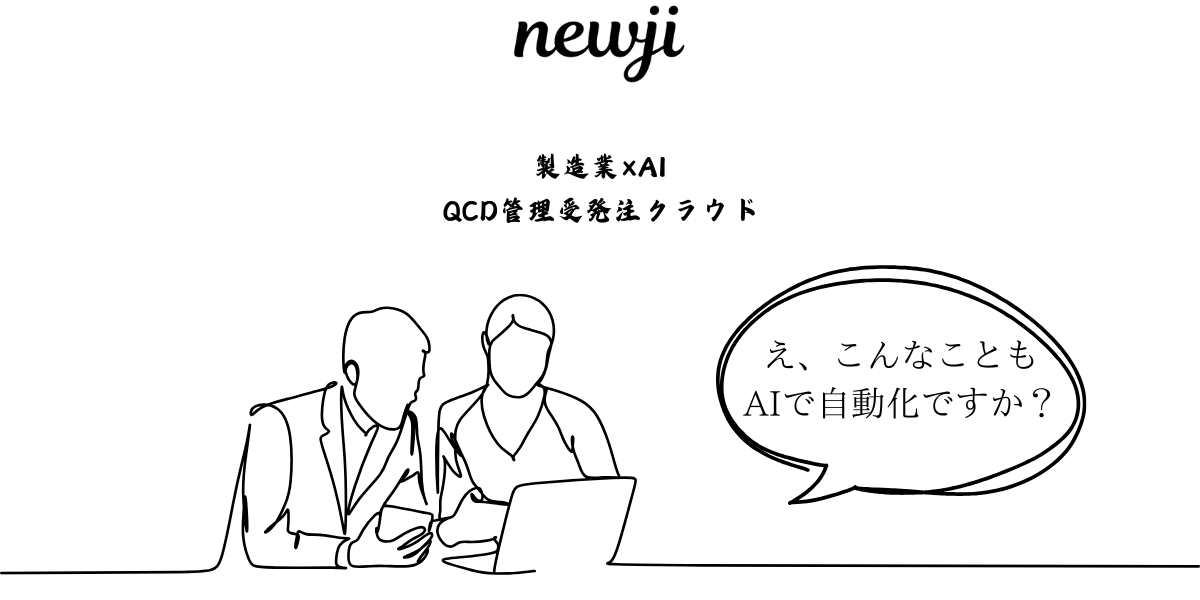
GAN model structure and training method
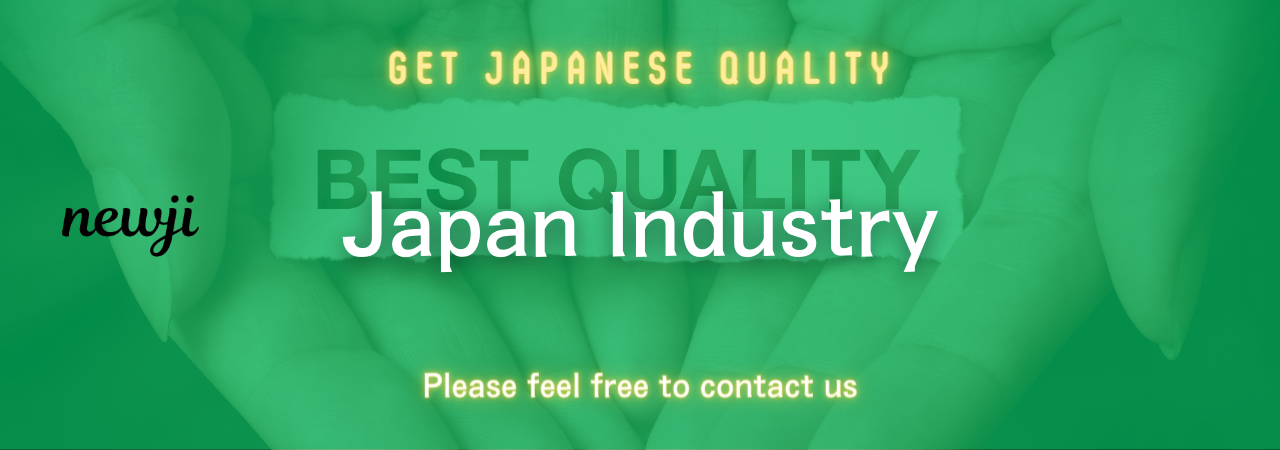
目次
Understanding GAN Model Structure
Generative Adversarial Networks (GANs) are a class of machine learning frameworks that have gained significant attention due to their ability to generate realistic data.
The structure of a GAN typically consists of two main components: the generator and the discriminator.
These two neural networks work together in a game-like setting, where both networks aim to improve their performance through continuous training.
The Role of the Generator
The generator is responsible for creating new data samples from random noise.
Its primary aim is to create data that is indistinguishable from real data.
To achieve this, the generator takes a random input and processes it through a deep neural network to produce an output that resembles the training data.
The quality of the output data depends on the architecture of the generator network and the efficiency of its training process.
The Function of the Discriminator
The discriminator’s role is to differentiate between real data and data generated by the generator.
It serves as a binary classifier that assesses whether a given data sample is genuine or fake.
The discriminator provides feedback to the generator by adjusting its weights based on how well it can tell the real data apart from the fake data.
This feedback is crucial for the generator to enhance its data generation capabilities over time.
How GANs Work: The Adversarial Process
GANs operate through an adversarial process resembling a competition between two players.
In this setting, the generator and discriminator are in constant opposition.
The generator strives to create data as convincing as possible, while the discriminator aims to accurately classify data as real or fake.
Training the GAN Through Iterations
GAN training involves a series of iterations where both networks are updated to improve their respective functions.
1. **Generator Improvement**: Initially, the generator produces samples that are likely recognized as fake by the discriminator.
However, during training, the generator learns to modify its output to increase the likelihood of fooling the discriminator.
2. **Discriminator Refinement**: Simultaneously, the discriminator enhances its capacity to discern real data from fake data by refining its own classification accuracy.
This refinement is achieved by penalizing errors in its predictions during each training cycle.
Over numerous training cycles, or epochs, both networks evolve, leading to improved data generation capabilities and more accurate discrimination.
Loss Functions in GANs
The effectiveness of a GAN model is often determined by its loss functions.
These functions measure the performance of both the generator and the discriminator during training.
The generator aims to minimize its loss, which relates to its success in deceiving the discriminator.
Conversely, the discriminator strives to minimize its own loss, representing its ability to detect fake data accurately.
Challenges in Training GANs
Despite their potential, GANs pose several training challenges that affect their efficiency and effectiveness.
These challenges need to be addressed to harness the full capabilities of GANs.
Mode Collapse
Mode collapse occurs when the generator produces a limited variety of outputs that only capture a subset of the data distribution.
This can lead to a lack of diversity in the generated data.
Addressing mode collapse requires fine-tuning the hyperparameters and architecture of the generator to ensure comprehensive data coverage.
Training Instability
Another common issue in GAN training is instability.
It happens when there is an imbalance between the capabilities of the generator and the discriminator.
If one of these networks outpaces the other, it can lead to unreliable training outcomes.
Researchers often adjust learning rates and utilize sophisticated architectures to maintain a balanced competition between the two networks.
Difficulties with Convergence
GANs can struggle to converge to an optimal solution during training.
Convergence issues arise from the complex interactions between the generator and discriminator.
To mitigate this problem, strategies such as implementing progressive learning, utilizing adaptive optimization, and incorporating constraints on network outputs are employed.
Improving GAN Training Methods
To enhance the training process of GANs, researchers have proposed various methods and enhancements.
These improvements aim to achieve stable and efficient training while enhancing the quality and diversity of generated data.
Use of Auxiliary Classifiers
Incorporating auxiliary classifiers into the GAN framework can provide additional guidance to the generator.
By sharing information across different samples, these classifiers can aid the GAN in generating higher-quality data with more diverse characteristics.
Deploying Wasserstein GANs
Wasserstein GANs (WGANs) offer a distinct approach to tackling convergence issues.
They modify the loss function to measure the ‘distance’ between the real and generated data distributions.
This change ensures smoother and more stable training, reducing mode collapse and improving the generator’s ability to capture the entire data distribution.
Progressive Growing of GANs
Progressive growing is a method that gradually increases the complexity of GAN models during training.
By commencing with lower resolution data and progressively introducing higher resolution data, this method enhances stability.
It allows the generator to develop the capability to produce detailed and highly realistic data over time.
The Real-World Applications of GANs
GANs find useful applications in various fields due to their ability to generate high-quality data.
From creating lifelike images to data augmentation, their potential impact is immense.
Image Generation and Enhancement
GANs are widely utilized for generating realistic images, which has applications in media, entertainment, and design.
They are also employed in image enhancement tasks such as super-resolution and inpainting, where missing parts of an image are realistically filled in.
Data Augmentation for Machine Learning
In machine learning, GANs are used for data augmentation by creating synthetic data samples.
This application is particularly beneficial in scenarios where real data is scarce or expensive to obtain, enabling models to train on a larger dataset for better performance.
Biometric and Medical Data Synthesis
GANs have promising applications in biometrics and the medical field.
They can be used to generate synthetic face images or medical scans, aiding research and development while preserving privacy and reducing the need for real sensitive data.
As we continue to refine GAN models and training methods, their capabilities and applications are likely to expand, driving innovation across various technological landscapes.
資料ダウンロード
QCD調達購買管理クラウド「newji」は、調達購買部門で必要なQCD管理全てを備えた、現場特化型兼クラウド型の今世紀最高の購買管理システムとなります。
ユーザー登録
調達購買業務の効率化だけでなく、システムを導入することで、コスト削減や製品・資材のステータス可視化のほか、属人化していた購買情報の共有化による内部不正防止や統制にも役立ちます。
NEWJI DX
製造業に特化したデジタルトランスフォーメーション(DX)の実現を目指す請負開発型のコンサルティングサービスです。AI、iPaaS、および先端の技術を駆使して、製造プロセスの効率化、業務効率化、チームワーク強化、コスト削減、品質向上を実現します。このサービスは、製造業の課題を深く理解し、それに対する最適なデジタルソリューションを提供することで、企業が持続的な成長とイノベーションを達成できるようサポートします。
オンライン講座
製造業、主に購買・調達部門にお勤めの方々に向けた情報を配信しております。
新任の方やベテランの方、管理職を対象とした幅広いコンテンツをご用意しております。
お問い合わせ
コストダウンが利益に直結する術だと理解していても、なかなか前に進めることができない状況。そんな時は、newjiのコストダウン自動化機能で大きく利益貢献しよう!
(Β版非公開)