- お役立ち記事
- Learn the basics of PID control through control experiments and key points for improving control performance through model-based design
月間76,176名の
製造業ご担当者様が閲覧しています*
*2025年3月31日現在のGoogle Analyticsのデータより
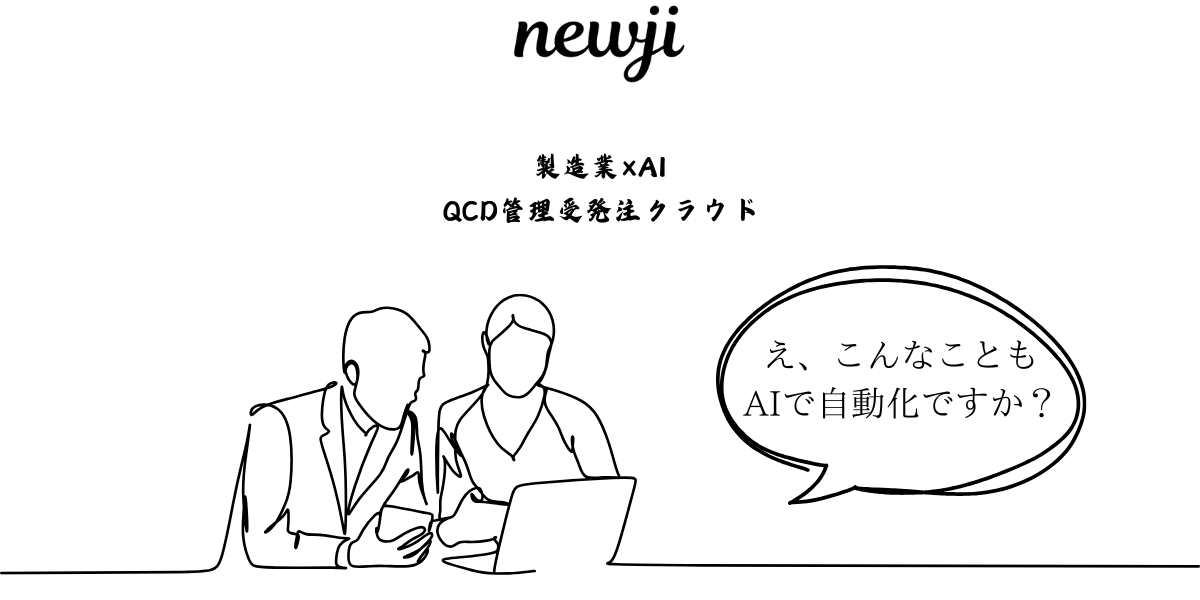
Learn the basics of PID control through control experiments and key points for improving control performance through model-based design
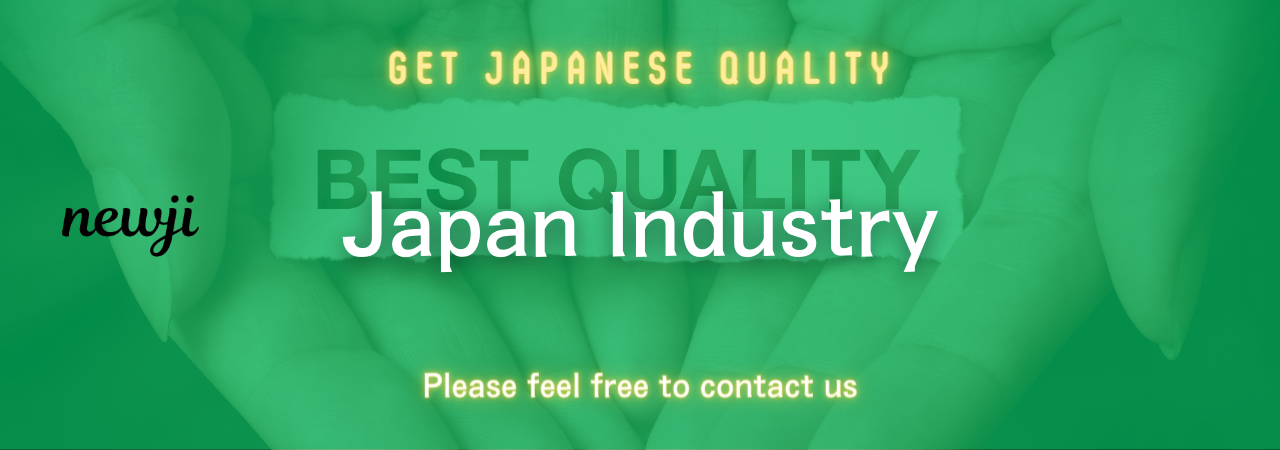
目次
Understanding PID Control
PID control, which stands for Proportional-Integral-Derivative control, is a widely used method in automation and control systems.
The fundamental objective of PID control is to maintain a desired set point by continuously adjusting the control input based on the feedback from the process being controlled.
This ensures stability and improves the performance of systems in various fields from industrial processes to everyday devices.
At its core, PID control operates using three primary components: the Proportional (P) term, the Integral (I) term, and the Derivative (D) term.
Each component plays a distinct role in achieving optimal control performance.
The Proportional term accounts for the present error, the Integral term sums up past errors to eliminate steady-state error, and the Derivative term anticipates future errors by considering the rate of change.
The Basics of Control Experiments
To better understand PID control, let’s delve into control experiments.
These experiments allow us to observe how changes to the PID parameters influence the behavior of the system.
Starting with a simple experiment helps in comprehending the practical aspects of PID control.
Imagine a scenario where you need to control temperature in an oven to maintain a consistent level.
By setting up a control loop, you can adjust the oven’s heat source so that the temperature remains close to the desired value.
Using a PID controller, you will experiment with different settings for P, I, and D terms, observing how the system responds.
Begin by adjusting the Proportional term while keeping the Integral and Derivative terms constant.
Notice how varying the Proportional gain (Kp) affects the system.
Higher values of Kp can lead to an aggressive response and can overshoot the target, while lower values may result in a sluggish response.
Next, introduce changes to the Integral term.
The Integral gain (Ki) helps eliminate any persistent offsets that the Proportional term couldn’t correct on its own.
A higher Integral gain can speed up the response but may also cause oscillations if too aggressive.
Finally, tweak the Derivative term.
The Derivative gain (Kd) predicts future errors and dampens oscillations by slowing down the rate of change.
However, excessive Derivative action can make the system too sensitive to noise.
Key Points for Improving Control Performance
Improving PID control performance requires a systematic approach and fine-tuning of the controller parameters.
However, even with optimal tuning, external factors and model specifications can significantly impact performance.
This is where model-based design becomes invaluable.
Utilizing a dynamic model of the system allows you to simulate various scenarios and refine the control strategy without affecting the actual process.
An accurate model provides insights into how the system behaves under different conditions, enabling improved PID parameter selection.
Steps to Enhance Control Through Model-Based Design
1. **Develop a System Model:**
Create a mathematical representation of the system you wish to control.
This could involve differential equations that describe the system dynamics or using software tools for complex systems.
2. **Perform System Identification:**
Gather data from the real system to adjust and validate your model.
Ensure the model accurately predicts the system’s behavior over various operating conditions.
3. **Simulate System Behavior:**
Use your model to simulate different PID settings.
Assess how changes to P, I, and D terms affect system stability, overshoot, settling time, and steady-state error.
4. **Optimize PID Parameters:**
Employ techniques such as Genetic Algorithms, Simulated Annealing, or Particle Swarm Optimization to determine the optimal PID parameters for desired performance.
5. **Implement and Test:**
Apply the optimized parameters to the actual system and conduct tests to verify performance improvements.
Fine-tune based on real-world feedback until the desired control performance is achieved.
Conclusion
Learning the basics of PID control through control experiments provides foundational knowledge that forms the basis of robust control systems.
It’s critical to understand the influence of each PID component and their combined effect on system behavior.
Furthermore, incorporating model-based design can significantly enhance control performance by allowing for detailed analysis and parameter optimization in a simulated environment.
By systematically experimenting and leveraging model-based approaches, you can enhance system reliability, efficiency, and adaptability, leading to improved outcomes in a wide range of practical applications.
資料ダウンロード
QCD管理受発注クラウド「newji」は、受発注部門で必要なQCD管理全てを備えた、現場特化型兼クラウド型の今世紀最高の受発注管理システムとなります。
ユーザー登録
受発注業務の効率化だけでなく、システムを導入することで、コスト削減や製品・資材のステータス可視化のほか、属人化していた受発注情報の共有化による内部不正防止や統制にも役立ちます。
NEWJI DX
製造業に特化したデジタルトランスフォーメーション(DX)の実現を目指す請負開発型のコンサルティングサービスです。AI、iPaaS、および先端の技術を駆使して、製造プロセスの効率化、業務効率化、チームワーク強化、コスト削減、品質向上を実現します。このサービスは、製造業の課題を深く理解し、それに対する最適なデジタルソリューションを提供することで、企業が持続的な成長とイノベーションを達成できるようサポートします。
製造業ニュース解説
製造業、主に購買・調達部門にお勤めの方々に向けた情報を配信しております。
新任の方やベテランの方、管理職を対象とした幅広いコンテンツをご用意しております。
お問い合わせ
コストダウンが利益に直結する術だと理解していても、なかなか前に進めることができない状況。そんな時は、newjiのコストダウン自動化機能で大きく利益貢献しよう!
(β版非公開)