- お役立ち記事
- How to develop low-noise fans based on fan noise generation mechanism and machine learning
月間76,176名の
製造業ご担当者様が閲覧しています*
*2025年3月31日現在のGoogle Analyticsのデータより
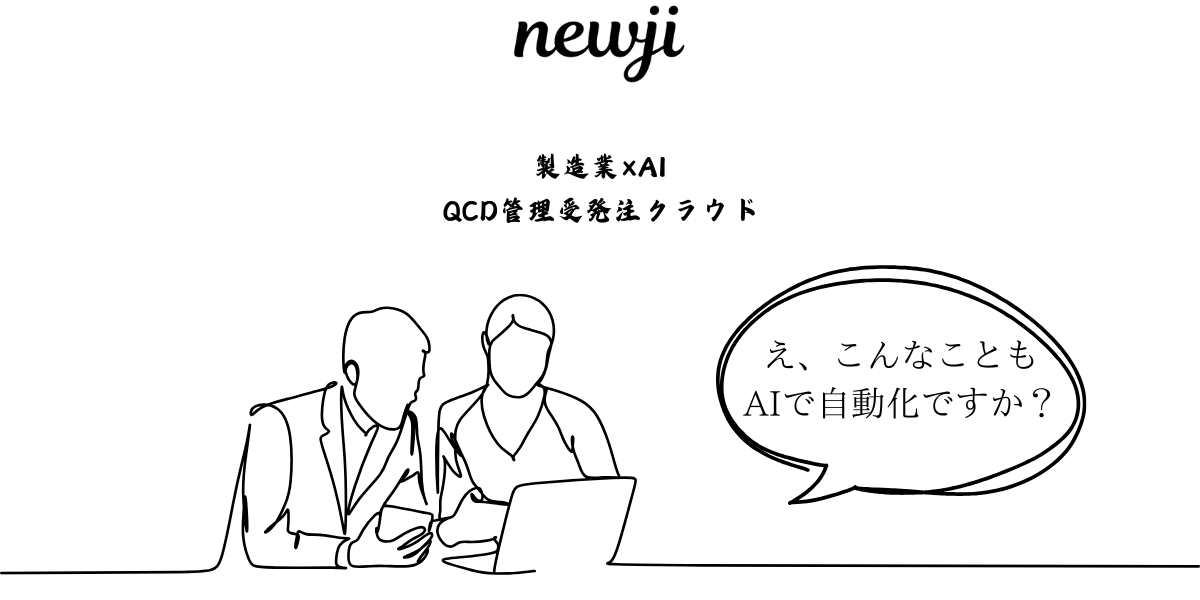
How to develop low-noise fans based on fan noise generation mechanism and machine learning
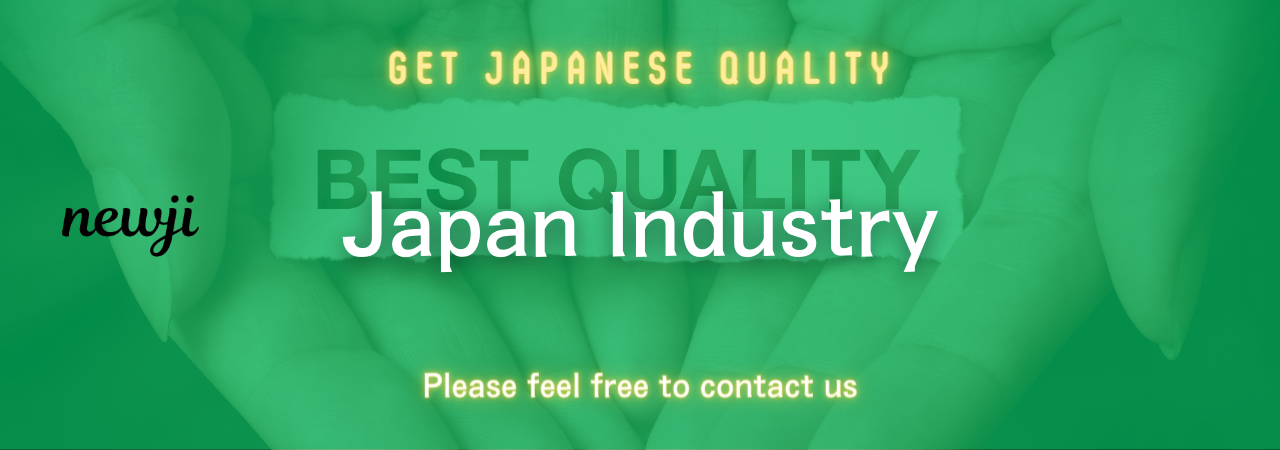
目次
Understanding Fan Noise Generation
The development of low-noise fans is crucial for enhancing user experience in various applications, from computer cooling systems to household appliances.
To achieve this, it’s essential to first understand how fan noise is generated.
Fan noise primarily arises from aerodynamic and mechanical sources.
Aerodynamic noise is the result of the interaction between the fan blades and the air they move.
This includes turbulence, vortex-shedding, and blade vortex interactions.
On the other hand, mechanical noise comes from the vibrations and resonance of fan components.
Both types of noise can significantly affect the overall noise level produced by a fan.
By analyzing these noise-generation mechanisms, engineers can identify key areas for improvement, leading to the design of quieter fans.
Exploring Machine Learning in Fan Design
The integration of machine learning into fan design offers new possibilities for reducing noise.
Machine learning algorithms can handle complex datasets and identify patterns that may not be immediately obvious through traditional analytical methods.
In the context of fan design, machine learning can help in several ways.
Firstly, it can assist in optimizing fan geometry by analyzing various design parameters such as blade shape, size, and pitch.
By simulating different designs and evaluating their acoustic performance, machine learning can recommend design solutions that minimize noise.
Secondly, machine learning models can be trained to predict noise levels based on different operational conditions.
This predictive capability allows designers to foresee potential noise issues and make necessary adjustments during the design phase, rather than after production.
Steps to Develop Low-Noise Fans Using Machine Learning
Developing low-noise fans using machine learning involves several systematic steps.
Data Collection and Preparation
Before machine learning models can be applied, extensive data collection is essential.
This data can include acoustic measurements from existing fan models, material properties, and operational conditions.
Ensuring high-quality, clean data is crucial, as machine learning models are only as good as the data they are trained on.
Data preprocessing helps to clean and organize the data, removing any inconsistencies or irrelevant information.
This step ensures that the models trained are efficient and reliable.
Choosing the Right Machine Learning Model
There are several types of machine learning models that can be considered, such as regression models, neural networks, or decision trees.
The choice of model depends on the specific requirements of the fan design project.
For example, regression models are suitable for predicting continuous noise levels, while classification models might be used to categorize fan designs into different noise categories.
Model Training and Validation
Once the model is chosen, the next step is training using the collected data.
During training, the model learns to identify the relationships between different design parameters and the resulting noise levels.
It’s important to also validate the model using a separate dataset to evaluate its accuracy and prevent overfitting.
This step ensures that the model can generalize its predictions to new, unseen data effectively.
Optimization and Testing
With a trained model in place, optimization algorithms can be used to explore various design alternatives.
The aim is to find a design that achieves the lowest possible noise level while maintaining performance.
After optimization, prototypes of the new low-noise fan designs are created and subjected to testing in real-world conditions.
Testing provides valuable feedback and ensures that the designs meet the desired noise reduction goals.
Challenges and Considerations
While machine learning offers a promising approach to reducing fan noise, there are challenges and considerations to keep in mind.
Data Quality and Availability
One of the significant challenges is having access to high-quality and diverse data.
Data scarcity or poor-quality data can lead to inaccurate model predictions.
Collaboration between manufacturers and researchers can help build comprehensive datasets necessary for effective machine learning applications.
Computational Resources
Machine learning, particularly for complex models like neural networks, can be computationally intensive.
Designers need access to sufficient computational resources to train and run models effectively.
Cloud computing solutions may offer an alternative by providing on-demand processing power.
Balancing Noise Reduction with Performance
In the pursuit of lower noise levels, it’s essential not to compromise the fan’s performance.
The balance between noise and efficiency must be carefully maintained.
Machine learning can help identify this equilibrium by examining the trade-offs involved.
The Future of Low-Noise Fan Development
The future of fan design, utilizing the synergy of noise generation understanding and machine learning, holds great promise.
As technology advances, we can expect more sophisticated models that offer even greater precision in designing ultra-quiet fans.
Additionally, continuous improvements in computational power and machine learning algorithms will make these tools more accessible to a wider range of engineers and designers.
Ultimately, the result will be fans that not only perform their function quietly but also enhance the comfort and efficiency across various applications, contributing positively to modern living and working environments.
資料ダウンロード
QCD管理受発注クラウド「newji」は、受発注部門で必要なQCD管理全てを備えた、現場特化型兼クラウド型の今世紀最高の受発注管理システムとなります。
ユーザー登録
受発注業務の効率化だけでなく、システムを導入することで、コスト削減や製品・資材のステータス可視化のほか、属人化していた受発注情報の共有化による内部不正防止や統制にも役立ちます。
NEWJI DX
製造業に特化したデジタルトランスフォーメーション(DX)の実現を目指す請負開発型のコンサルティングサービスです。AI、iPaaS、および先端の技術を駆使して、製造プロセスの効率化、業務効率化、チームワーク強化、コスト削減、品質向上を実現します。このサービスは、製造業の課題を深く理解し、それに対する最適なデジタルソリューションを提供することで、企業が持続的な成長とイノベーションを達成できるようサポートします。
製造業ニュース解説
製造業、主に購買・調達部門にお勤めの方々に向けた情報を配信しております。
新任の方やベテランの方、管理職を対象とした幅広いコンテンツをご用意しております。
お問い合わせ
コストダウンが利益に直結する術だと理解していても、なかなか前に進めることができない状況。そんな時は、newjiのコストダウン自動化機能で大きく利益貢献しよう!
(β版非公開)