- お役立ち記事
- Key points of abnormal noise recognition technology and noise suppression/separation technology and their application to abnormality detection
月間76,176名の
製造業ご担当者様が閲覧しています*
*2025年3月31日現在のGoogle Analyticsのデータより
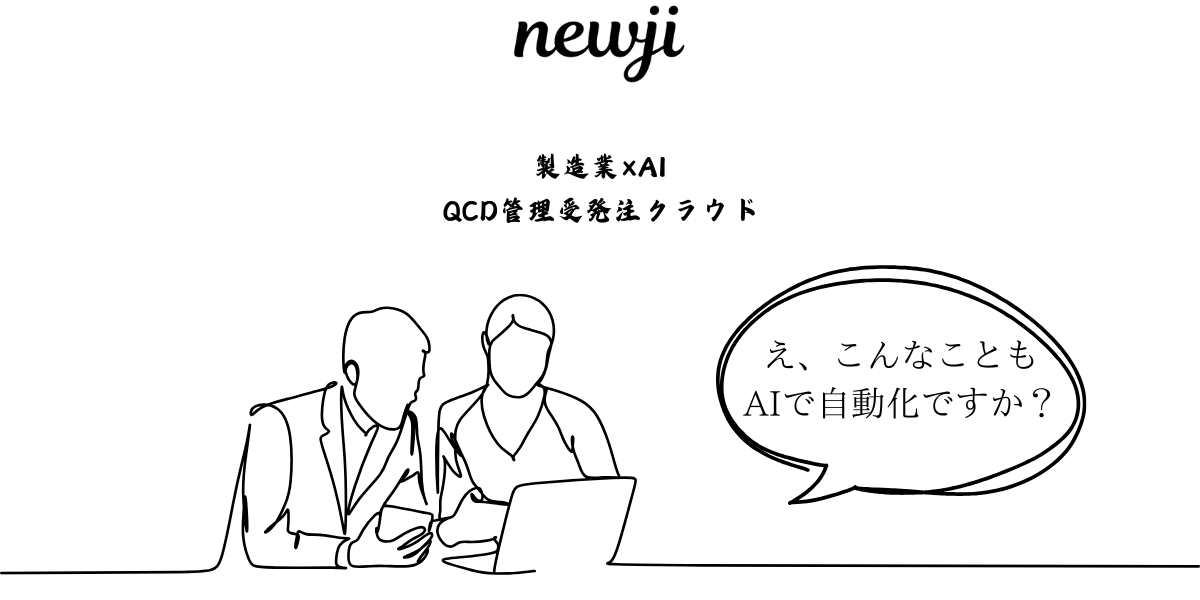
Key points of abnormal noise recognition technology and noise suppression/separation technology and their application to abnormality detection
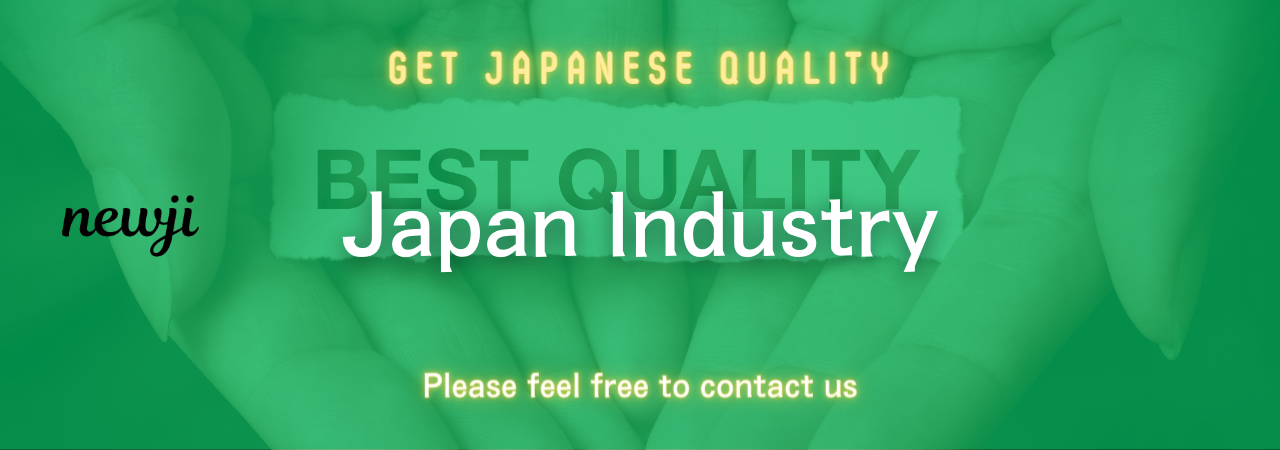
目次
Understanding Abnormal Noise Recognition Technology
Abnormal noise recognition technology is a sophisticated field that bridges the gap between sound analysis and machine learning.
Its primary role is in identifying irregular sounds that deviate from the norm in various environments.
These variations can indicate potential issues or malfunctions, hence the importance of accurately recognizing such noises.
Imagine a factory setting where machines operate continuously.
The consistent hum and periodic clicks represent normal operations.
However, an unexpected clatter or grinding noise might suggest a component fault.
Abnormal noise recognition technology goes beyond human auditory limitations by using algorithms trained to detect these subtle yet critical deviations.
The Role of Machine Learning
Machine learning plays a pivotal role in noise recognition.
By processing vast amounts of sound data, these systems learn to differentiate between normal and abnormal sounds without human intervention.
Algorithms such as deep learning and neural networks are often employed to improve recognition capabilities.
These algorithms first require a well-structured dataset featuring both normal and abnormal sound samples.
During the training phase, the system analyzes these samples to develop sound models.
When new data is introduced, the system compares it against these models, effectively highlighting anomalies.
Noise Suppression and Separation Technology
Noise suppression and separation technologies are integral to auditory data analysis.
These technologies help in isolating abnormal sounds by removing background noise, thus improving recognition accuracy.
Noise suppression focuses on reducing or eliminating unwanted sounds that may overshadow more significant auditory signals.
Advanced techniques, such as spectral subtraction and Wiener filtering, are often used to accomplish this.
These methods work by analyzing the frequency components of sound, identifying noise patterns, and minimizing their impact.
On the other hand, noise separation is about distinguishing different sound sources within an audio mix.
It allows professionals to isolate specific sounds, making it easier to identify and analyze them.
Independent Component Analysis (ICA) and Blind Source Separation (BSS) are popular methods that aid in unwinding these sound mixtures.
Application of These Technologies
Both noise suppression and separation technologies have far-reaching applications across various industries.
In automotive manufacturing, they ensure that car engines and other systems operate smoothly by flagging any abnormal noises.
Similarly, in aerospace, these technologies help detect irregular signifiers in machinery, ensuring safety and performance.
In the healthcare industry, hospitals use sound analysis to monitor the functionality of medical devices.
Through early detection of unusual noises, maintenance or replacement of faulty equipment can be carried out before a potential failure.
The consumer electronics sector also benefits, particularly in voice-activated technologies where distinguishing between human and background noise is crucial.
These technologies ensure accurate voice command recognition, thus enhancing user experience.
Implementing Abnormality Detection
Abnormality detection using noise analysis can be implemented in several steps.
First, gathering and preparing a comprehensive dataset of sound samples is essential.
This includes collecting both normal and abnormal noise data from the environment considered.
Next, the selection of an appropriate machine learning model tailored for sound analysis is crucial.
With a multitude of models available, choosing one that balances complexity and recognition capabilities is vital.
Once these steps are completed, the model undergoes training where it learns from the dataset.
Subsequently, this trained model is deployed in real-time environments to monitor for abnormal noises, allowing for immediate response to detected irregularities.
Challenges and Future Prospects
Despite the advances, several challenges remain in abnormal noise recognition and suppression.
Diverse environments and the plethora of potential sounds create variability that can affect accuracy.
Additionally, there is a constant need to update and retrain models to adapt to new sounds and equipment over time.
However, the future outlook remains promising.
With continuous refinements in artificial intelligence and computational power, noise recognition and suppression technologies will only become more precise and efficient.
Moreover, as more industries recognize the cost-saving and safety benefits, widespread adoption is likely to drive further innovations.
In conclusion, the development and application of noise recognition, suppression, and separation technologies serve as a significant enabler for modern industries.
By ensuring operational efficiency and safety through early anomaly detection, these technologies embody a vital component of current and future engineering solutions.
資料ダウンロード
QCD管理受発注クラウド「newji」は、受発注部門で必要なQCD管理全てを備えた、現場特化型兼クラウド型の今世紀最高の受発注管理システムとなります。
ユーザー登録
受発注業務の効率化だけでなく、システムを導入することで、コスト削減や製品・資材のステータス可視化のほか、属人化していた受発注情報の共有化による内部不正防止や統制にも役立ちます。
NEWJI DX
製造業に特化したデジタルトランスフォーメーション(DX)の実現を目指す請負開発型のコンサルティングサービスです。AI、iPaaS、および先端の技術を駆使して、製造プロセスの効率化、業務効率化、チームワーク強化、コスト削減、品質向上を実現します。このサービスは、製造業の課題を深く理解し、それに対する最適なデジタルソリューションを提供することで、企業が持続的な成長とイノベーションを達成できるようサポートします。
製造業ニュース解説
製造業、主に購買・調達部門にお勤めの方々に向けた情報を配信しております。
新任の方やベテランの方、管理職を対象とした幅広いコンテンツをご用意しております。
お問い合わせ
コストダウンが利益に直結する術だと理解していても、なかなか前に進めることができない状況。そんな時は、newjiのコストダウン自動化機能で大きく利益貢献しよう!
(β版非公開)