- お役立ち記事
- Image recognition technology using convolutional neural networks and its application to high-precision visual inspection
Image recognition technology using convolutional neural networks and its application to high-precision visual inspection
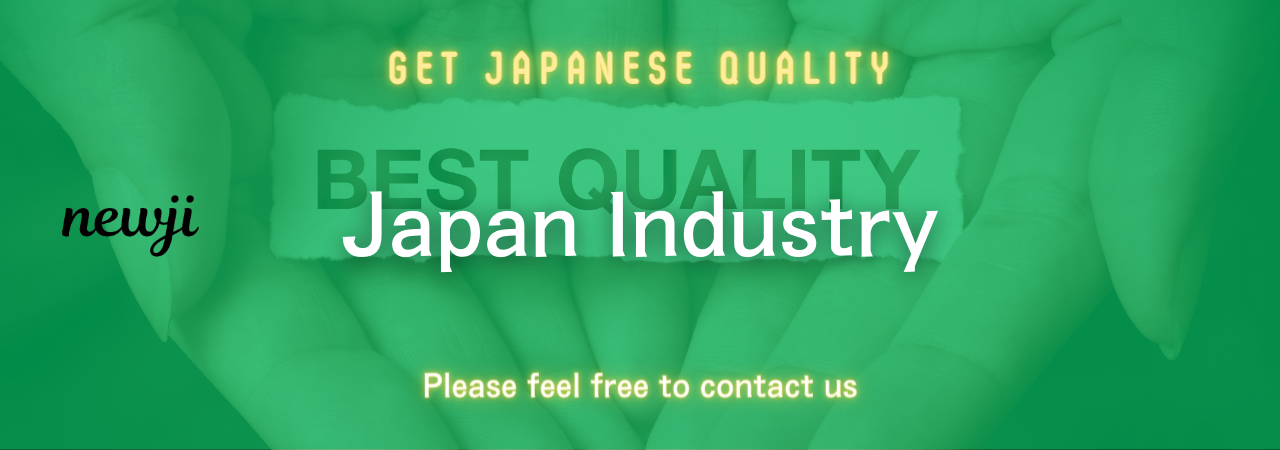
目次
Introduction to Image Recognition Technology
Image recognition technology has significantly evolved over the past few decades, largely thanks to advancements in machine learning and deep learning.
At the heart of these breakthroughs lies convolutional neural networks (CNNs), which have revolutionized the way machines understand visual data.
This technology mimics the human brain’s method of processing images, allowing machines to recognize, classify, and even understand images with remarkable precision.
In recent years, CNNs have become the cornerstone for various applications, especially in fields requiring high-precision visual inspection.
From quality control in manufacturing to innovative medical imaging techniques, the applications of CNN-based image recognition are immense.
Understanding Convolutional Neural Networks
Convolutional neural networks are a specialized class of artificial neural networks designed for processing structured grid data, such as images.
Unlike traditional neural networks, CNNs are designed to take advantage of the spatial structure of an image, making them particularly effective in image recognition tasks.
A CNN consists of several layers, each serving a unique purpose.
The initial layers deal with simple image features like edges and corners, while deeper layers focus on complex aspects such as shapes and objects.
The key components of CNNs include convolutional layers, pooling layers, and fully connected layers, each working in harmony to analyze an image comprehensively.
The convolutional layer is the core building block of a CNN.
It applies filters (also known as kernels) to the input image, producing feature maps that highlight specific patterns.
Pooling layers help in reducing the spatial size of these feature maps, conserving computational resources while retaining important information.
Finally, fully connected layers are used to make predictions based on the extracted features.
The Power of CNNs in Image Recognition
CNNs are exceptionally powerful in image recognition due to their ability to learn hierarchies of features.
This means they can automatically capture intricate details of images without manual feature extraction, leading to more accurate results.
Moreover, CNNs are highly scalable, allowing them to handle large and complex datasets effectively.
The robustness of CNNs in handling variations in images, such as changes in lighting, orientation, and scale, further underscores their superiority in image recognition tasks.
This resilience comes from their use of a sliding window (convolution) approach, which ensures that small variations in the image do not lead to incorrect classifications.
Applications in High-Precision Visual Inspection
The application of CNNs in image recognition has paved the way for significant improvements in high-precision visual inspection.
Industries such as manufacturing, healthcare, and security have greatly benefited from these advancements.
Manufacturing and Quality Control
In manufacturing, maintaining high standards of quality control is crucial.
CNNs have been employed to automate the inspection processes, allowing for real-time monitoring and detection of defects in products.
For instance, in electronics manufacturing, CNNs can identify minute defects in circuit boards that might be invisible to the human eye.
This ensures that only products that meet strict quality criteria proceed to the next stage of production, reducing waste and enhancing efficiency.
Healthcare and Medical Imaging
In healthcare, CNNs have revolutionized medical imaging techniques.
They are used to improve the accuracy of diagnostics by processing complex medical images like MRIs and CT scans.
CNN-based models can detect abnormalities such as tumors or fractures with high precision, sometimes outperforming human radiologists in terms of speed and accuracy.
By learning from vast datasets of medical images, CNNs provide invaluable assistance to medical professionals, enabling earlier diagnosis and treatment of various conditions.
This leads to better patient outcomes and helps alleviate the burden on healthcare systems.
Security and Surveillance
In the realm of security, image recognition using CNNs plays a pivotal role in facial recognition systems.
These systems are employed in surveillance to automatically identify and verify individuals, enhancing security measures in sensitive areas such as airports and public events.
CNNs enable these systems to function in real-time, offering swift and reliable recognition even in crowded environments.
Challenges and Future Directions
Despite the remarkable capabilities of CNNs, several challenges remain that need addressing to further enhance their efficiency and accuracy.
Training CNNs requires large datasets and considerable computational resources, which might not be readily available in all scenarios.
Moreover, there is a need for more interpretability and transparency in CNN models to understand how they arrive at specific decisions.
The future of CNNs in image recognition looks promising, with ongoing research aimed at making these models more efficient and accessible.
Techniques such as transfer learning, which allows models to leverage pre-existing knowledge from related tasks, and the development of lighter architectures like MobileNets, could pave the way for CNNs to be used effectively even in resource-constrained environments.
Conclusion
Image recognition technology using convolutional neural networks has dramatically advanced the field of visual inspection, offering high precision and reliability across various applications.
From ensuring quality in manufacturing to advancing medical diagnostics and enhancing security, the impact of CNNs is profound and far-reaching.
As technology continues to evolve, the integration of CNNs into more applications is inevitable.
With further research and development, CNNs are set to become even more powerful tools, driving innovations across numerous industries and contributing to a future where artificial intelligence continues to assist and augment human capabilities.
資料ダウンロード
QCD調達購買管理クラウド「newji」は、調達購買部門で必要なQCD管理全てを備えた、現場特化型兼クラウド型の今世紀最高の購買管理システムとなります。
ユーザー登録
調達購買業務の効率化だけでなく、システムを導入することで、コスト削減や製品・資材のステータス可視化のほか、属人化していた購買情報の共有化による内部不正防止や統制にも役立ちます。
NEWJI DX
製造業に特化したデジタルトランスフォーメーション(DX)の実現を目指す請負開発型のコンサルティングサービスです。AI、iPaaS、および先端の技術を駆使して、製造プロセスの効率化、業務効率化、チームワーク強化、コスト削減、品質向上を実現します。このサービスは、製造業の課題を深く理解し、それに対する最適なデジタルソリューションを提供することで、企業が持続的な成長とイノベーションを達成できるようサポートします。
オンライン講座
製造業、主に購買・調達部門にお勤めの方々に向けた情報を配信しております。
新任の方やベテランの方、管理職を対象とした幅広いコンテンツをご用意しております。
お問い合わせ
コストダウンが利益に直結する術だと理解していても、なかなか前に進めることができない状況。そんな時は、newjiのコストダウン自動化機能で大きく利益貢献しよう!
(Β版非公開)