- お役立ち記事
- Image feature extraction by matrix decomposition
Image feature extraction by matrix decomposition
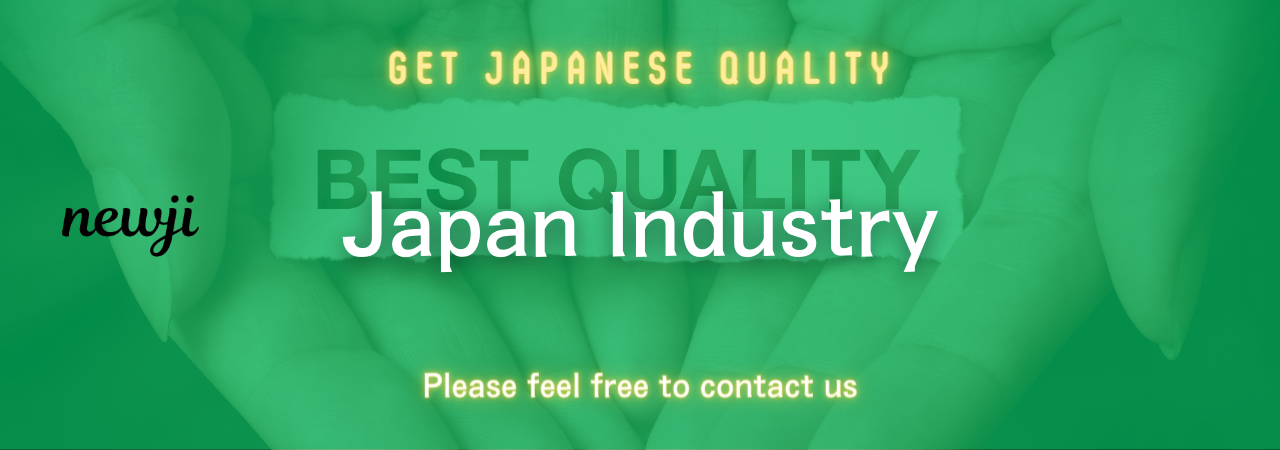
目次
Understanding Image Feature Extraction
Image feature extraction is a crucial component of image processing and computer vision.
It allows us to transform and manipulate images in order to retrieve meaningful information.
One of the advanced techniques utilized for feature extraction is matrix decomposition.
By breaking down the image data into simpler parts, matrix decomposition helps in identifying the most important features.
This method has a wide range of applications, from enhancing image recognition systems to improving data compression.
Basics of Matrix Decomposition
Matrix decomposition, also known as matrix factorization, involves breaking down a matrix into multiple components.
For image data, this matrix represents pixel values and color intensities.
The decomposition of this matrix can simplify complex operations, making it easier to identify patterns or key features within the image.
There are several methods of matrix decomposition, but some of the most common ones include Singular Value Decomposition (SVD), Principal Component Analysis (PCA), and Non-negative Matrix Factorization (NMF).
Singular Value Decomposition (SVD)
SVD is one of the most prominent techniques used for matrix decomposition.
It expresses a matrix as a product of three other matrices.
The primary goal of SVD in image processing is to reduce noise, improve clarity, and compress data without compromising vital information.
Through this decomposition, the most significant features of the image can be identified, making it easier to process and analyze.
Principal Component Analysis (PCA)
PCA is another powerful tool in feature extraction.
It focuses on reducing the dimensionality of the data while maintaining the variance.
This technique uses eigendecomposition which separates data into a few principal components.
These components represent the data in a simplified way, eliminating unnecessary details and noise.
In the context of images, PCA can enhance recognition tasks by focusing only on the essential components.
Non-negative Matrix Factorization (NMF)
NMF is a method that constrains factors to be non-negative in a matrix decomposition.
This non-negativity property is particularly useful for image feature extraction because images naturally have non-negative values.
By using NMF, the hidden patterns within the image become more accessible, improving the efficiency of extracting relevant features.
This technique is widely used in facial recognition and image segmentation.
Applications of Matrix Decomposition in Image Processing
Matrix decomposition has several applications and benefits in the field of image processing.
One of the primary uses is in image recognition, where it helps machines discern objects and patterns within images.
By identifying the critical features, the system becomes more adept at recognizing and classifying images.
Additionally, matrix decomposition assists in data compression by breaking down an image into its fundamental components.
This leads to more effective storage and retrieval of image data without significant loss of quality.
Image enhancement is another area where matrix decomposition proves useful.
Using techniques like SVD, noise can be reduced, and image contrast can be enhanced.
This allows for clearer, sharper images, which are important for better interpretation and analysis.
Moreover, matrix decomposition is also used in image forecasting and prediction.
By understanding the patterns within an image, computational models can predict changes over time.
This is particularly valuable in satellite imaging or medical imaging, where anticipating changes can lead to proactive measures.
Challenges and Considerations
While matrix decomposition offers numerous advantages in feature extraction, it also comes with challenges.
The computational complexity can be significant, especially with large datasets or high-resolution images.
This often requires powerful processing capabilities and can be time-consuming.
Selecting the appropriate technique for matrix decomposition is another challenge.
Different images and applications might require different methods or combinations to achieve the best results.
Therefore, understanding the nature of the image data and the desired outcome is crucial in choosing the right approach.
Another consideration is the trade-off between accuracy and efficiency.
While decomposing into more components might give more accurate reconstructions, it can also lead to overfitting.
Balancing accuracy with efficiency is essential to ensure practical and effective image processing.
The Future of Image Feature Extraction
As technology continues to evolve, feature extraction through matrix decomposition will see further advancements.
Improved algorithms and computing power will aid in overcoming current challenges, making image processing faster and more accurate.
Integration of artificial intelligence and deep learning with matrix decomposition techniques holds the potential for groundbreaking developments.
These advances could revolutionize fields like autonomous vehicles, predictive maintenance, and medical diagnostics.
Matrix decomposition will remain a fundamental aspect of image processing, continually contributing to our ability to understand and manipulate visual data effectively.
This field’s ongoing research and development promise even more efficient, accurate, and innovative solutions for extracting valuable insights from images.
資料ダウンロード
QCD調達購買管理クラウド「newji」は、調達購買部門で必要なQCD管理全てを備えた、現場特化型兼クラウド型の今世紀最高の購買管理システムとなります。
ユーザー登録
調達購買業務の効率化だけでなく、システムを導入することで、コスト削減や製品・資材のステータス可視化のほか、属人化していた購買情報の共有化による内部不正防止や統制にも役立ちます。
NEWJI DX
製造業に特化したデジタルトランスフォーメーション(DX)の実現を目指す請負開発型のコンサルティングサービスです。AI、iPaaS、および先端の技術を駆使して、製造プロセスの効率化、業務効率化、チームワーク強化、コスト削減、品質向上を実現します。このサービスは、製造業の課題を深く理解し、それに対する最適なデジタルソリューションを提供することで、企業が持続的な成長とイノベーションを達成できるようサポートします。
オンライン講座
製造業、主に購買・調達部門にお勤めの方々に向けた情報を配信しております。
新任の方やベテランの方、管理職を対象とした幅広いコンテンツをご用意しております。
お問い合わせ
コストダウンが利益に直結する術だと理解していても、なかなか前に進めることができない状況。そんな時は、newjiのコストダウン自動化機能で大きく利益貢献しよう!
(Β版非公開)