- お役立ち記事
- Generative AI and Deep Learning Basics with RAG System Implementation
Generative AI and Deep Learning Basics with RAG System Implementation
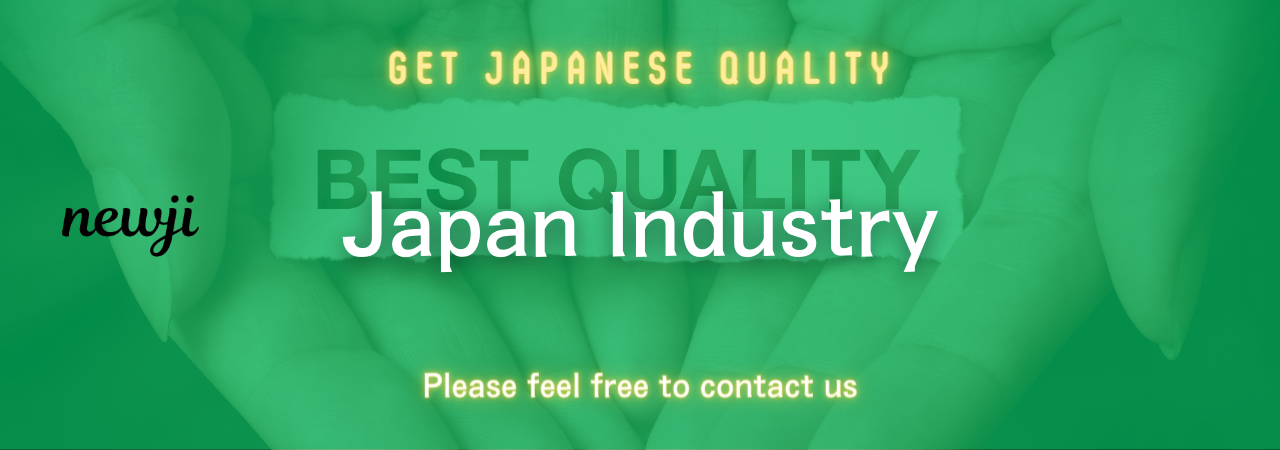
目次
Understanding Generative AI and Deep Learning
Generative AI has become a pivotal innovation in the field of artificial intelligence, enabling machines to create new data from learned representations.
Unlike traditional AI that focuses on analyzing or classifying existing data, generative AI generates new content that mimics the input data it was trained on.
Deep learning, a subset of machine learning, plays a crucial role in powering generative AI applications.
With the use of neural networks, deep learning enables machines to learn complex patterns in data and reproduce variations effectively.
Generative AI uses models like Generative Adversarial Networks (GANs) and Variational Autoencoders (VAEs) to create new data.
GANs consist of two networks—a generator and a discriminator—where the generator creates new data instances, while the discriminator evaluates them for authenticity.
This adversarial process helps in refining the output to be indistinguishable from real data.
VAEs, on the other hand, use encoding and decoding processes to understand data distribution and generate new samples.
Introduction to RAG Systems
Retrieval-Augmented Generation (RAG) is a promising approach that combines retrieval-based techniques with generative models, enhancing the quality and relevance of AI-generated content.
RAG systems leverage large datasets to retrieve information and then utilize generative models to refine and present this data effectively.
The integration of retrieval methods ensures that generative models have access to a vast array of information, allowing them to produce more accurate and relevant outputs.
In RAG systems, the retrieval phase involves searching through existing datasets to find pertinent information.
This is typically followed by the generation phase, where deep learning models synthesize the retrieved data into coherent and contextually appropriate outputs.
RAG systems are particularly useful in applications like question-answering, conversational agents, and information synthesis, where accuracy and relevance are paramount.
Implementing Deep Learning with RAG Systems
To implement a RAG system using deep learning techniques, one must follow a structured approach that combines retrieval and generation seamlessly.
The first step is to establish a robust retrieval mechanism capable of searching extensive datasets efficiently.
This can involve natural language processing (NLP) techniques to understand and interpret queries, as well as databases or knowledge graphs as the information source.
Once the retrieval mechanism is in place, the next step involves using a generative model to process the retrieved information.
Deep learning models like transformers, which excel in sequence-to-sequence tasks, are particularly suited for this purpose.
These models can take the retrieved data, understand its context, and generate responses or outputs that are both relevant and coherent.
Training a RAG system requires a significant amount of data and computational power.
The deep learning models must undergo extensive training to grasp the nuances of language and information distribution effectively.
Moreover, fine-tuning is essential to ensure that the retrieval and generation components work in harmony, producing outputs that meet the desired precision and creativity.
Applications of RAG Systems
RAG systems have a wide range of applications across various industries.
In healthcare, they can assist in compiling patient information and generating reports based on medical histories.
In academia, RAG systems can be used to summarize research papers, extracting vital information and presenting it succinctly.
In customer service, RAG systems enhance the capabilities of chatbots by providing relevant and accurate responses to customer inquiries.
By accessing a broad repository of information, these systems can address customer needs more effectively, resulting in improved satisfaction and engagement.
Moreover, RAG systems have been employed in content creation, empowering writers with resourceful tools for generating ideas and structuring articles.
This is particularly useful for creating domain-specific content where extensive background knowledge is required.
Challenges and Considerations
Despite the promising capabilities of RAG systems, there are several challenges and considerations to address.
One of the primary concerns is data privacy, especially when handling sensitive or proprietary information.
Implementing safeguards and ensuring compliance with data protection regulations is paramount to mitigate risks associated with data breaches.
Another challenge is ensuring the quality and bias-free nature of generated content.
RAG systems rely heavily on the underlying datasets, which may contain biases that need to be identified and corrected.
This requires continual monitoring and updating of both retrieval and generative models to maintain accuracy and fairness.
Scalability is also a significant consideration, as the extensive datasets and computational resources involved can be demanding.
Efforts to optimize the efficiency of RAG systems, such as utilizing cloud-based infrastructure and parallel processing, can aid in achieving scalability without compromising performance.
Conclusion
Generative AI and deep learning have transformed the capabilities of artificial intelligence, with RAG systems offering an innovative approach to integrating relevance and creativity in machine-generated content.
As the technology continues to evolve, its applications and impact across different sectors are expected to grow exponentially.
Understanding the fundamentals of generative AI, deep learning, and RAG systems is crucial for professionals seeking to harness these advanced capabilities.
With continued advancements and a focus on overcoming existing challenges, RAG systems hold the promise of delivering more intelligent and reliable solutions across a multitude of fields.
資料ダウンロード
QCD調達購買管理クラウド「newji」は、調達購買部門で必要なQCD管理全てを備えた、現場特化型兼クラウド型の今世紀最高の購買管理システムとなります。
ユーザー登録
調達購買業務の効率化だけでなく、システムを導入することで、コスト削減や製品・資材のステータス可視化のほか、属人化していた購買情報の共有化による内部不正防止や統制にも役立ちます。
NEWJI DX
製造業に特化したデジタルトランスフォーメーション(DX)の実現を目指す請負開発型のコンサルティングサービスです。AI、iPaaS、および先端の技術を駆使して、製造プロセスの効率化、業務効率化、チームワーク強化、コスト削減、品質向上を実現します。このサービスは、製造業の課題を深く理解し、それに対する最適なデジタルソリューションを提供することで、企業が持続的な成長とイノベーションを達成できるようサポートします。
オンライン講座
製造業、主に購買・調達部門にお勤めの方々に向けた情報を配信しております。
新任の方やベテランの方、管理職を対象とした幅広いコンテンツをご用意しております。
お問い合わせ
コストダウンが利益に直結する術だと理解していても、なかなか前に進めることができない状況。そんな時は、newjiのコストダウン自動化機能で大きく利益貢献しよう!
(Β版非公開)