- お役立ち記事
- Key points for introducing and implementing “AI-based abnormality detection technology” at manufacturing sites
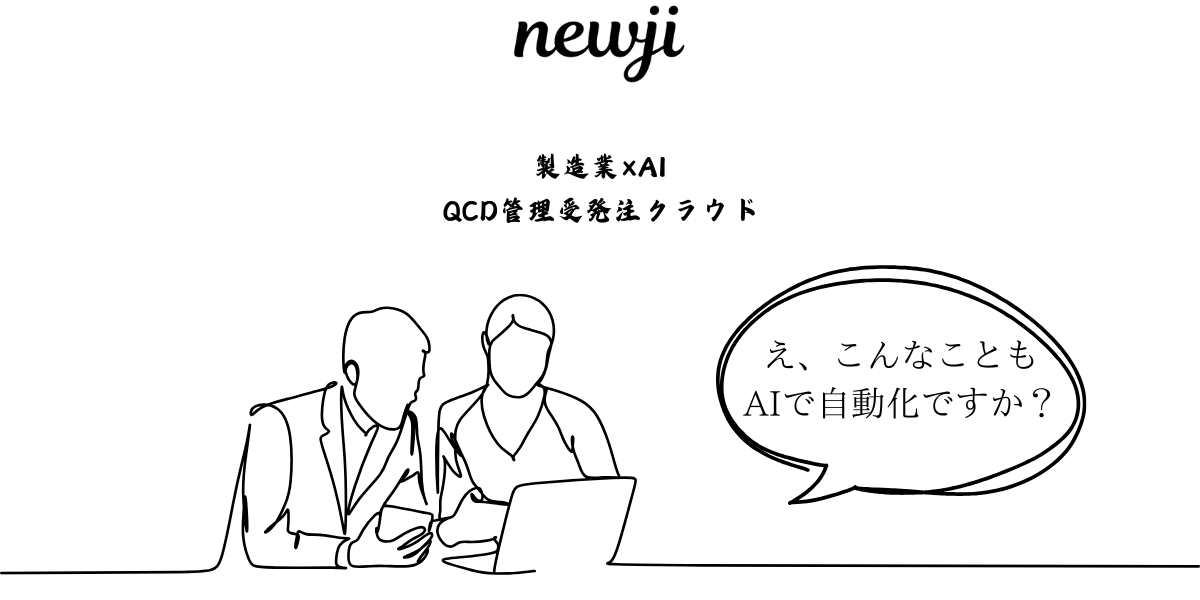
Key points for introducing and implementing “AI-based abnormality detection technology” at manufacturing sites
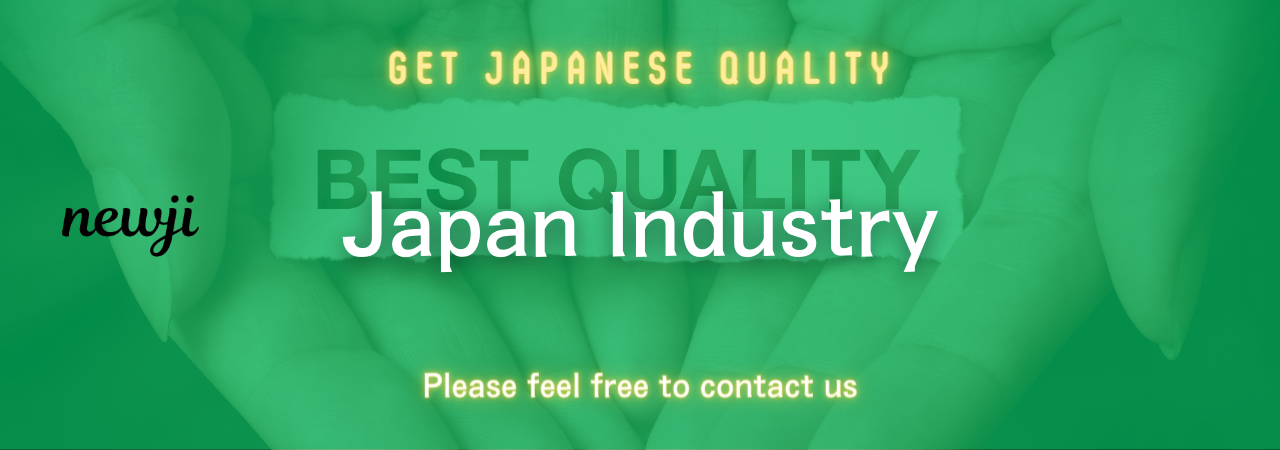
目次
Understanding AI-Based Abnormality Detection in Manufacturing
AI-based abnormality detection technology is revolutionizing manufacturing processes by enhancing efficiency and reducing unexpected downtimes.
This advanced technology uses artificial intelligence to monitor, analyze, and detect anomalies in machinery and production lines, ensuring optimal performance and safety.
Implementing AI-based systems can seem complex, but breaking down the key points can help manufacturing businesses adapt seamlessly to these cutting-edge solutions.
The Importance of Abnormality Detection
In a manufacturing environment, abnormalities can manifest as mechanical failures, deviations in production quality, or unpredictable equipment behavior.
These anomalies can lead to costly repairs, product defects, and even safety hazards if not promptly addressed.
Conventional monitoring systems and manual checks often fall short in handling the vast amounts of data generated in real-time, requiring an automated, intelligent approach.
AI-based abnormality detection leverages machine learning algorithms and data analytics to identify and predict potential issues before they escalate.
This technology is invaluable for maintaining production flow, enhancing product quality, and ensuring workplace safety.
Choosing the Right AI Technology
Selecting the appropriate AI technology for abnormality detection involves understanding the specific needs and constraints of your manufacturing environment.
Firstly, it’s crucial to identify the areas where anomalies are most likely to occur and the factors contributing to their development.
Consider the following points:
– **Type of Equipment**: Is it older, requiring more frequent monitoring, or newer with more sophisticated built-in diagnostics?
– **Data Availability**: Determine the accessibility and quality of historical and real-time data streams to train AI algorithms effectively.
– **Integration Capability**: Assess the ease of integrating AI systems with existing technology infrastructure to ensure seamless operation.
– **Scalability**: Ensure that the chosen AI solution can scale with production demands and adapt to future technological advancements.
Implementation Steps for AI-Based Abnormality Detection
Implementing AI-based abnormality detection involves several crucial steps, from preparation to deployment and ongoing maintenance.
Data Collection and Preparation
Data is the cornerstone of any AI solution.
Begin by gathering comprehensive historical data along with real-time data from sensors and other monitoring devices.
Data must be cleansed to remove noise and irrelevant information that could impede accurate model training.
Model Selection and Training
Choose machine learning models that best suit your anomaly detection needs, such as supervised learning models using labeled datasets or unsupervised models that can detect anomalies autonomously.
Training these models involves feeding them large volumes of pre-processed data to recognize patterns that indicate normal and abnormal conditions.
The more accurate your training data and model selection, the better the system’s ability to detect anomalies.
Testing and Validation
Testing is a critical phase where you evaluate the model’s performance in identifying anomalies under different scenarios and conditions.
It’s important to validate the accuracy of anomaly detection and minimize false positives and negatives to prevent unnecessary alerts or missed detections.
Integration and Deployment
Once validated, integrate the AI-based abnormality detection system into your production environment.
This integration involves connecting it with your existing monitoring systems, such as SCADA or IoT platforms, to ensure holistic oversight.
During deployment, configure alerting mechanisms to notify relevant personnel of detected anomalies promptly.
This might include immediate communication through dashboards, alarms, or other notification systems.
Monitoring and Maintenance
After deployment, continuous monitoring is essential to ensure the system adapts to changes in the manufacturing process and maintains its effectiveness.
Regularly update and re-train the models with new data to address evolving production conditions and emerging anomalies.
Overcoming Challenges in Implementation
While AI-based abnormality detection offers numerous benefits, its implementation can come with challenges.
Data Quality and Quantity
Lack of sufficient quality data can limit the AI model’s accuracy.
Ensure your data collection processes are robust and capable of capturing precise and comprehensive datasets.
Investment and Cost-Effectiveness
Initial implementation costs can be substantial, but consider the long-term savings achieved through reduced downtime and improved productivity.
Evaluate the return on investment (ROI) by calculating the cost savings from enhanced efficiency and reduced failure rates.
Change Management
Introduce AI solutions sensitively to ensure acceptance from staff who might be wary of automation or AI.
Provide education and training to help teams understand the benefits and workings of the technology, enhancing user trust and competency.
The Future of AI in Manufacturing
AI-based abnormality detection is just the beginning.
As technology evolves, AI’s role in manufacturing will expand, offering more comprehensive solutions across the production lifecycle.
Future AI systems will likely integrate deeper with predictive maintenance and advanced analytics, providing insights beyond anomaly detection.
Manufacturers who embrace AI will not only safeguard their operations but also gain a competitive edge through heightened innovation and efficiency.
By carefully selecting, implementing, and maintaining AI-based abnormality detection technology, manufacturing facilities can significantly reduce risks, improve outcomes, and advance their operational capabilities.
資料ダウンロード
QCD調達購買管理クラウド「newji」は、調達購買部門で必要なQCD管理全てを備えた、現場特化型兼クラウド型の今世紀最高の購買管理システムとなります。
ユーザー登録
調達購買業務の効率化だけでなく、システムを導入することで、コスト削減や製品・資材のステータス可視化のほか、属人化していた購買情報の共有化による内部不正防止や統制にも役立ちます。
NEWJI DX
製造業に特化したデジタルトランスフォーメーション(DX)の実現を目指す請負開発型のコンサルティングサービスです。AI、iPaaS、および先端の技術を駆使して、製造プロセスの効率化、業務効率化、チームワーク強化、コスト削減、品質向上を実現します。このサービスは、製造業の課題を深く理解し、それに対する最適なデジタルソリューションを提供することで、企業が持続的な成長とイノベーションを達成できるようサポートします。
オンライン講座
製造業、主に購買・調達部門にお勤めの方々に向けた情報を配信しております。
新任の方やベテランの方、管理職を対象とした幅広いコンテンツをご用意しております。
お問い合わせ
コストダウンが利益に直結する術だと理解していても、なかなか前に進めることができない状況。そんな時は、newjiのコストダウン自動化機能で大きく利益貢献しよう!
(Β版非公開)