- お役立ち記事
- Image recognition troubles caused by deepfake images and adversarial samples and key points of countermeasure techniques
Image recognition troubles caused by deepfake images and adversarial samples and key points of countermeasure techniques
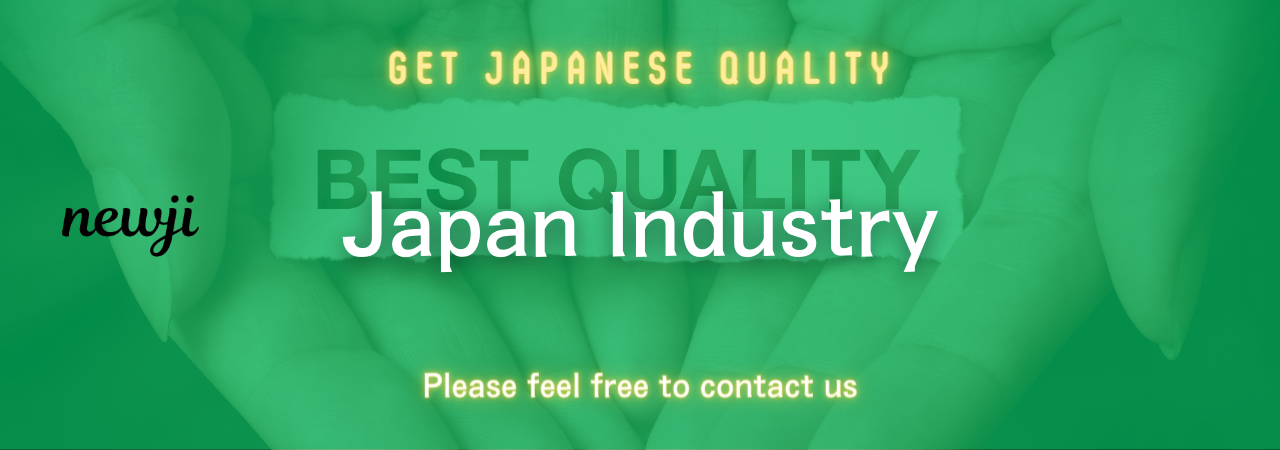
目次
Understanding Deepfake Images
Deepfake images have gained significant attention in recent years.
They are synthetic media where an image or video is manipulated to appear as someone else by using artificial intelligence (AI) technologies.
These creations can be shockingly convincing, often making it difficult to differentiate between what is real and what is fake.
The implications of such technology are vast, covering everything from entertainment to misinformation campaigns.
The technology behind deepfakes involves using deep learning algorithms, particularly Generative Adversarial Networks (GANs).
These networks serve as the backbone for creating highly realistic images by constantly improving the fake images through feedback loops.
One neural network creates the fake image, while another evaluates its authenticity.
This continuous process sharpens the fake image until it convincingly mimics reality.
What Are Adversarial Samples?
Adversarial samples are another aspect of image recognition troubles.
These are inputs intentionally designed to confuse AI models.
By introducing small perturbations to an image, it’s possible to trick an AI into misclassifying it.
Though the changes might be imperceptible to the human eye, they can heavily impact AI systems, leading to potential vulnerabilities in various applications, from security systems to autonomous vehicles.
These adversarial samples pose serious challenges because their primary goal is to exploit the weaknesses of neural networks.
The manipulations are often subtle, calculated distortions that shift the input data just enough to cause an AI system to make errors in its interpretations.
Challenges Posed by Deepfake and Adversarial Images
The advent of deepfake and adversarial technologies poses distinct challenges to image recognition systems.
Trust in digital media has been diminished due to the ease with which it can be altered.
These technologies can be used for harmful purposes, such as misinformation campaigns, fraud, and violations of privacy.
This erodes public trust and increases the risk of malicious activities going unchecked.
For adversarial samples, the stakes are just as high.
Consider the risk in autonomous vehicles that rely heavily on image recognition systems to navigate.
An adversarial sample directed at these systems could potentially lead to misinterpretations of crucial visual cues, causing catastrophic consequences.
Furthermore, adversarial attacks can also impact biometric security systems like facial recognition technology.
These systems must remain resilient to adversarial inputs to maintain security and operational effectiveness in public and private sectors.
Countermeasure Techniques
To combat the threats posed by deepfake and adversarial technologies, several countermeasures have been developed.
These countermeasures strive to enhance the robustness and reliability of image recognition systems.
Detection of Deepfake Images
Developing systems that can effectively identify deepfake images is paramount in curbing their threat.
Typically, detection algorithms analyze inconsistencies in facial movements or artifacts created during the generation process.
Researchers are using AI to identify these subtle irregularities, improving the accuracy of detection methods.
Furthermore, blockchain technology is emerging as a potential solution by providing a transparent and immutable way of verifying the authenticity of digital media.
It’s being explored to create a digital fingerprint for original content, ensuring that any alterations are quickly detectable.
Defending Against Adversarial Samples
Robustness against adversarial samples requires strengthening AI model defenses.
Some methods include adversarial training, where models are continually exposed to adversarial examples during training.
This process helps the models learn how to recognize and counter these trick inputs effectively.
Another approach is the use of defensive distillation, a technique that simplifies and stabilizes the model to reduce sensitivity against adversarial perturbations.
Additionally, researchers are working on developing adaptive algorithms that dynamically learn and adjust their responses in real-time to potential adversarial threats.
Collaboration and Legislation
Technological approaches alone may not suffice.
Collaboration between stakeholders, including government, industry leaders, and academic researchers, is vital to establish standardized protocols and share knowledge on effective defense measures.
Legislative measures can also play a significant role.
Implementing legal frameworks to regulate the misuse of deepfake technology and adversarial attacks can act as a deterrent.
Clear laws and consequences can help mitigate the malicious use of these technologies, fostering a safer digital environment.
The Road Ahead
Navigating the complexities of deepfake images and adversarial samples requires ongoing vigilance and innovation.
As technologies evolve, so too must our methods for identifying and countering their misuse.
Continuing to develop advanced AI detection systems while fostering cross-sector cooperation will be key to maintaining the integrity and trust in digital media.
By embracing a comprehensive approach that combines technology, collaboration, and legislation, we can effectively mitigate the risks and harness the potential of AI technologies for beneficial purposes.
With careful oversight and proactive measures, the digital world can remain a space of innovation and trust amidst the challenges posed by deepfake and adversarial images.
資料ダウンロード
QCD調達購買管理クラウド「newji」は、調達購買部門で必要なQCD管理全てを備えた、現場特化型兼クラウド型の今世紀最高の購買管理システムとなります。
ユーザー登録
調達購買業務の効率化だけでなく、システムを導入することで、コスト削減や製品・資材のステータス可視化のほか、属人化していた購買情報の共有化による内部不正防止や統制にも役立ちます。
NEWJI DX
製造業に特化したデジタルトランスフォーメーション(DX)の実現を目指す請負開発型のコンサルティングサービスです。AI、iPaaS、および先端の技術を駆使して、製造プロセスの効率化、業務効率化、チームワーク強化、コスト削減、品質向上を実現します。このサービスは、製造業の課題を深く理解し、それに対する最適なデジタルソリューションを提供することで、企業が持続的な成長とイノベーションを達成できるようサポートします。
オンライン講座
製造業、主に購買・調達部門にお勤めの方々に向けた情報を配信しております。
新任の方やベテランの方、管理職を対象とした幅広いコンテンツをご用意しております。
お問い合わせ
コストダウンが利益に直結する術だと理解していても、なかなか前に進めることができない状況。そんな時は、newjiのコストダウン自動化機能で大きく利益貢献しよう!
(Β版非公開)