- お役立ち記事
- How to predict damage occurrence using AI
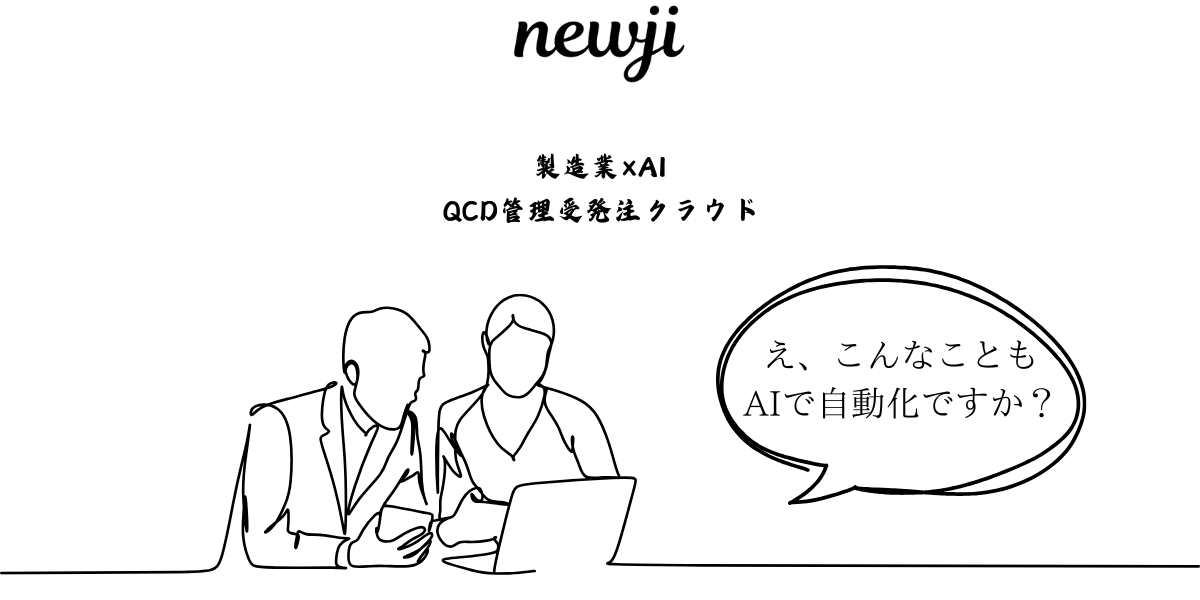
How to predict damage occurrence using AI
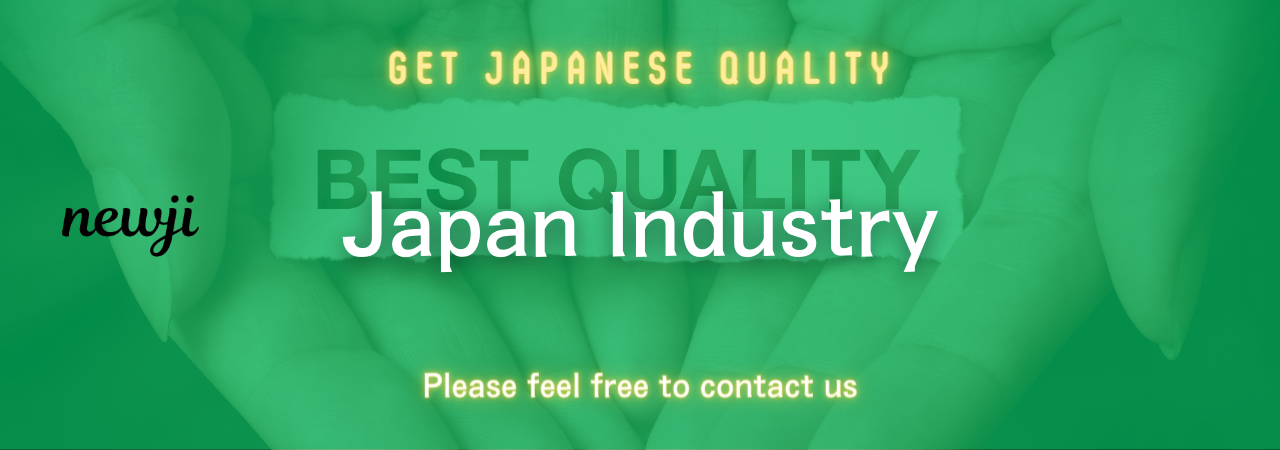
目次
Understanding AI and Damage Prediction
Artificial Intelligence (AI) has permeated various sectors, revolutionizing how we approach problems and develop solutions.
One intriguing application is the prediction of damage occurrence.
This technique employs AI to foresee potential damages before they happen, effectively saving resources and enhancing safety.
But how exactly does AI predict damages, and why is it important?
To start, AI leverages vast amounts of data to predict damage occurrences by analyzing patterns and trends.
Using algorithms and models, AI systems can identify correlations and cause-effect relationships that may not be immediately apparent to human observers.
These insights can then be applied to forecast potential damages in a range of contexts—from infrastructure and machinery to natural disasters and environmental degradation.
How AI Works in Damage Prediction
The process begins with data collection.
An AI system requires substantial and relevant data to function accurately.
This can include historical data, real-time sensor data, maintenance records, weather patterns, and more, depending on the specific application.
Once the data is gathered, it is processed and preprocessed.
This step involves organizing the data, cleaning it to remove inaccuracies, and converting it into a format suitable for analysis.
The data is then fed into AI models, which use machine learning techniques to analyze and learn from it.
Machine learning algorithms are integral to AI’s predictive abilities.
These algorithms identify patterns in the data and use them to make predictions about future events.
For instance, in predicting machinery failure, an AI system might look for patterns in temperature fluctuations, vibration levels, or operational speed that have historically led to damage.
In the case of natural disasters, AI systems might analyze weather data, seismic activity records, and geographic information to predict the likelihood of events such as floods or earthquakes.
By assessing the probability and potential severity of such events, AI helps in planning and mitigating possible damage.
Types of AI Used in Damage Prediction
There are several types of AI technologies employed in damage prediction, each with its strengths and suitable applications.
1. **Machine Learning (ML):** This is a core AI technique that enables systems to learn from data and improve over time.
ML models can adapt and refine their predictions continually as they are exposed to more data, making them ideal for dynamic environments.
2. **Deep Learning:** This is a subset of machine learning that uses neural networks to mimic human decision-making processes.
Deep learning is particularly effective for complex prediction tasks, such as image and speech recognition, which can also be applied in identifying defects from images or sensor data.
3. **Natural Language Processing (NLP):** While not always directly used in damage prediction, NLP can analyze text data (e.g., maintenance logs or reports) to extract valuable insights that contribute to predicting potential damage.
Real-World Applications of AI in Damage Prediction
The utility of AI in predicting damage is already making significant impacts across various industries.
Infrastructure and Construction
AI plays a crucial role in monitoring the structural health of buildings, bridges, and roads.
By continuously analyzing data from various sensors, AI systems can predict where and when infrastructure might fail.
This ability aids in proactive maintenance, ensuring safety and extending the lifespan of costly infrastructure.
Manufacturing
In manufacturing, predictive maintenance is a game-changer.
AI systems monitor machinery to detect any deviations from normal operation that could indicate impending failure.
This allows manufacturers to perform maintenance before any actual damage occurs, minimizing downtime and reducing repair costs.
Environmental Monitoring
AI is also used in environmental monitoring to predict and prevent damage caused by natural disasters.
By analyzing meteorological data and environmental indicators, AI systems can forecast events like floods, landslides, or hurricanes.
This early warning enables communities to prepare, ultimately decreasing human and material losses.
Automotive and Aviation
In the automotive and aviation industries, AI can predict vehicle and aircraft component failures.
Constant monitoring and data analysis help identify wear and tear, ensuring that maintenance can be scheduled before any critical damage.
Benefits of Using AI for Damage Prediction
The advantages of utilizing AI for predicting damage occurrences are substantial.
– **Improved Safety:** By predicting potential failures, AI enhances the safety of operations in industries ranging from aviation to infrastructure.
– **Cost Efficiency:** Early identification of issues allows for timely maintenance, preventing costly repairs or replacements, and reducing operational downtime.
– **Resource Optimization:** Companies can allocate resources more efficiently, focusing on the most critical areas needing attention.
– **Enhanced Decision-Making:** AI provides actionable insights that contribute to better strategic planning and decision-making.
Challenges and Future Prospects
Despite its benefits, implementing AI for damage prediction is not without challenges.
High-quality data is essential for accurate predictions, and gathering this data can be costly and time-consuming.
Additionally, AI systems must be continually updated and maintained, as outdated models can result in inaccurate forecasts.
Looking forward, AI technology for damage prediction shows great promise.
As data collection becomes more efficient and algorithms increasingly sophisticated, AI’s predictive capabilities will only improve.
Moreover, advancements in AI research, such as improved interpretability of AI decisions, will encourage broader adoption across different sectors.
In conclusion, predicting damage occurrences with AI is a powerful application that enhances safety and efficiency across industries.
As technology evolves, its potential to prevent damage and optimize operations will continue to be realized, ushering in a new era of predictive maintenance and risk management.
資料ダウンロード
QCD調達購買管理クラウド「newji」は、調達購買部門で必要なQCD管理全てを備えた、現場特化型兼クラウド型の今世紀最高の購買管理システムとなります。
ユーザー登録
調達購買業務の効率化だけでなく、システムを導入することで、コスト削減や製品・資材のステータス可視化のほか、属人化していた購買情報の共有化による内部不正防止や統制にも役立ちます。
NEWJI DX
製造業に特化したデジタルトランスフォーメーション(DX)の実現を目指す請負開発型のコンサルティングサービスです。AI、iPaaS、および先端の技術を駆使して、製造プロセスの効率化、業務効率化、チームワーク強化、コスト削減、品質向上を実現します。このサービスは、製造業の課題を深く理解し、それに対する最適なデジタルソリューションを提供することで、企業が持続的な成長とイノベーションを達成できるようサポートします。
オンライン講座
製造業、主に購買・調達部門にお勤めの方々に向けた情報を配信しております。
新任の方やベテランの方、管理職を対象とした幅広いコンテンツをご用意しております。
お問い合わせ
コストダウンが利益に直結する術だと理解していても、なかなか前に進めることができない状況。そんな時は、newjiのコストダウン自動化機能で大きく利益貢献しよう!
(Β版非公開)